| F1-scores in % | |
---|
Participant | A | C00 | C01 | C02 | C03 | C04 | C05 | C06 | C07 | C08 | C09 | C10 | mF1 | OA |
---|
ifp-RF210223 | ➥ | 90.36 | 88.55 | 66.89 | 51.55 | 96.06 | 78.47 | 67.25 | 95.91 | 47.91 | 59.73 | 80.65 | 74.85 | 87.43 |
ifp-SCN210223 | ➥ | 92.31 | 88.14 | 63.51 | 57.17 | 96.86 | 83.19 | 68.59 | 96.98 | 44.81 | 78.20 | 73.61 | 76.67 | 88.42 |
Gao-KPConv210422 | ➥ | 88.57 | 88.93 | 82.10 | 63.89 | 97.13 | 85.13 | 75.24 | 97.38 | 42.68 | 80.87 | 0.00 | 72.90 | 87.69 |
Gao-PN++210422 | ➥ | 78.11 | 72.07 | 31.78 | 13.65 | 73.98 | 47.79 | 28.34 | 71.80 | 9.65 | 21.67 | 4.39 | 41.20 | 68.50 |
Sevgen220117 | ➥ | 83.86 | 77.21 | 66.95 | 42.64 | 95.60 | 80.09 | 59.53 | 96.06 | 25.68 | 81.51 | 73.85 | 71.18 | 79.25 |
grilli220725 | ➥ | 88.91 | 87.74 | 74.89 | 54.27 | 96.83 | 78.85 | 53.21 | 93.28 | 43.73 | 75.89 | 58.22 | 73.26 | 85.33 |
Sevgen220725 | ➥ | 90.88 | 89.40 | 77.28 | 55.76 | 97.05 | 81.88 | 62.06 | 97.10 | 23.17 | 80.27 | 80.28 | 75.92 | 87.59 |
Shi220705 | ➥ | 87.62 | 85.62 | 52.40 | 36.71 | 95.48 | 69.30 | 47.39 | 94.28 | 25.08 | 65.94 | 38.59 | 63.49 | 84.20 |
Zhan221025 | ➥ | 84.33 | 77.86 | 58.11 | 42.32 | 93.25 | 65.41 | 53.53 | 95.29 | 23.66 | 59.85 | 64.66 | 65.30 | 79.69 |
Zhan221112 | ➥ | 58.74 | 22.06 | 0.32 | 16.65 | 57.06 | 30.02 | 5.07 | 69.34 | 0.11 | 28.59 | 2.22 | 26.38 | 46.10 |
jiabin221114 | ➥ | 66.21 | 18.02 | 34.18 | 38.03 | 72.00 | 68.99 | 47.70 | 78.65 | 9.84 | 35.93 | 8.32 | 43.44 | 58.29 |
WHU221118 | ➥ | 92.90 | 90.23 | 78.51 | 57.89 | 95.71 | 80.43 | 68.46 | 97.21 | 62.37 | 73.08 | 72.45 | 79.02 | 89.75 |
Esmoris230207 | ➥ | 84.83 | 81.95 | 19.45 | 42.38 | 94.83 | 77.77 | 59.06 | 94.53 | 3.55 | 73.80 | 69.18 | 63.76 | 81.67 |
Esmoris230208 | ➥ | 71.13 | 55.83 | 2.73 | 25.85 | 93.74 | 73.28 | 38.95 | 93.21 | 20.68 | 70.40 | 49.84 | 54.15 | 68.84 |
Letard230213 | ➥ | 68.48 | 65.40 | 32.95 | 42.06 | 93.61 | 80.39 | 43.72 | 94.17 | 14.33 | 72.84 | 42.45 | 59.13 | 66.70 |
WHU230322 | ➥ | 94.11 | 91.92 | 79.42 | 59.16 | 97.20 | 81.86 | 68.31 | 96.96 | 78.94 | 78.42 | 84.58 | 82.81 | 91.77 |
Qiu230831 | ➥ | 91.81 | 84.74 | 64.60 | 52.24 | 95.04 | 80.59 | 68.02 | 97.35 | 42.11 | 74.76 | 85.10 | 76.03 | 86.81 |
Zhang231204 | ➥ | 91.89 | 90.56 | 84.12 | 64.75 | 97.98 | 84.21 | 71.07 | 97.15 | 70.66 | 85.13 | 84.72 | 83.84 | 90.45 |
Results - Details for ifp-RF210223
Confusion Matrix
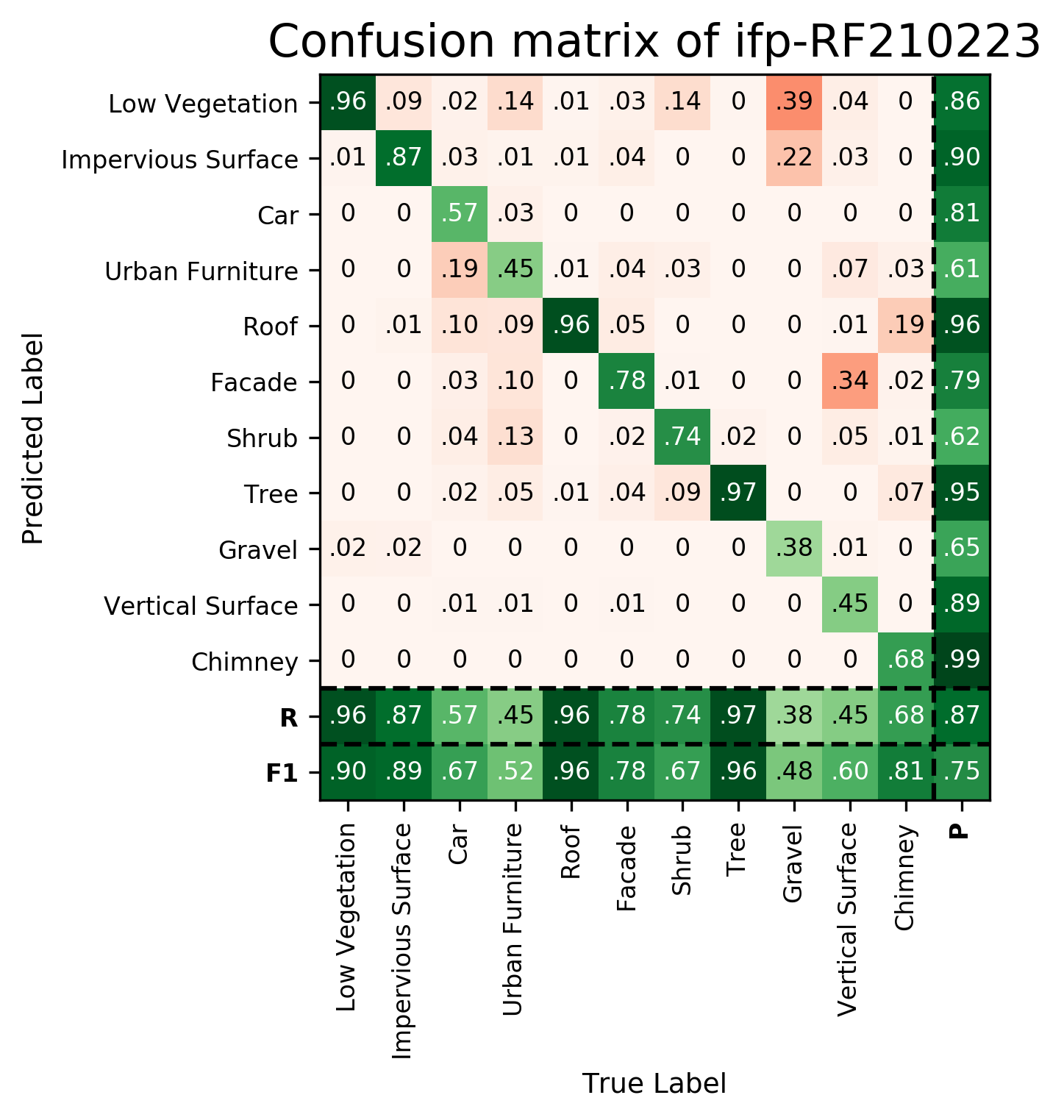
View 1
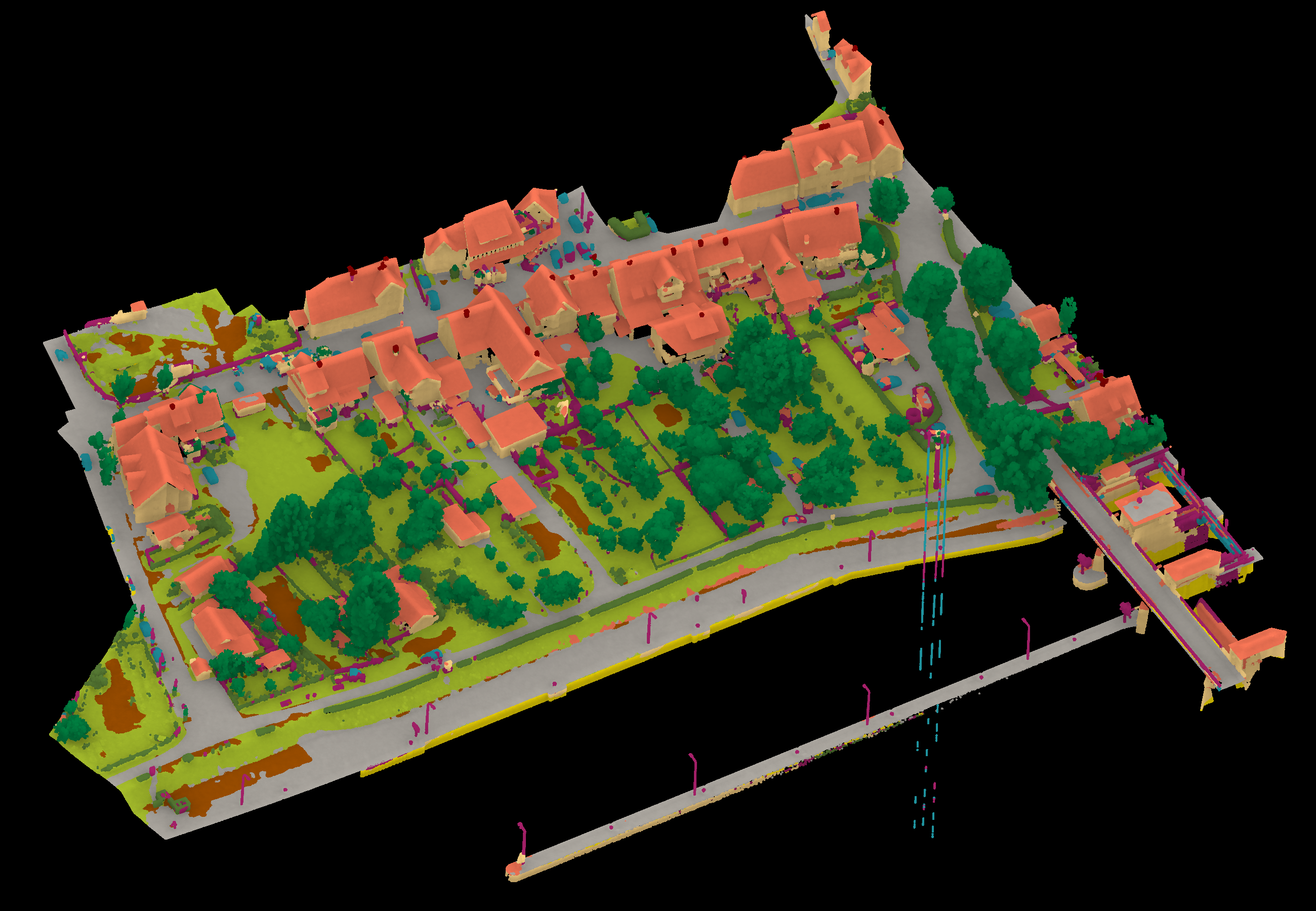
View 2
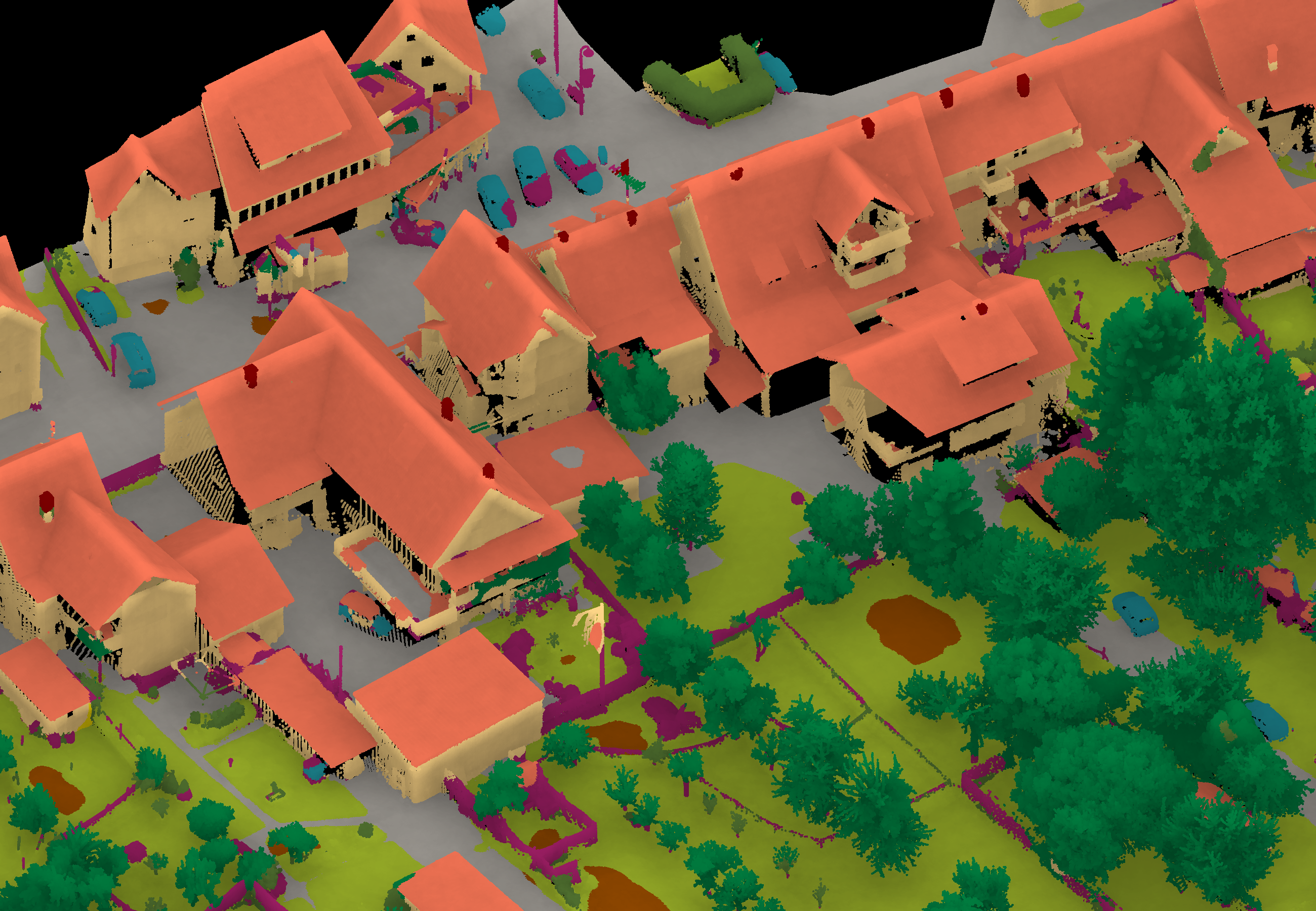
Results - Details for ifp-SCN210223
Confusion Matrix
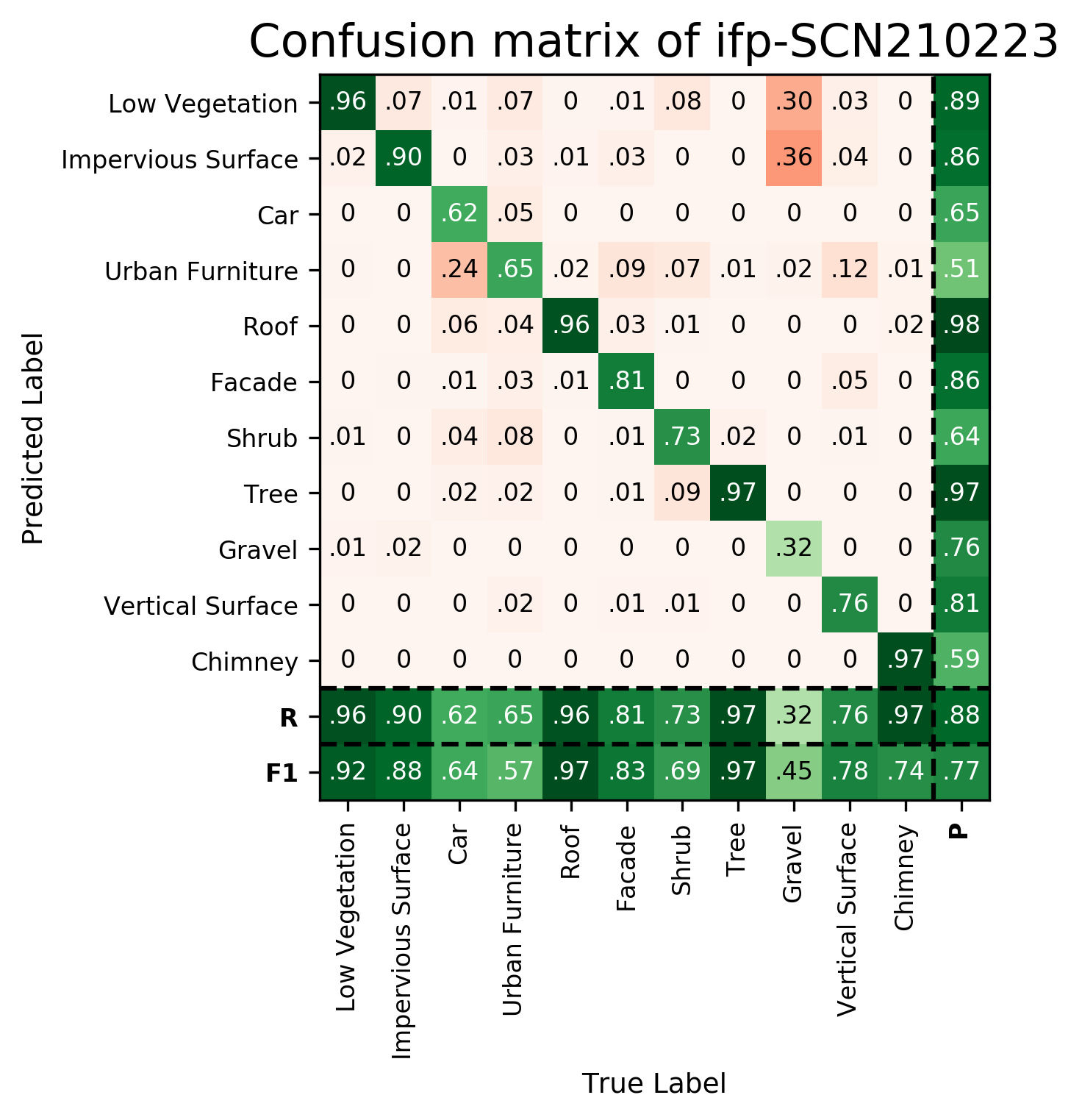
View 1
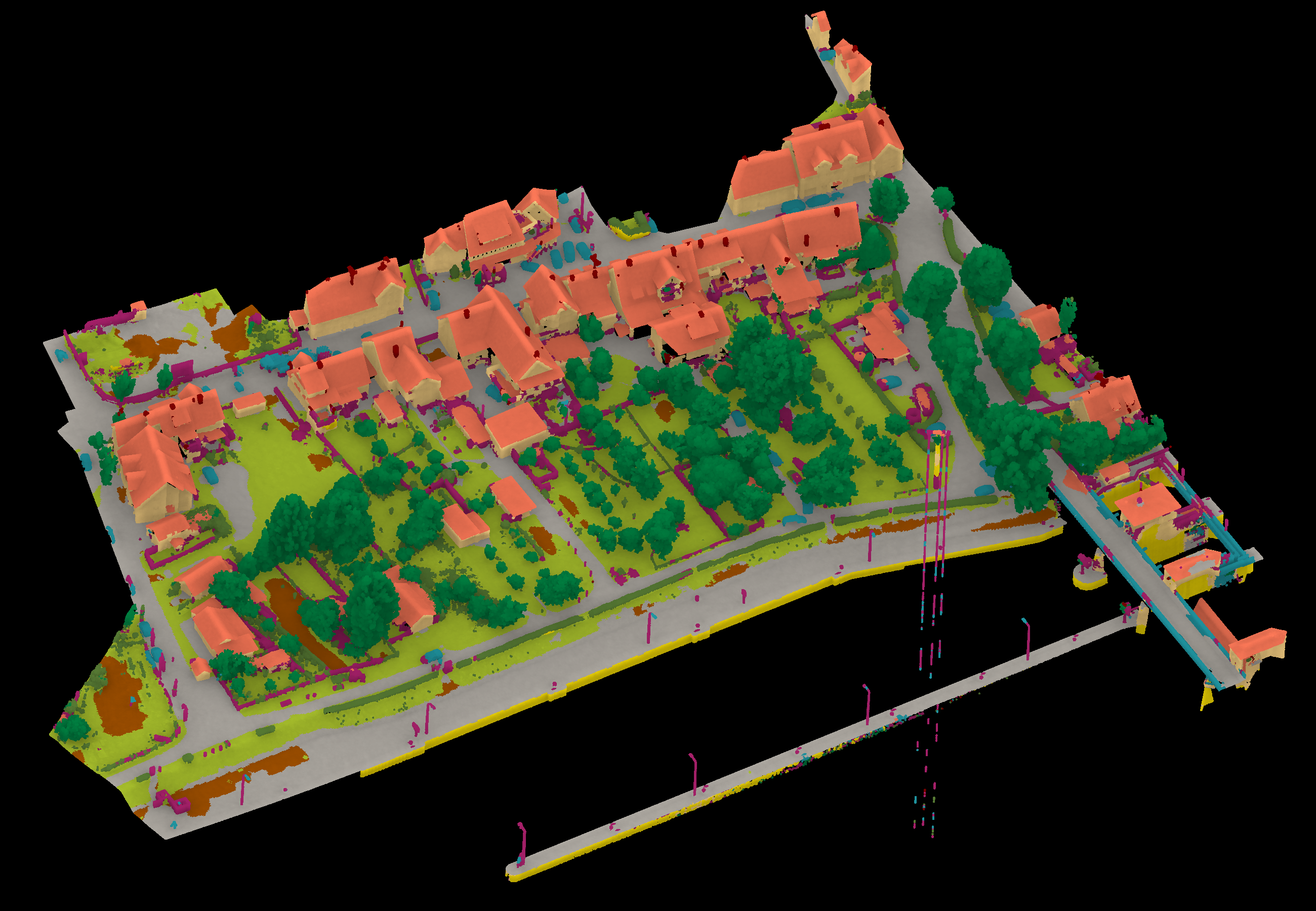
View 2
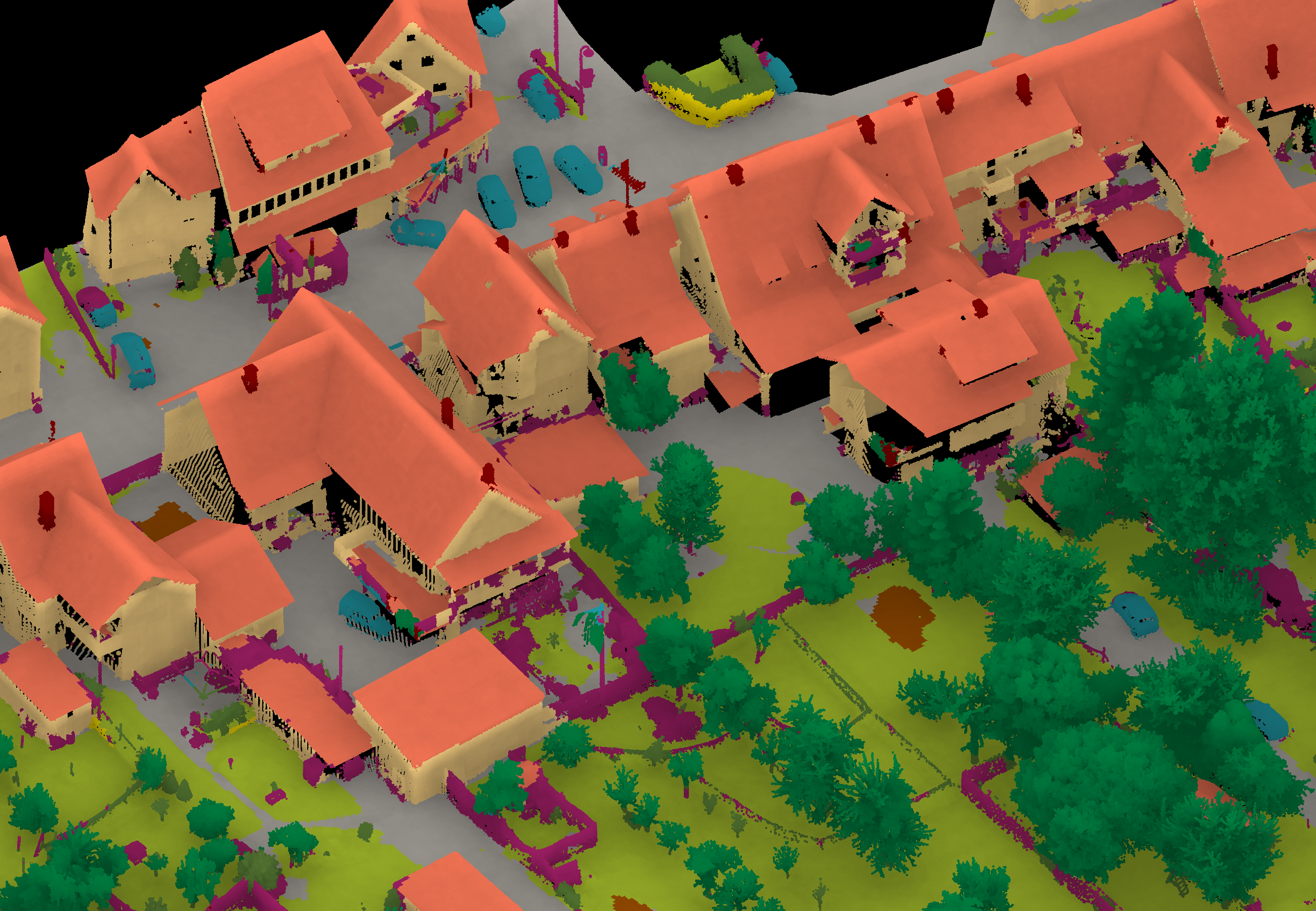
Results - Details for Gao-KPConv210422
Confusion Matrix
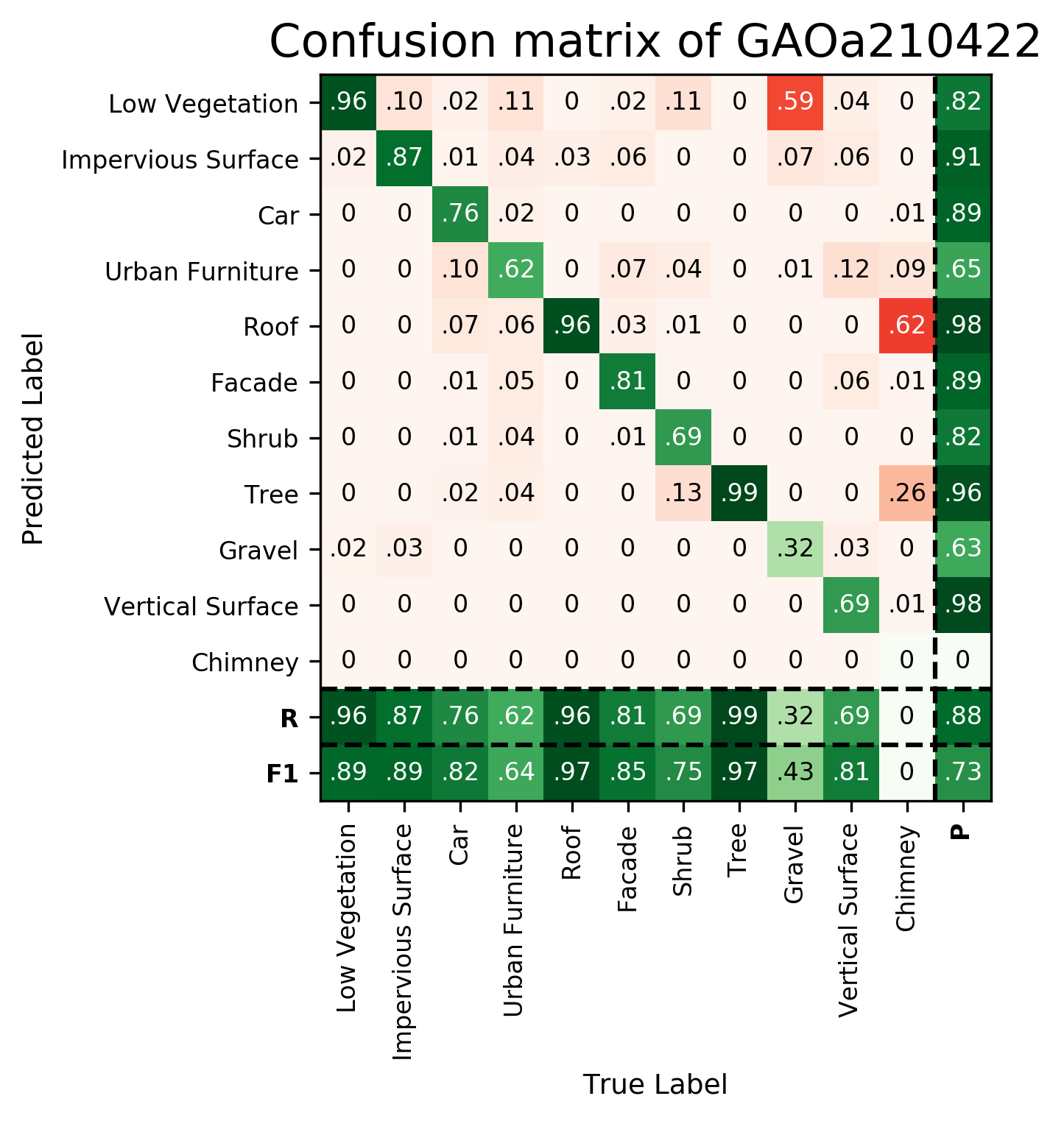
View 1
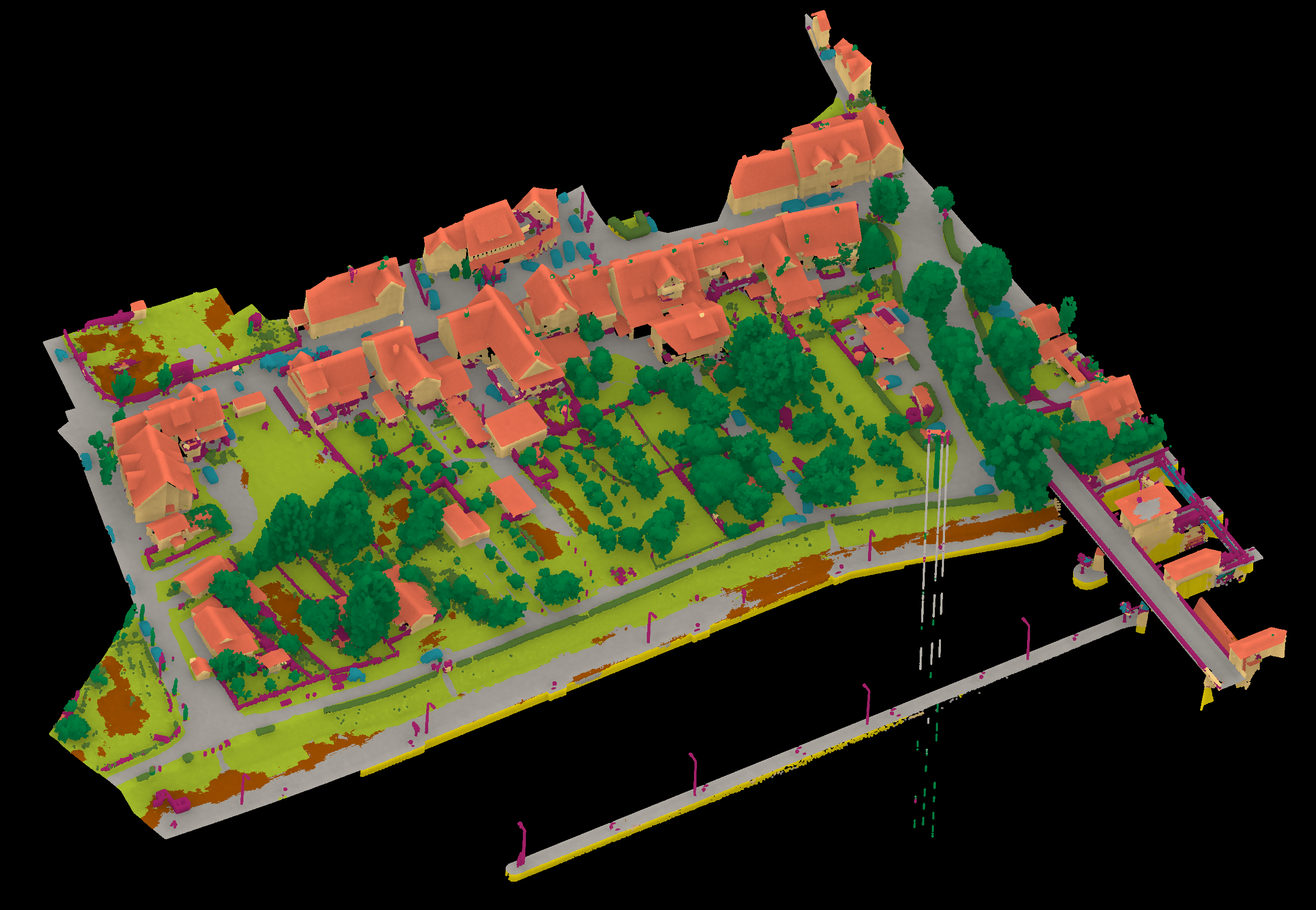
View 2
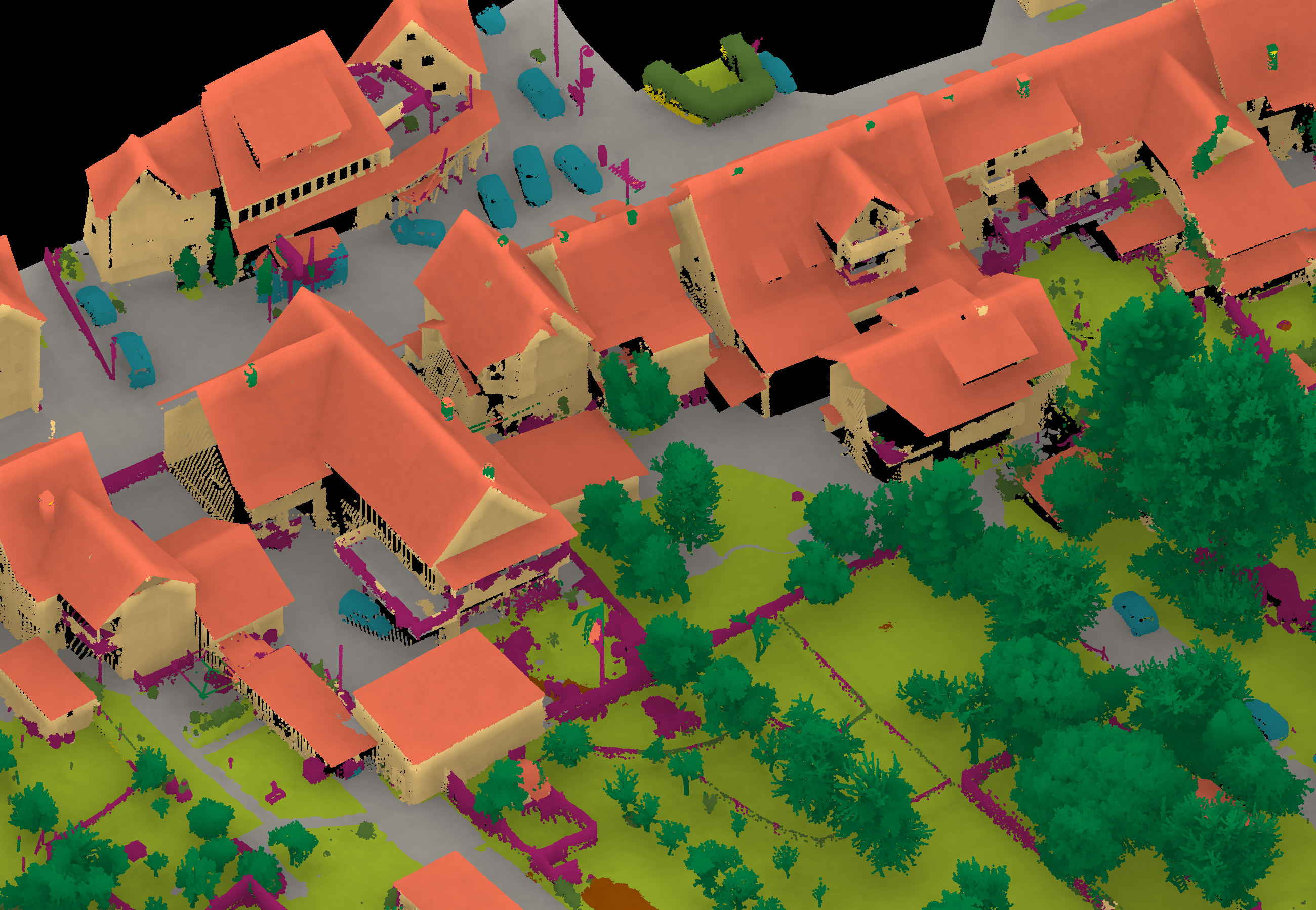
Results - Details for Gao-PN++210422
Confusion Matrix
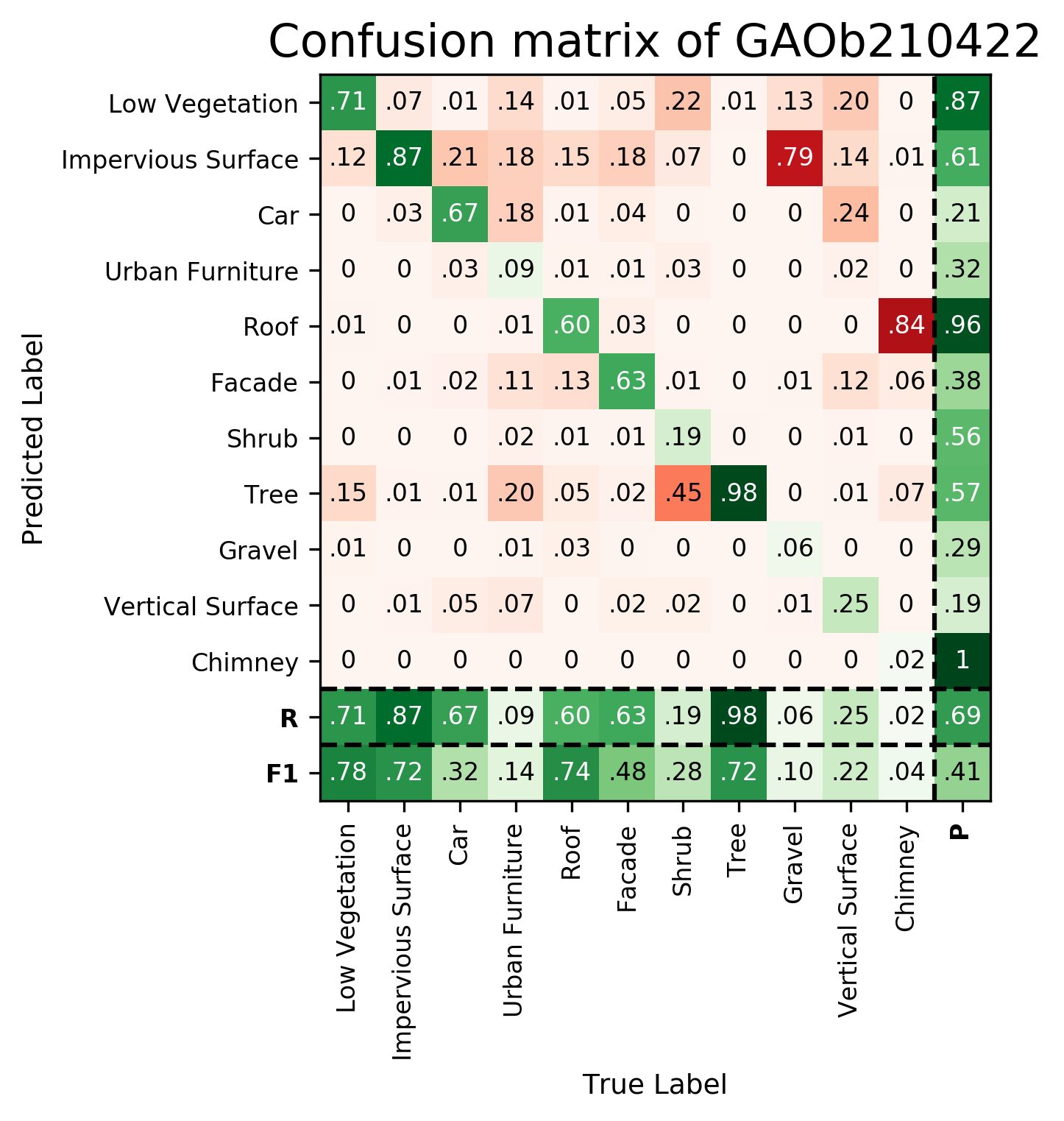
View 1
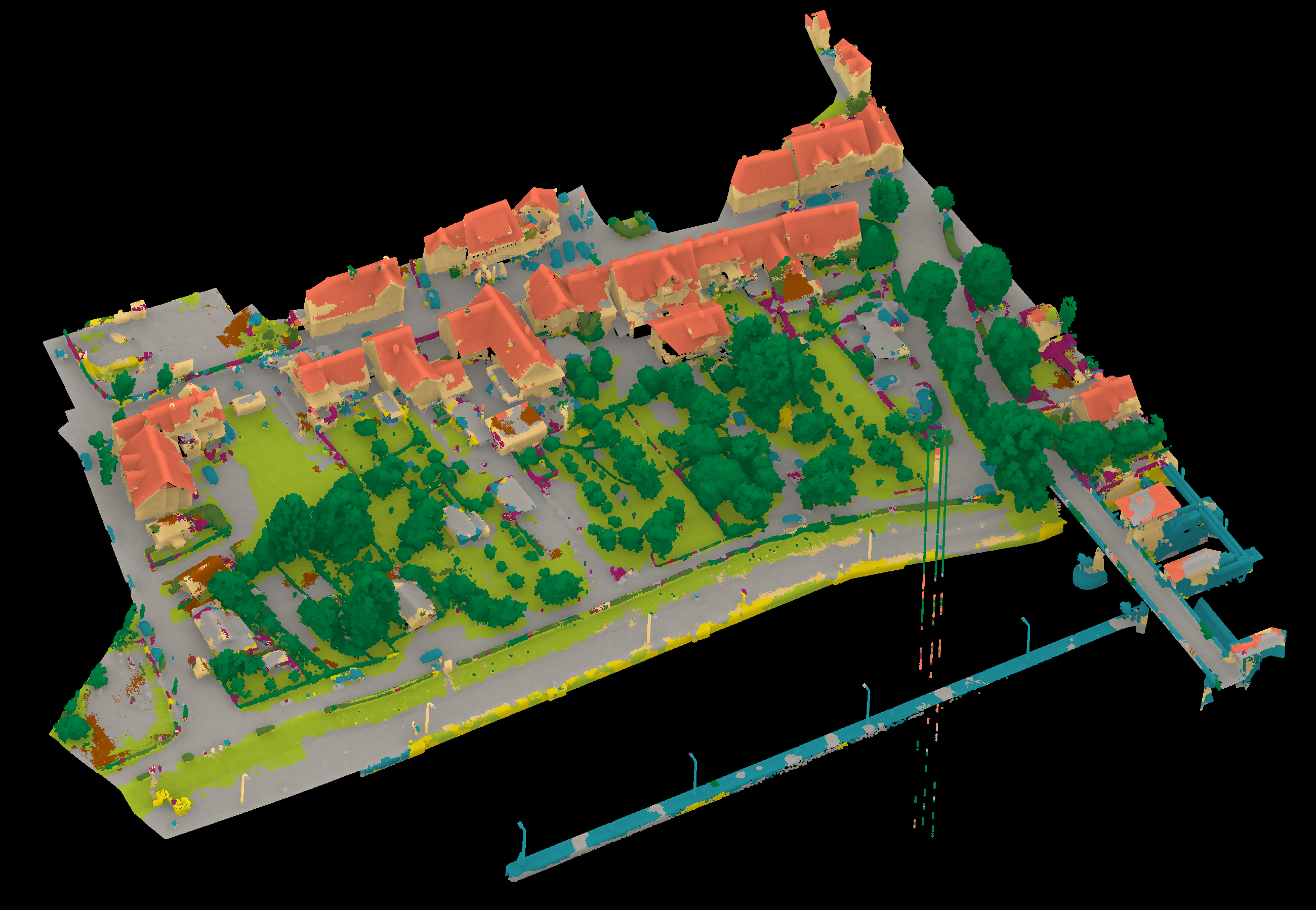
View 2
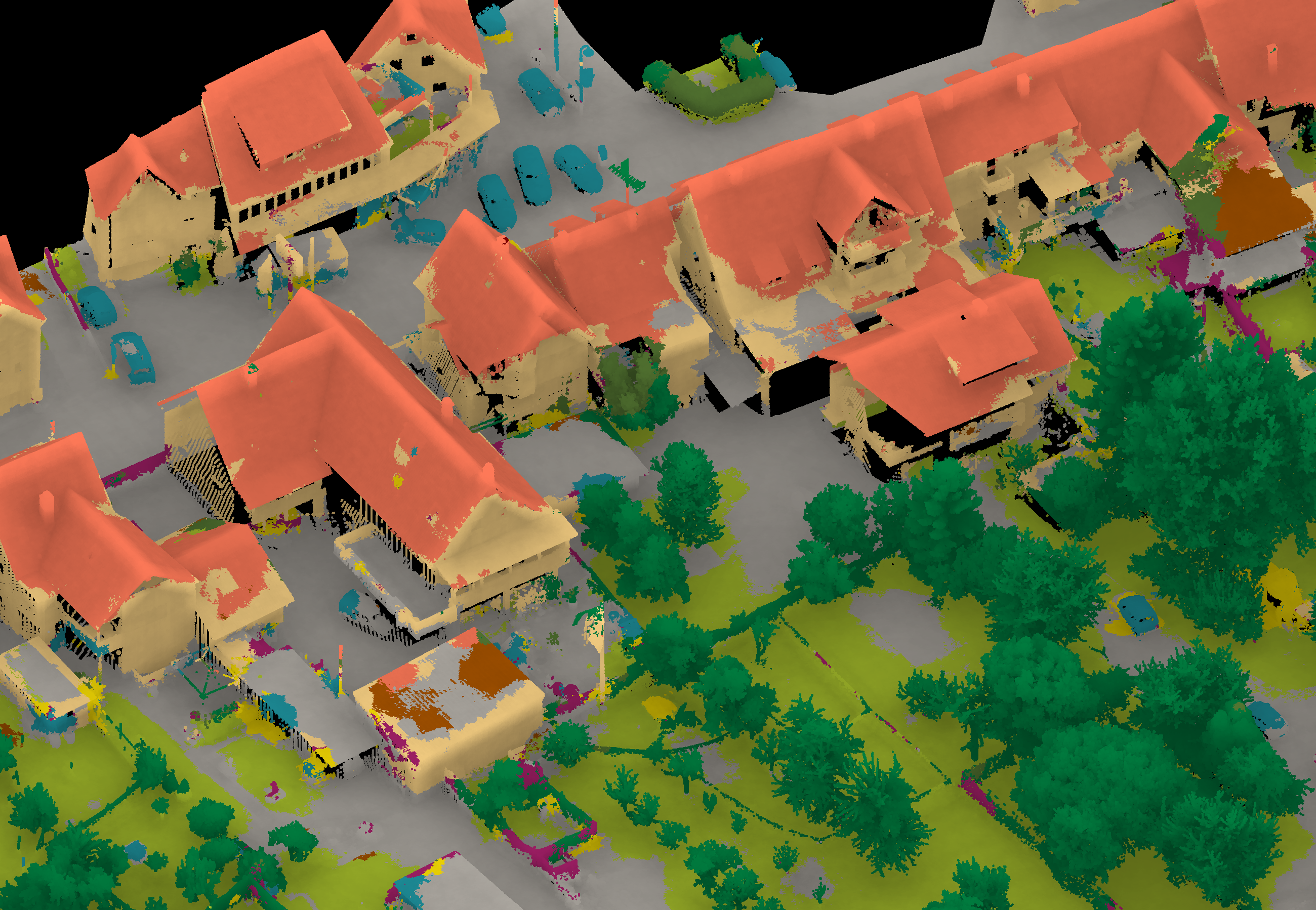
Results - Details for Sevgen220117
Confusion Matrix
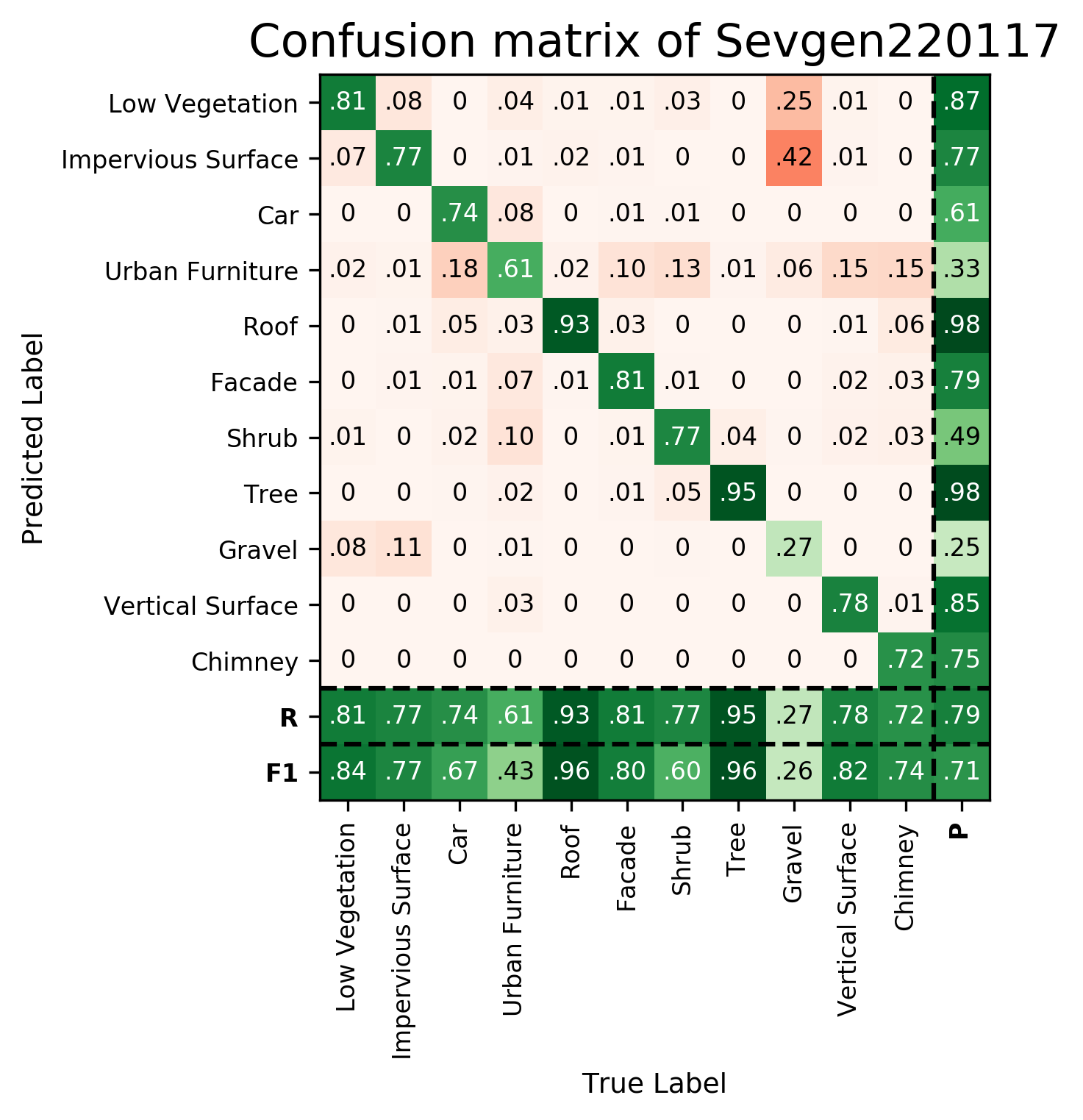
View 1
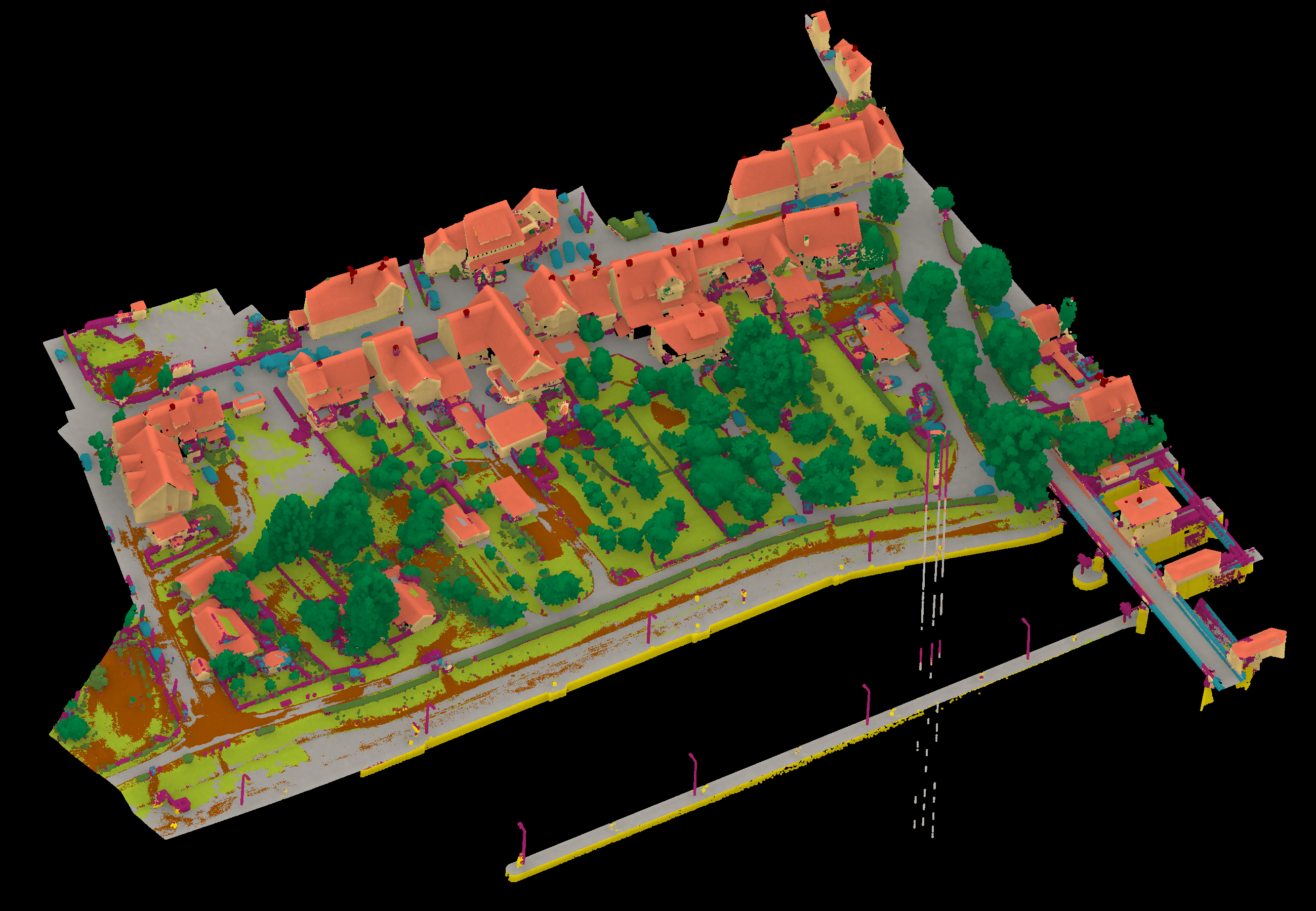
View 2
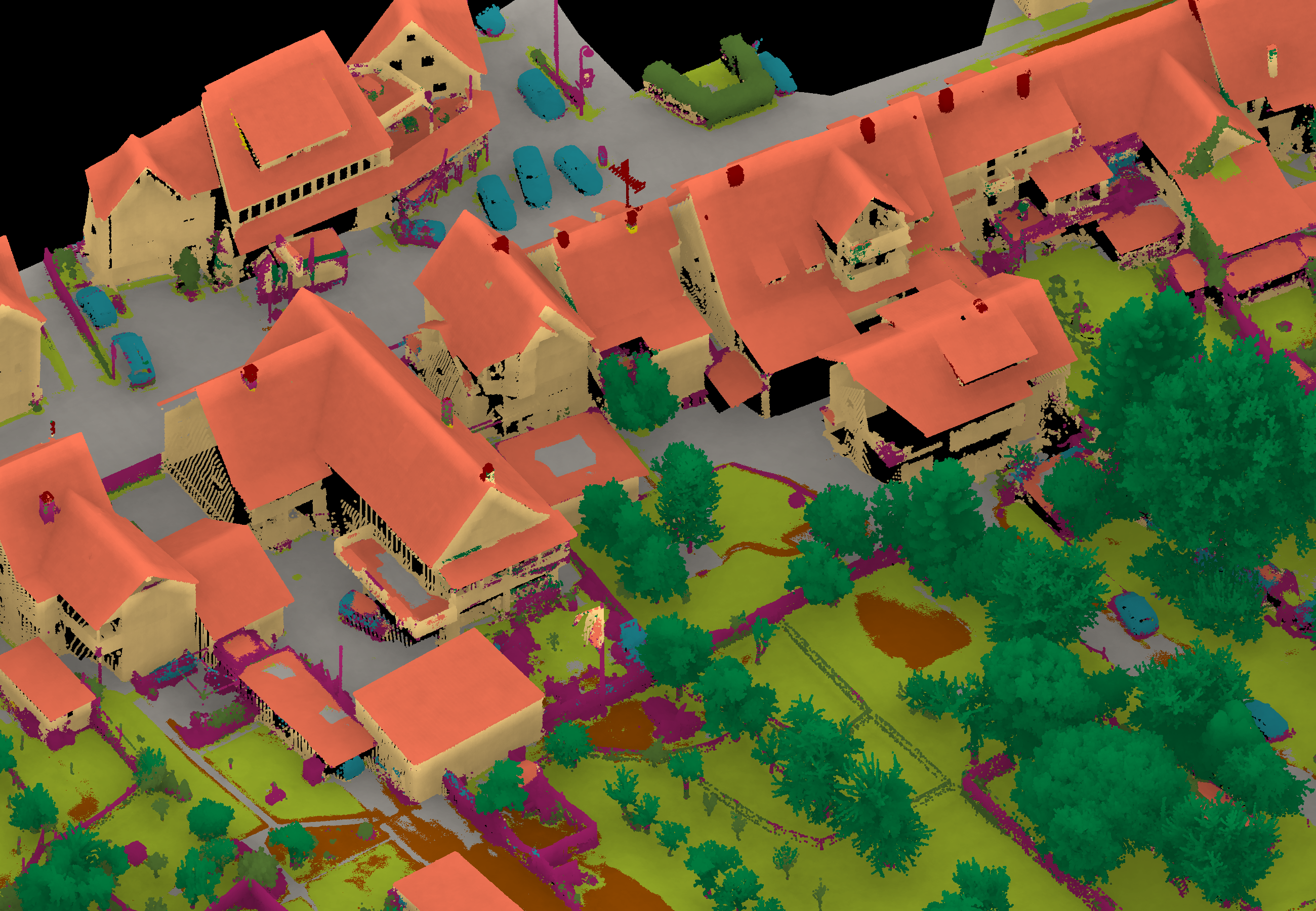
Results - Details for grilli220725
Confusion Matrix
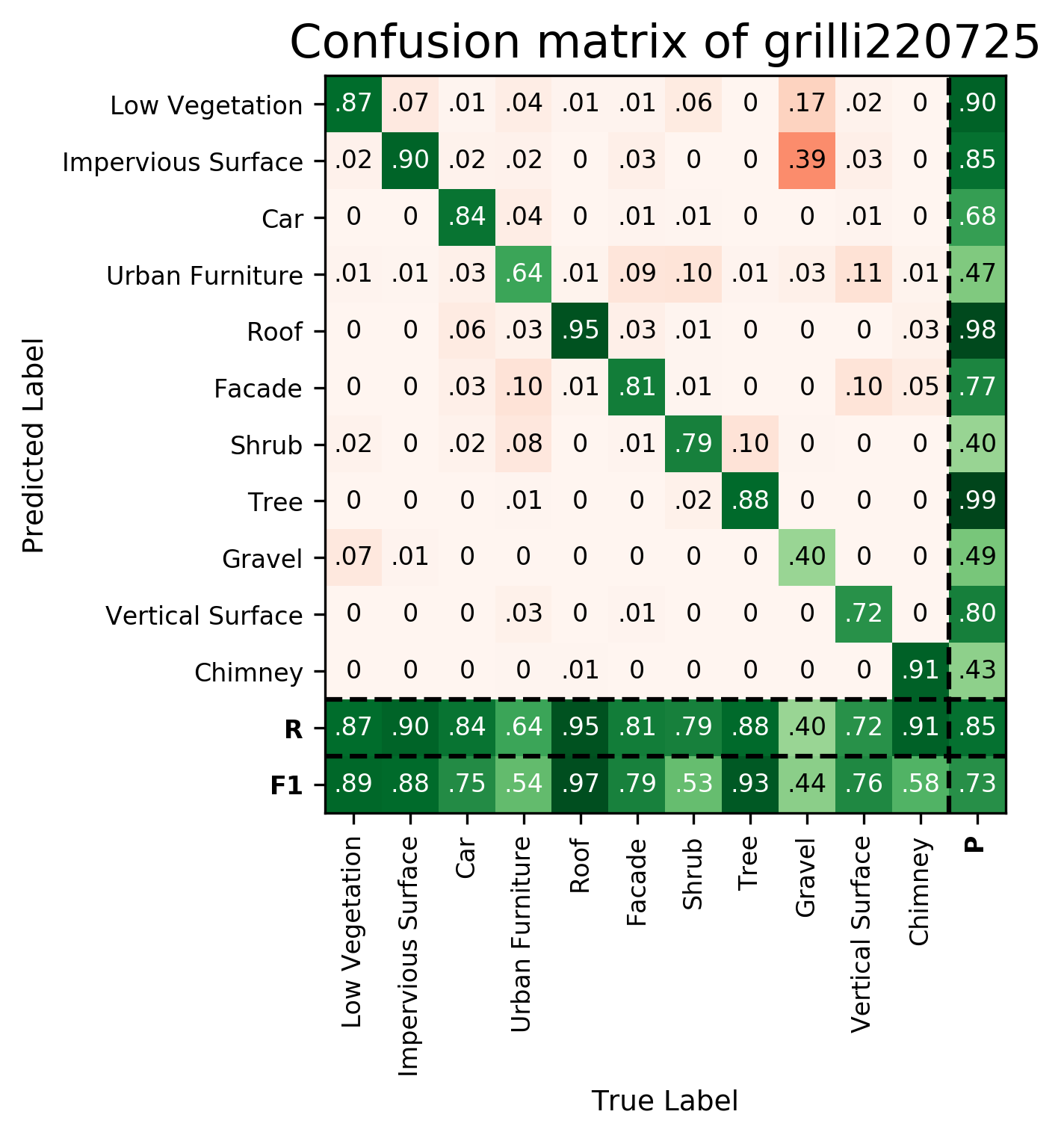
View 1
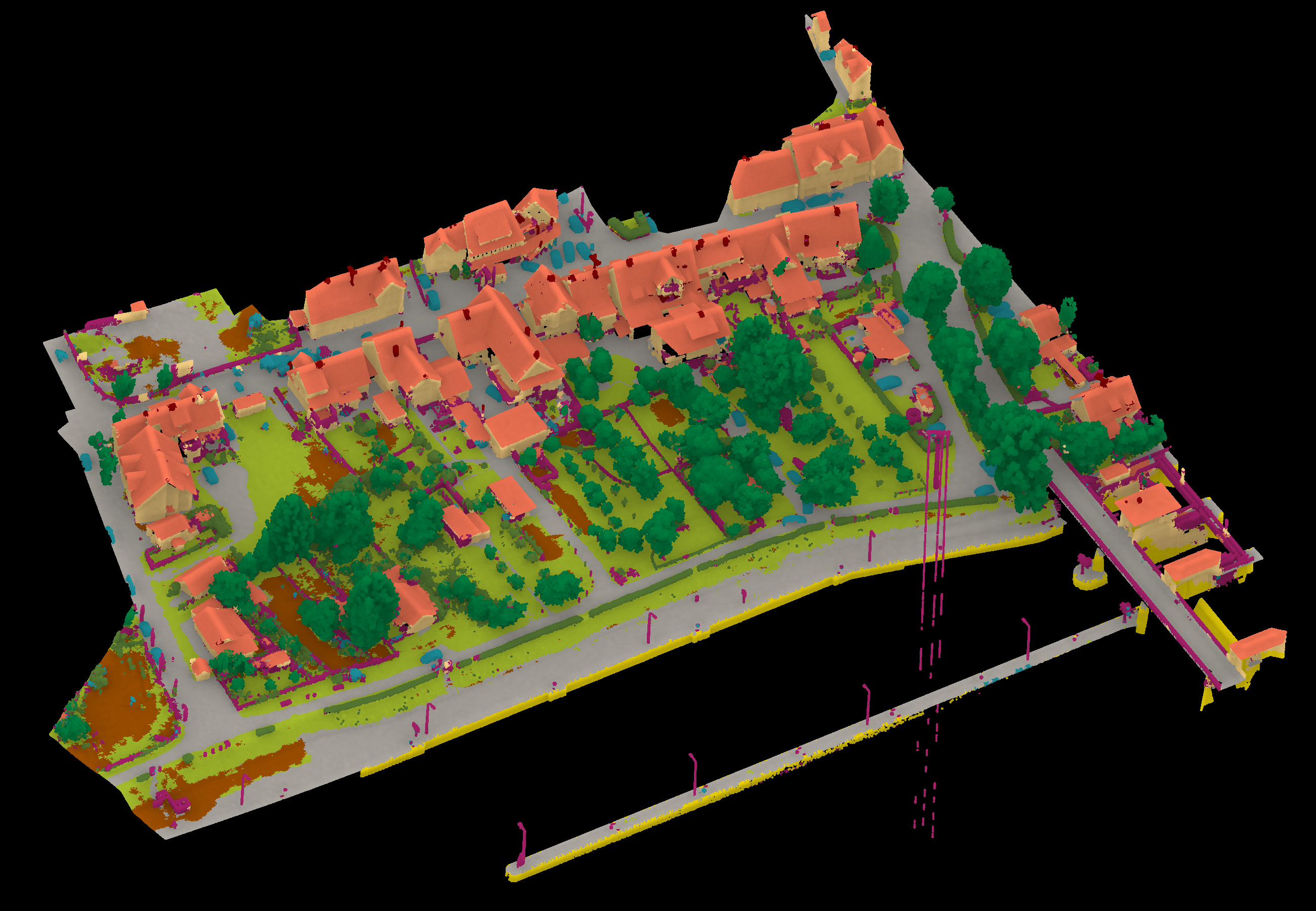
View 2
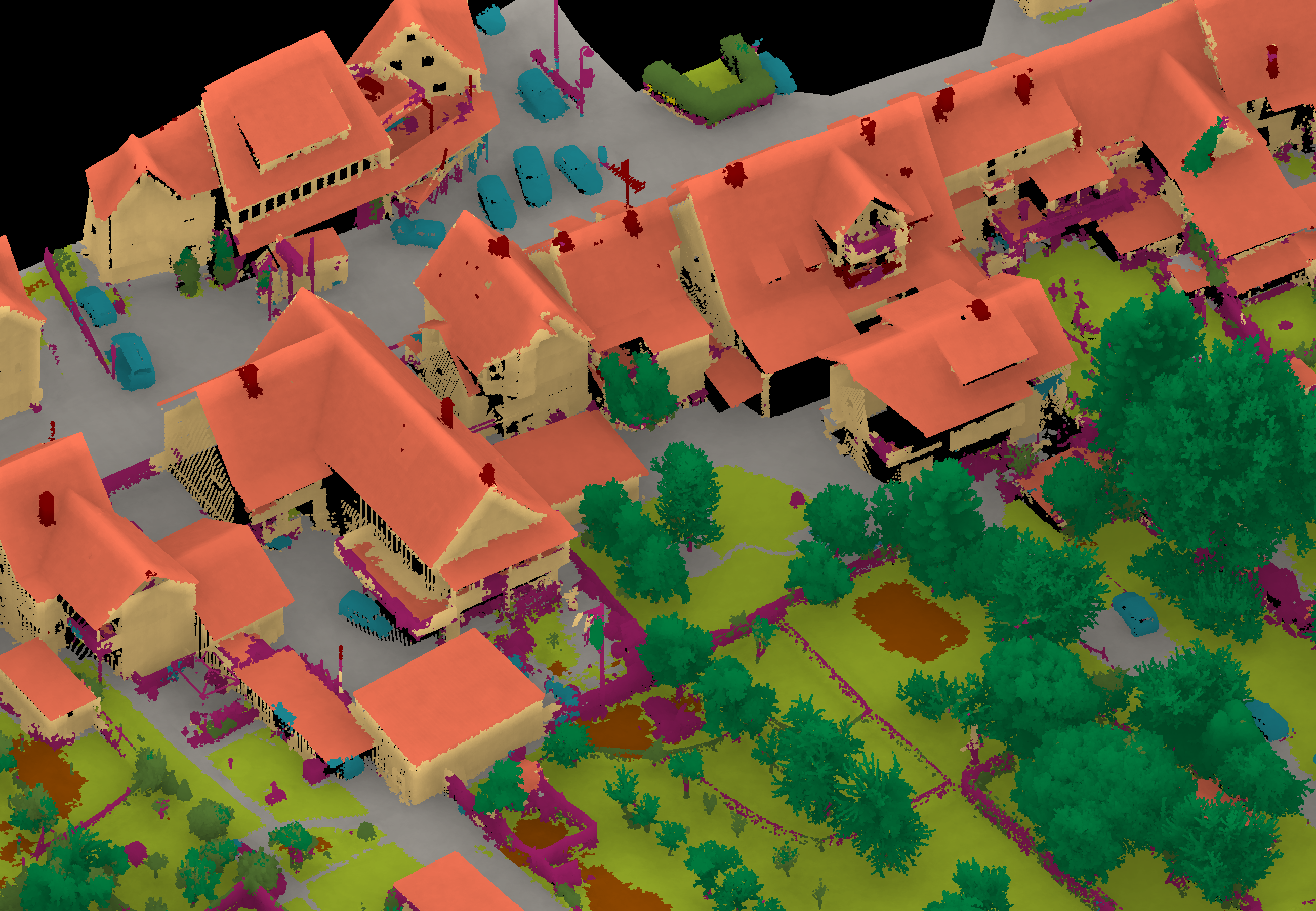
Results - Details for Sevgen220725
Confusion Matrix
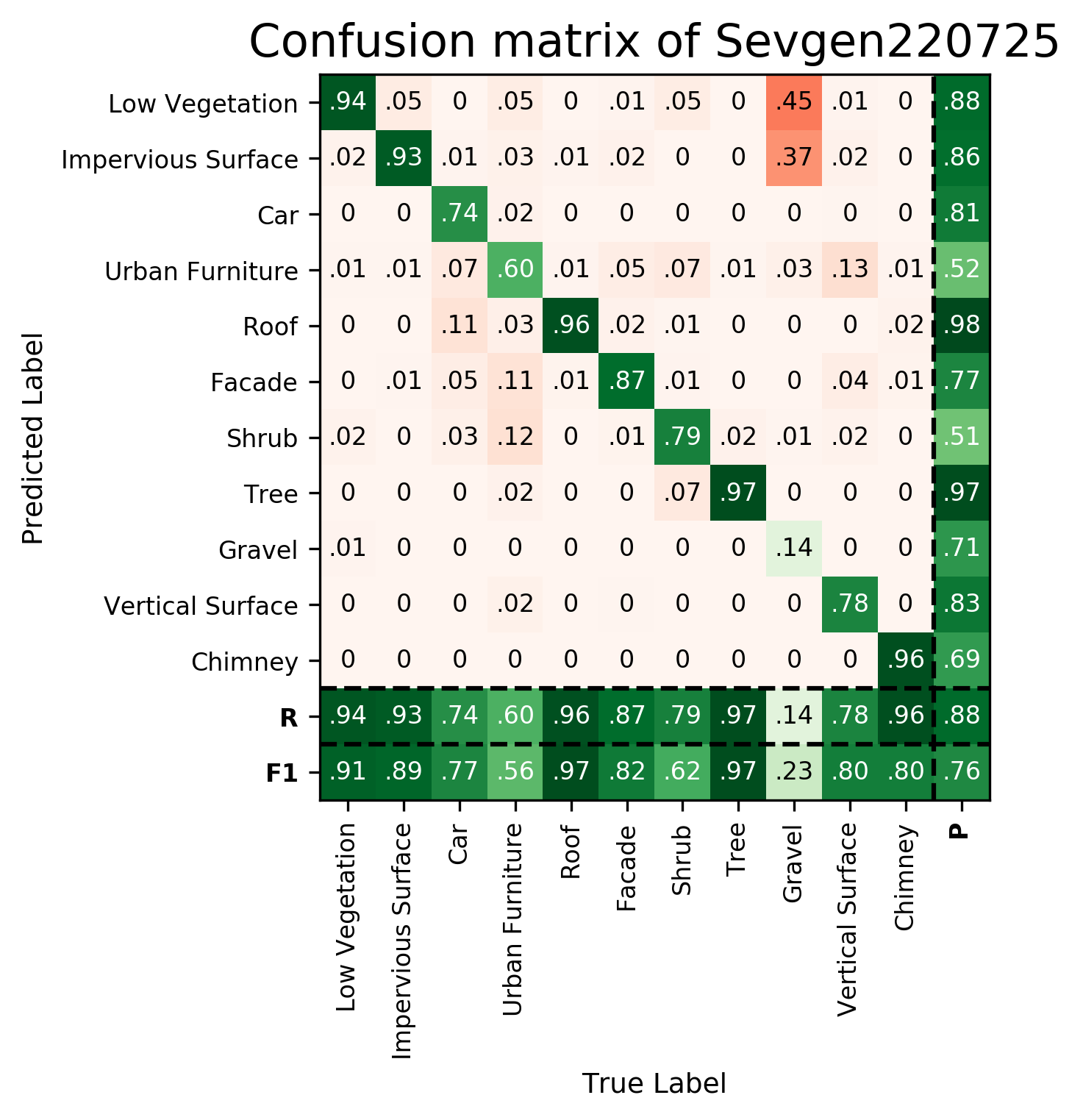
View 1
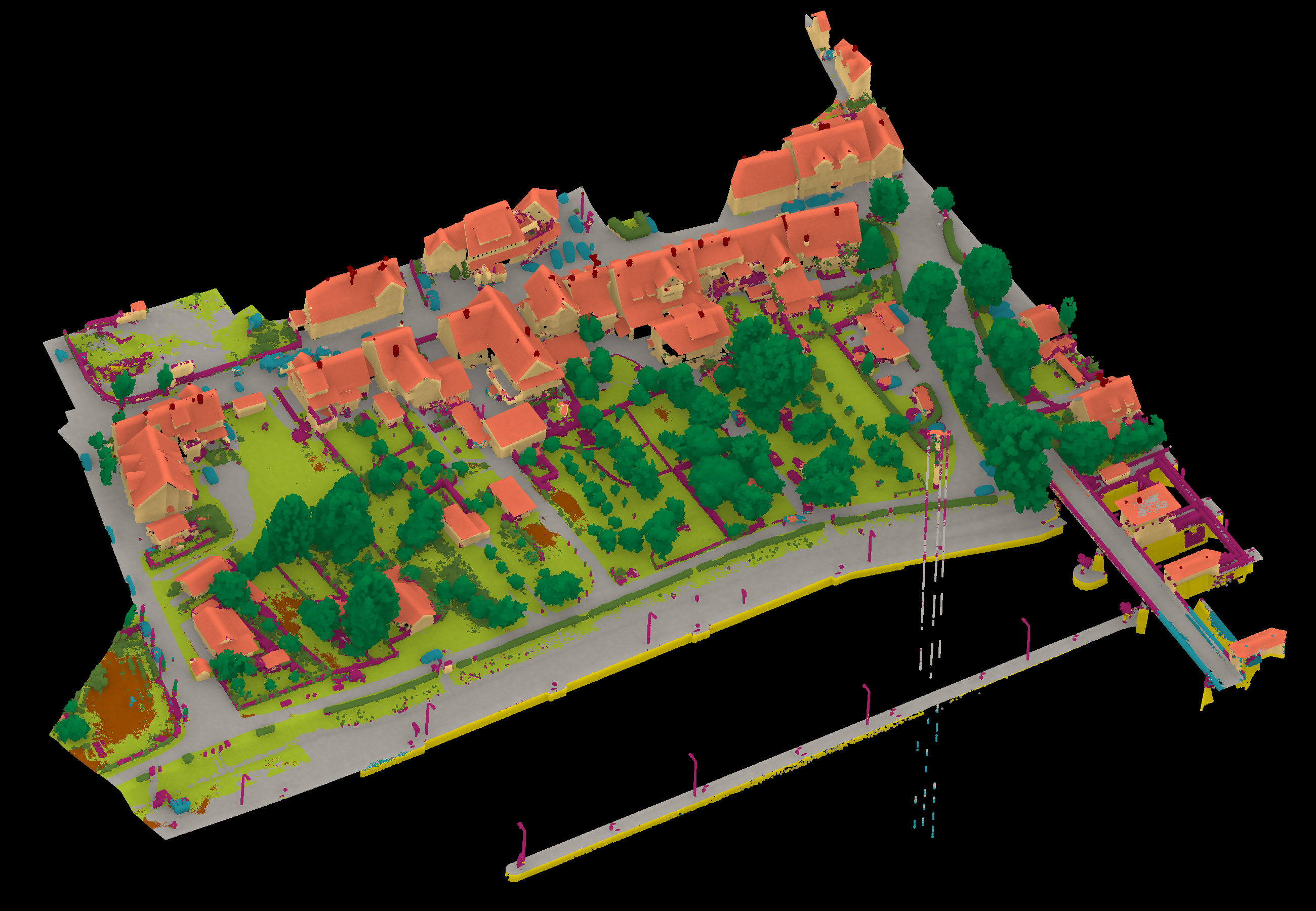
View 2
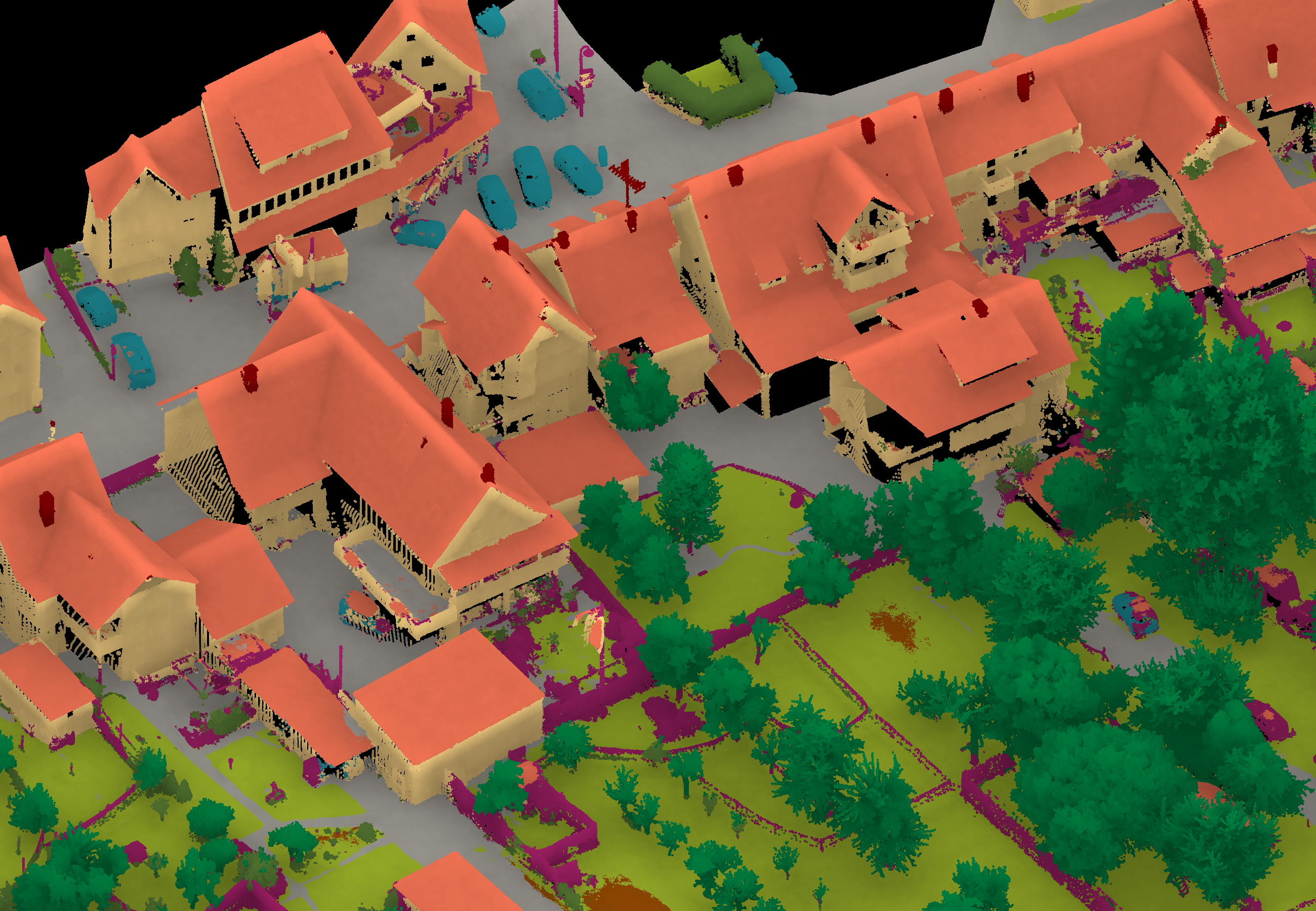
Results - Details for Shi220705
Confusion Matrix
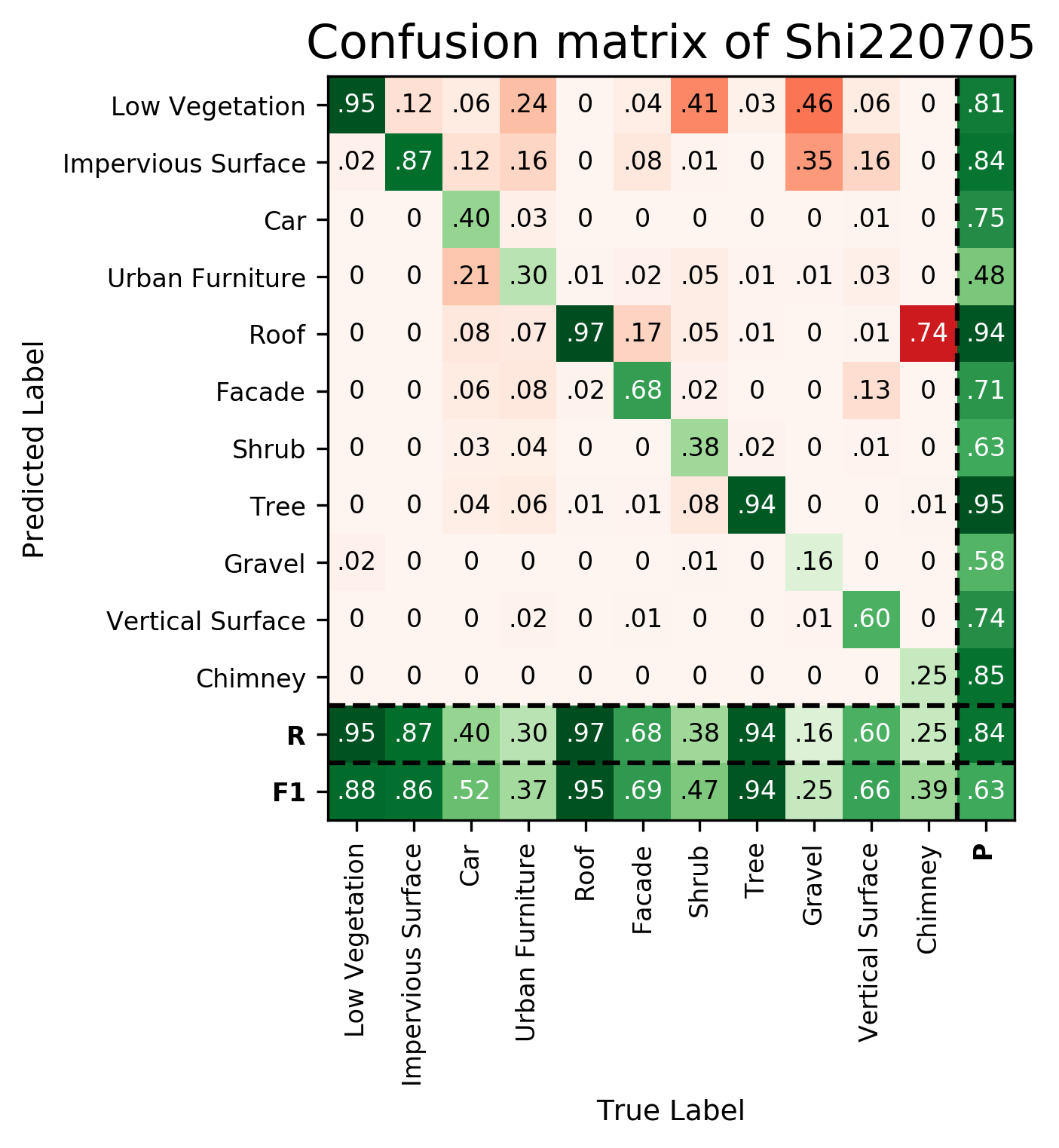
View 1
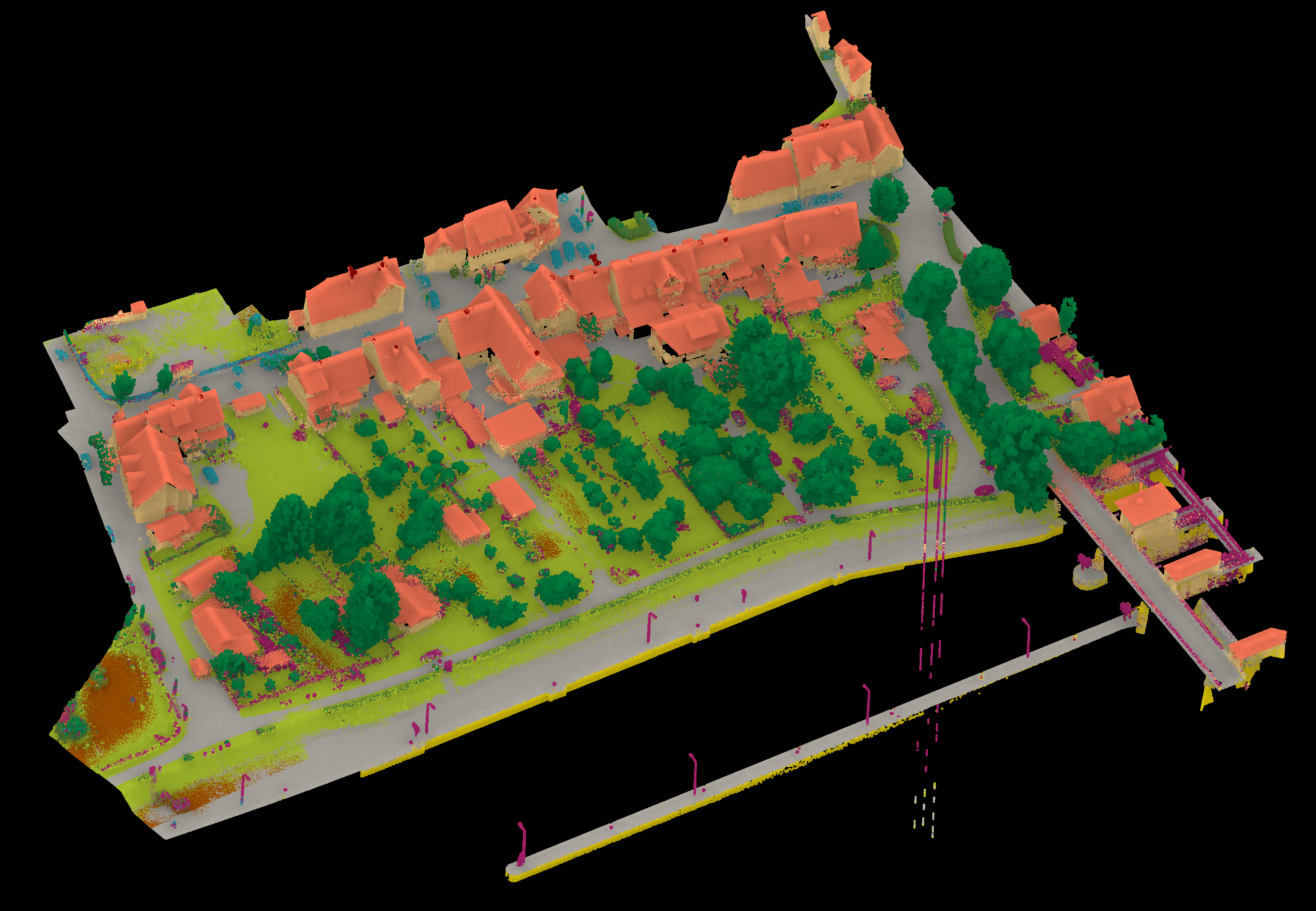
View 2
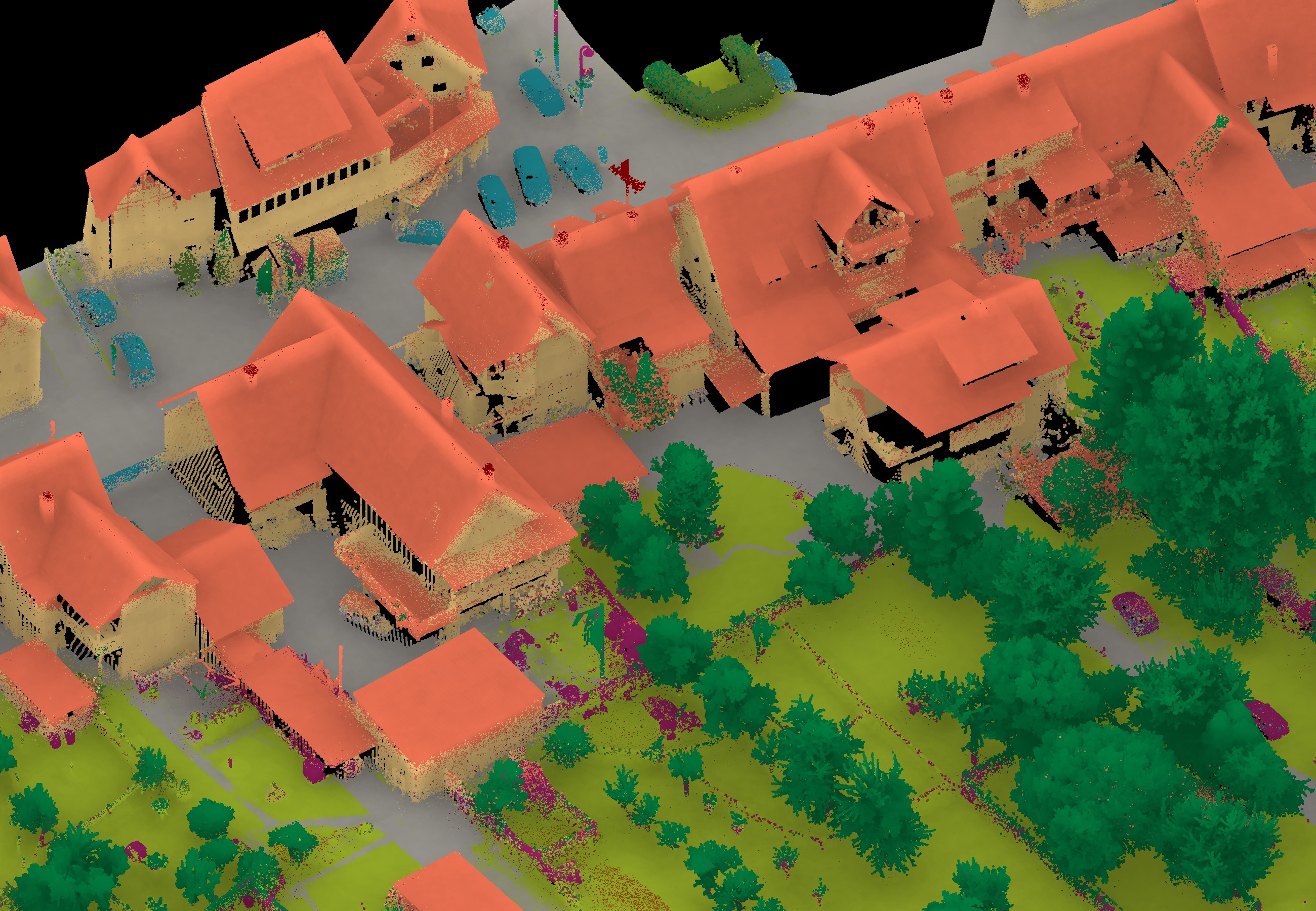
Results - Details for Zhan221025
Confusion Matrix
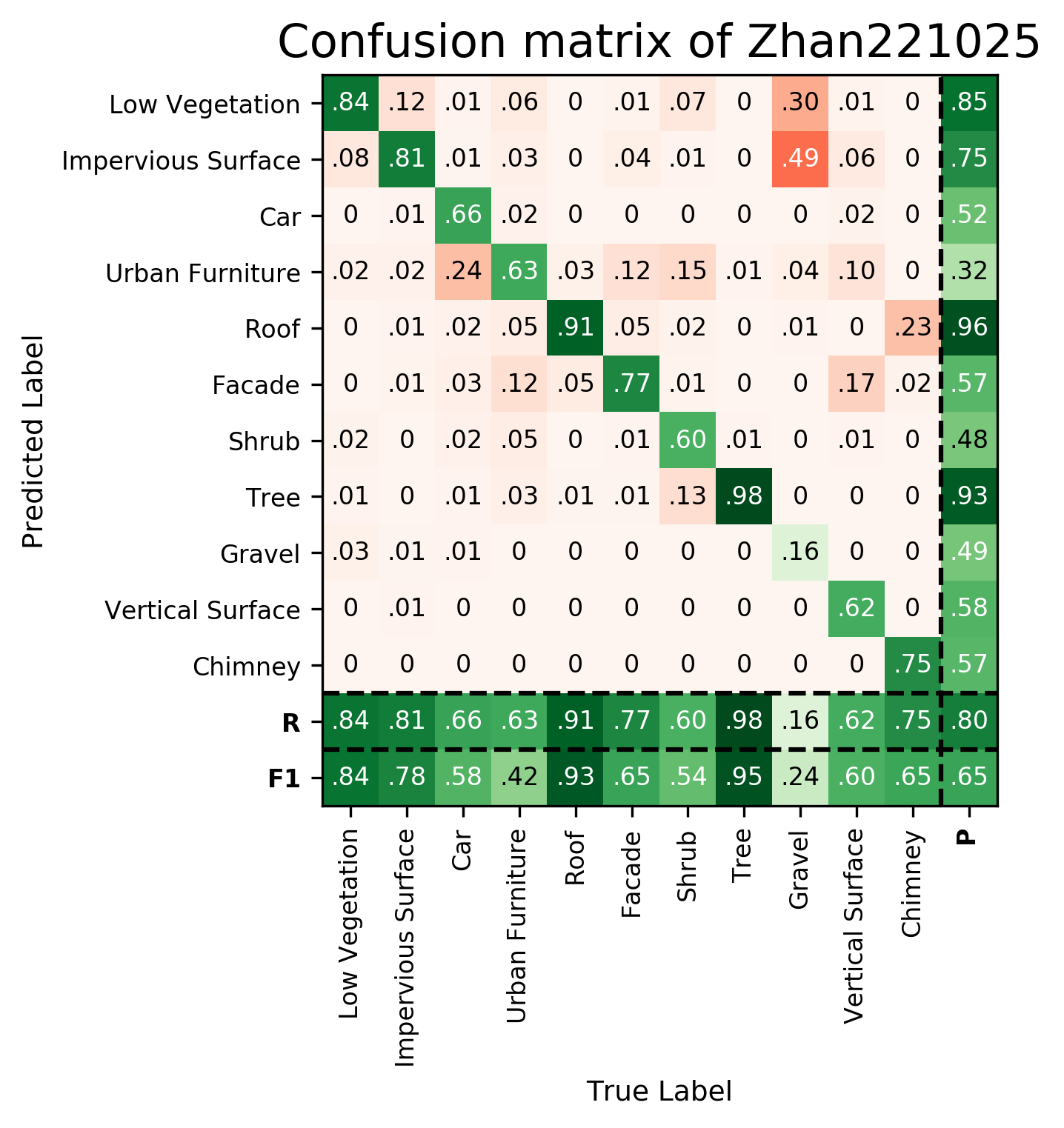
View 1
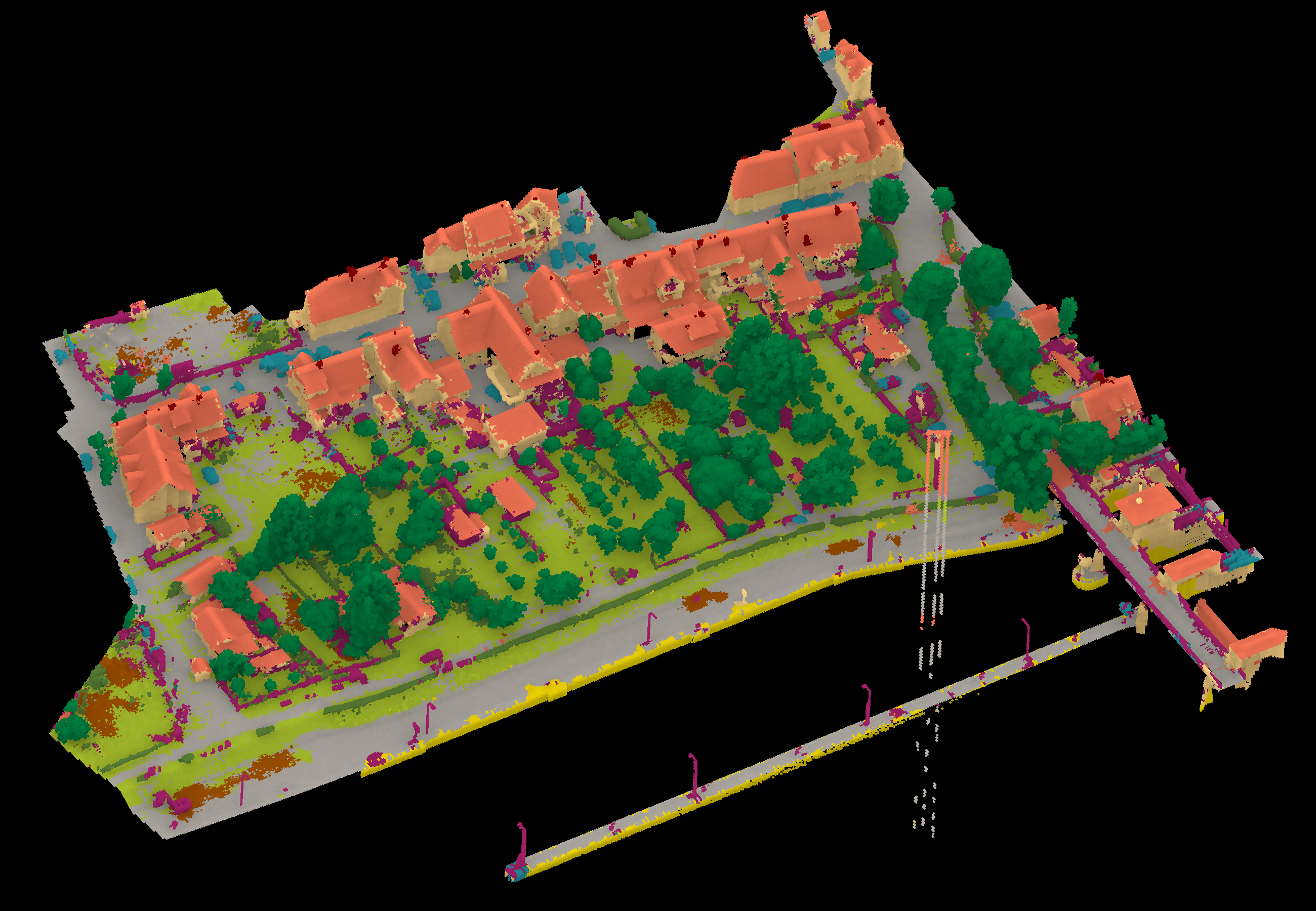
View 2
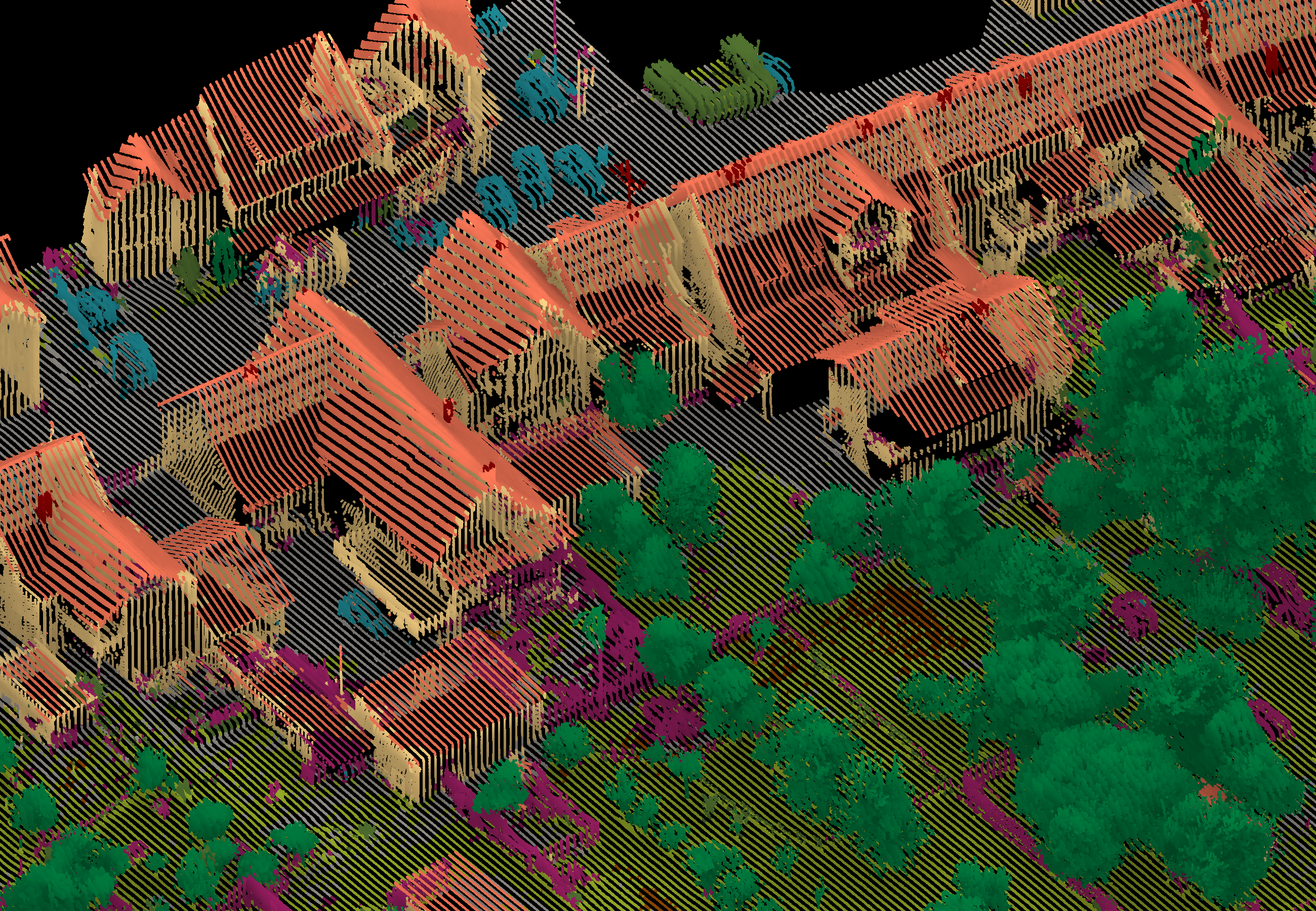
Results - Details for Zhan221112
Confusion Matrix
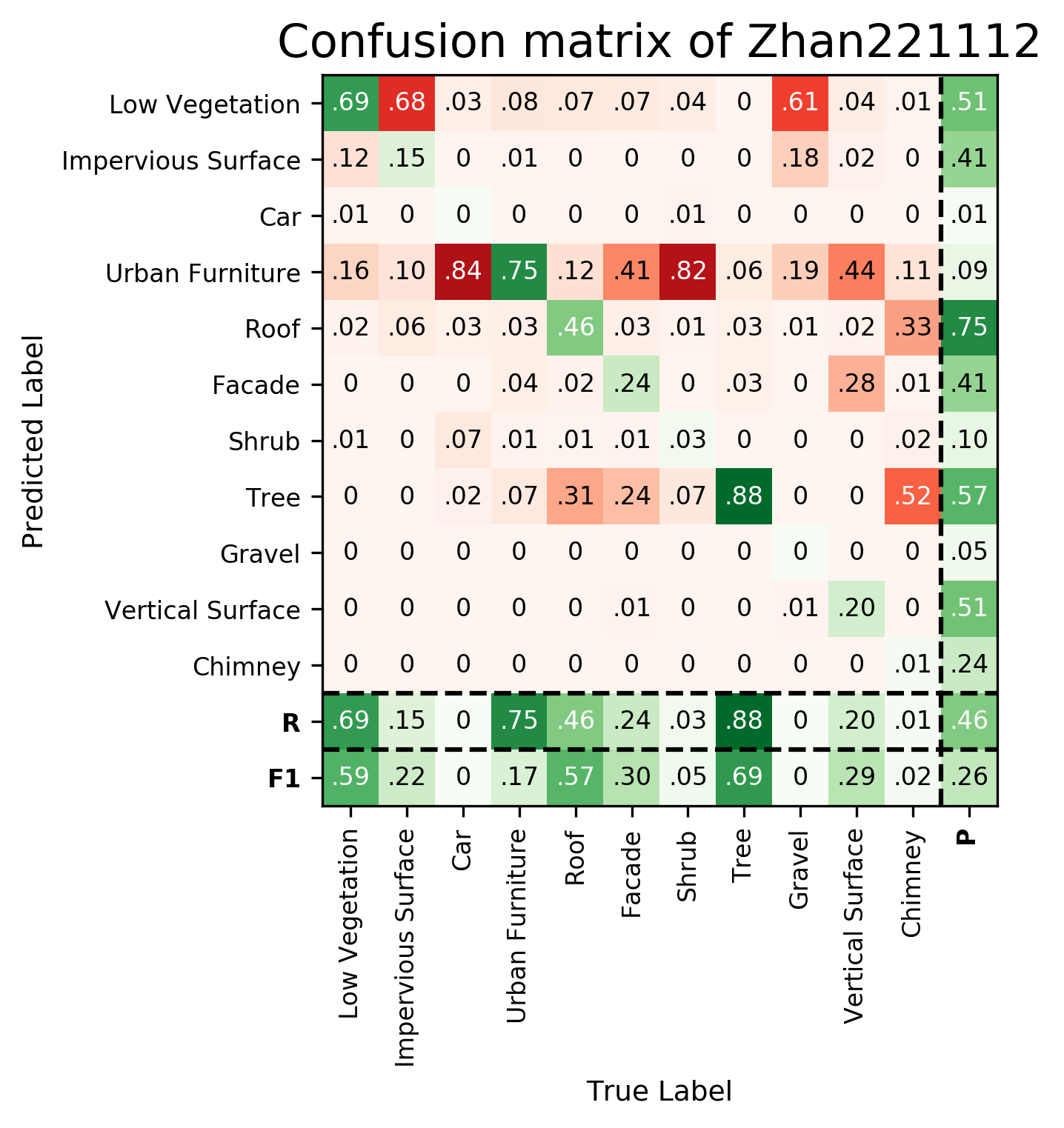
View 1
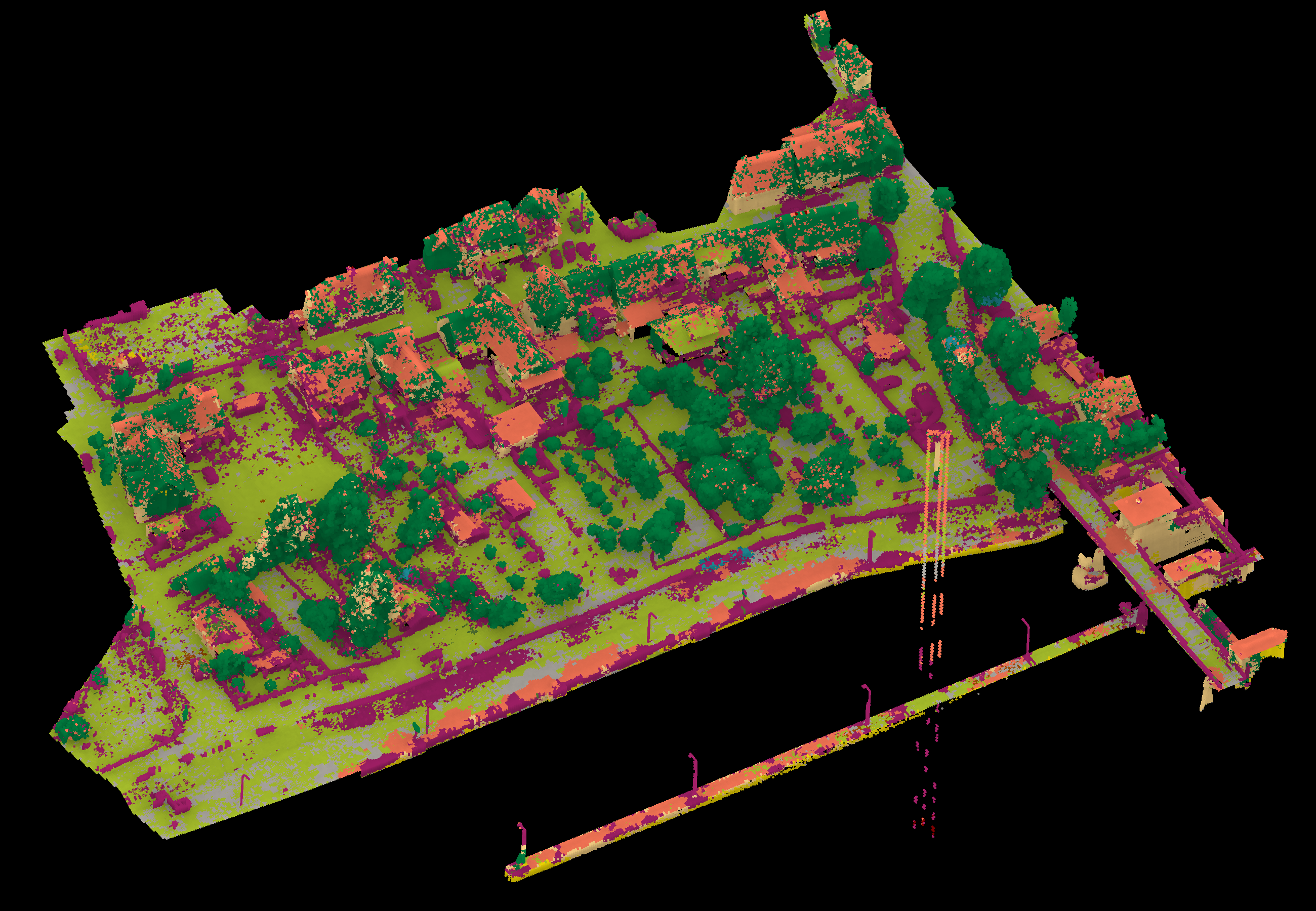
View 2
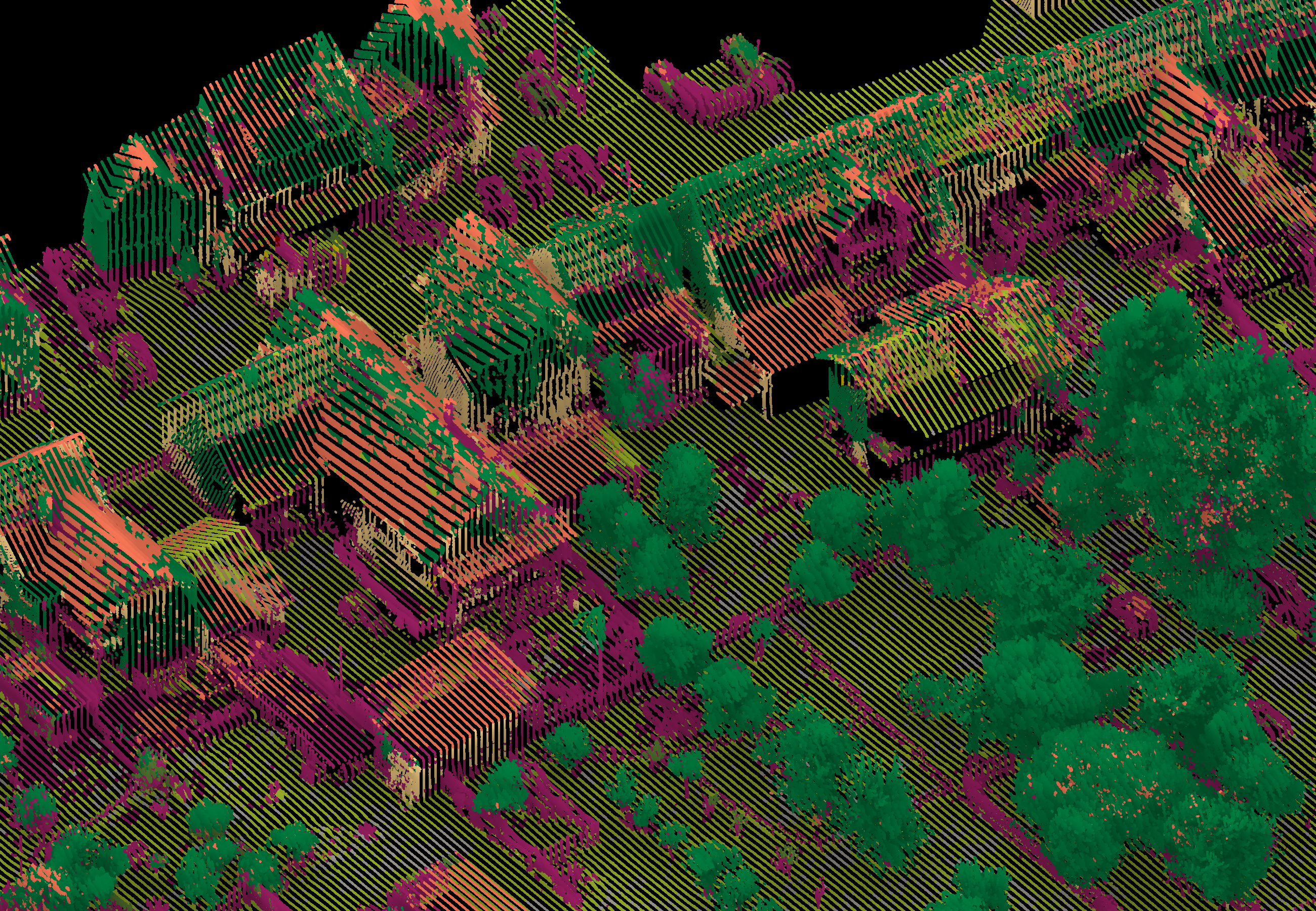
Results - Details for jiabin221114
Confusion Matrix
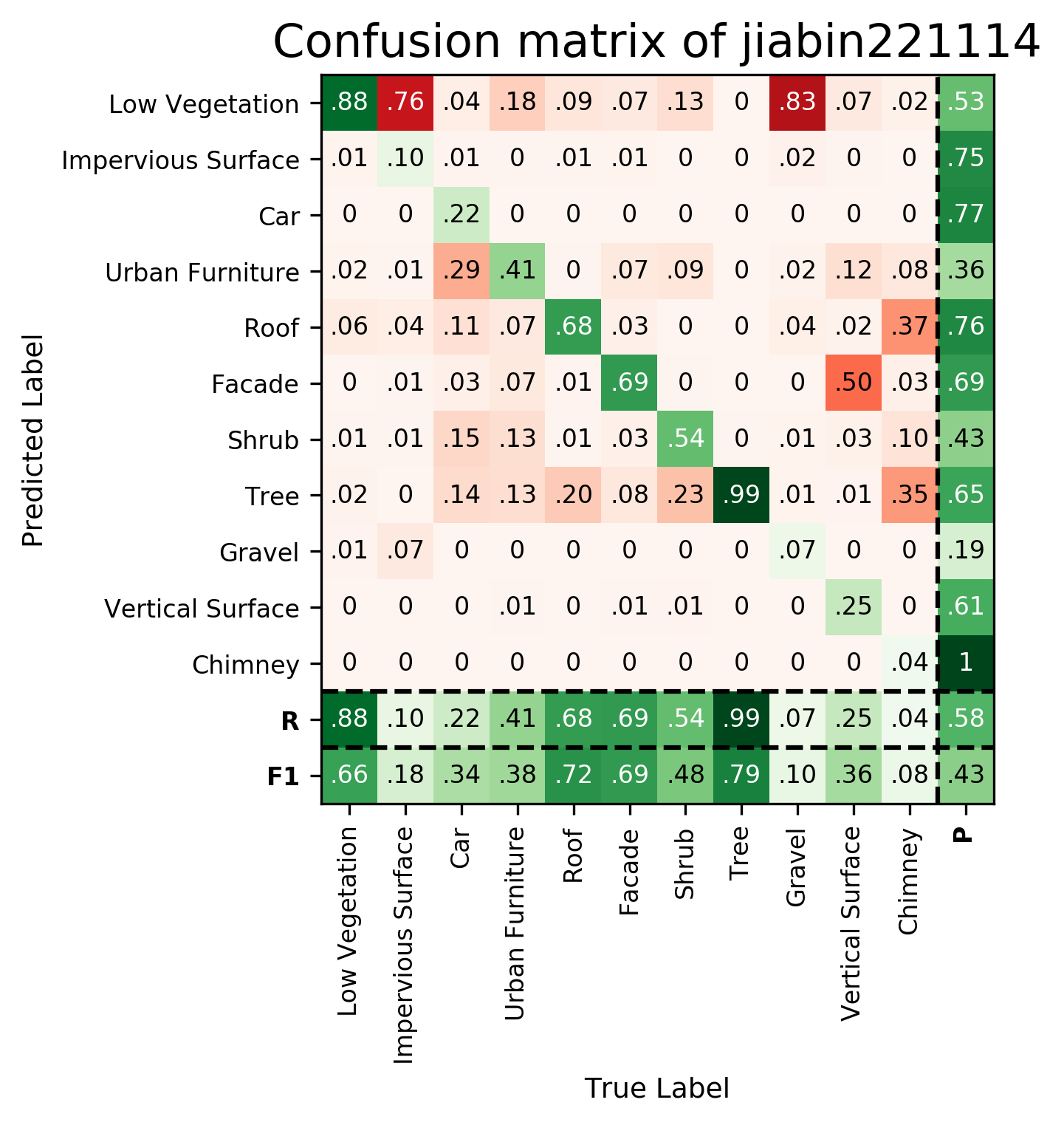
View 1
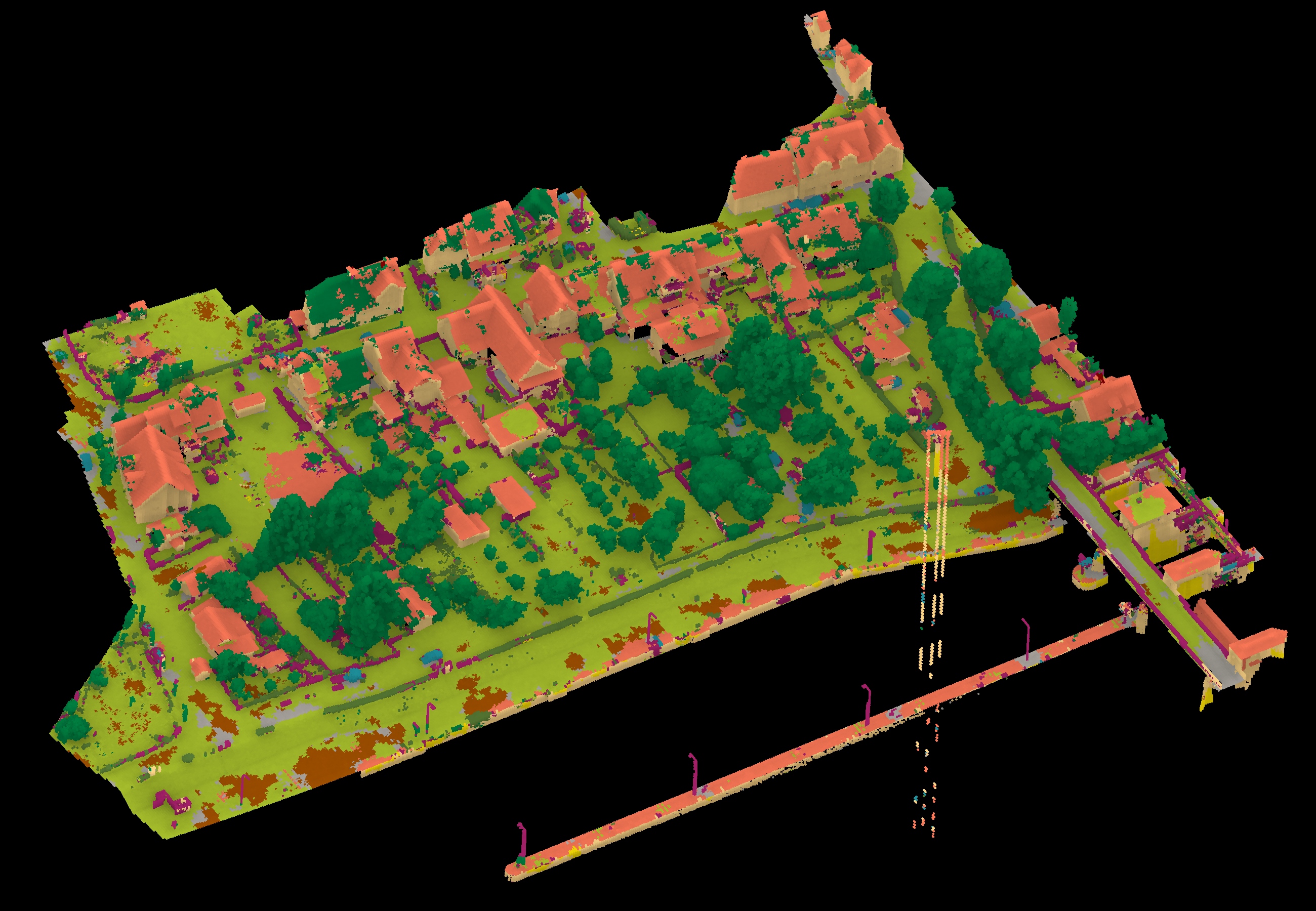
View 2
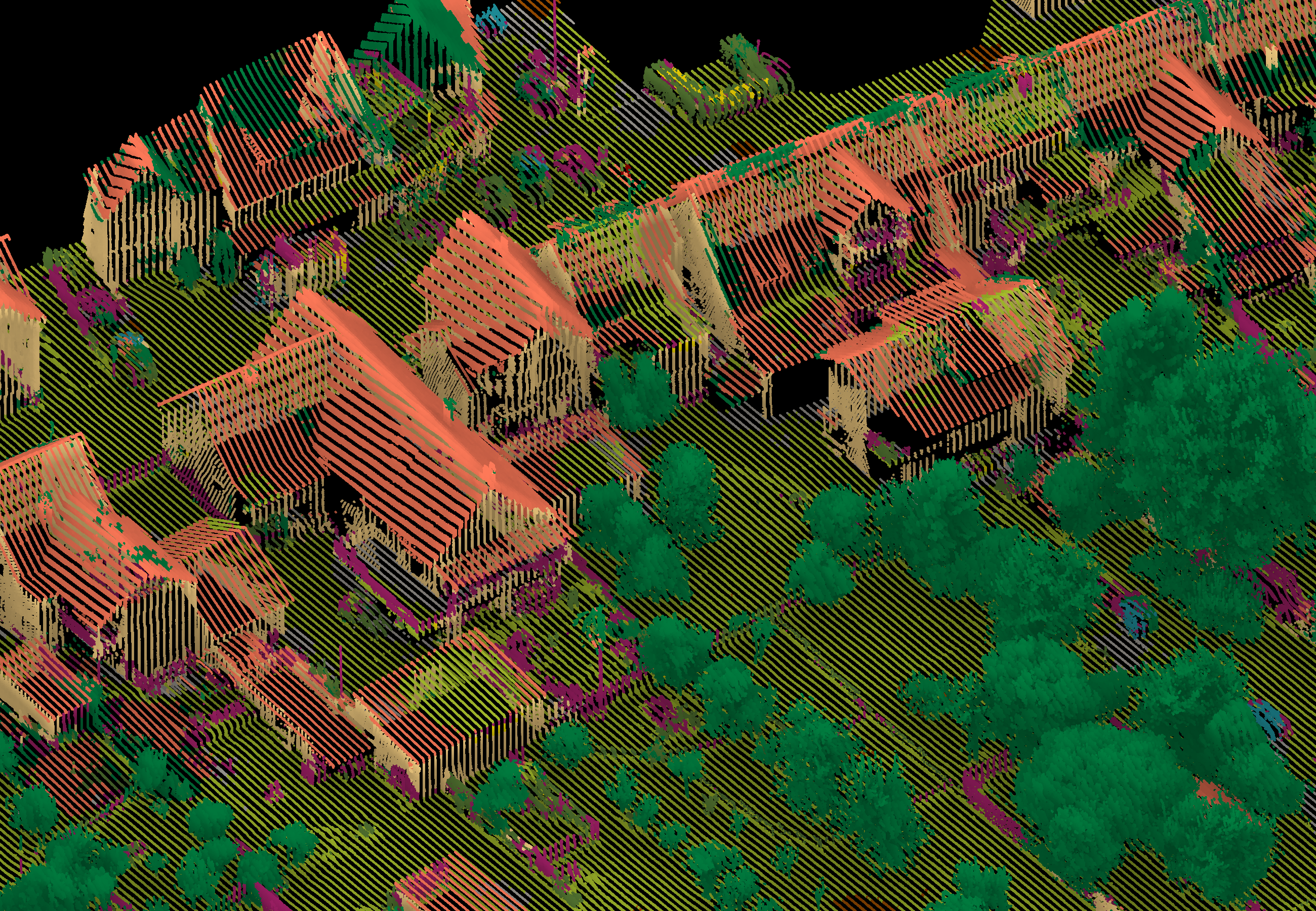
Results - Details for WHU221118
Confusion Matrix
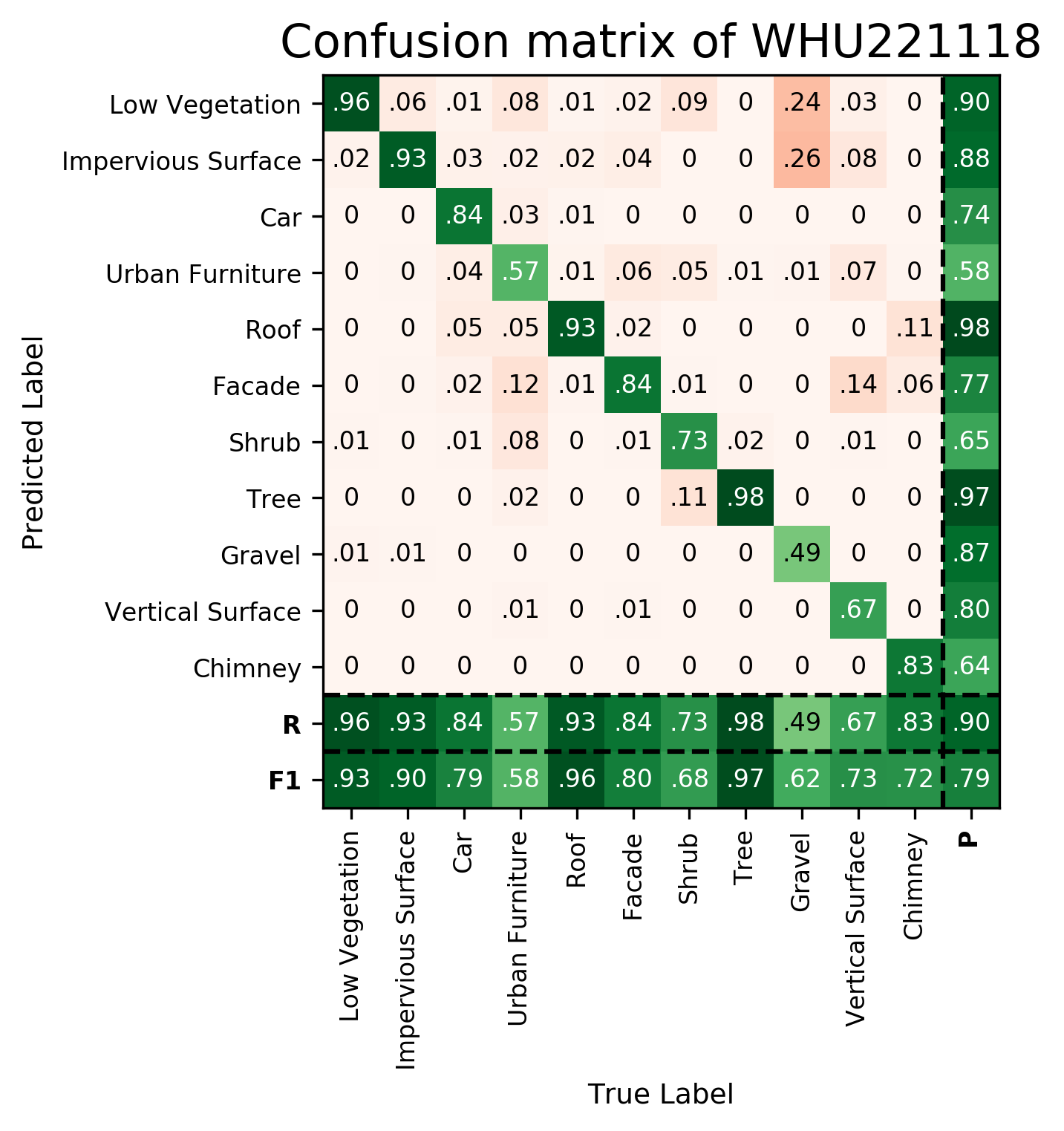
View 1
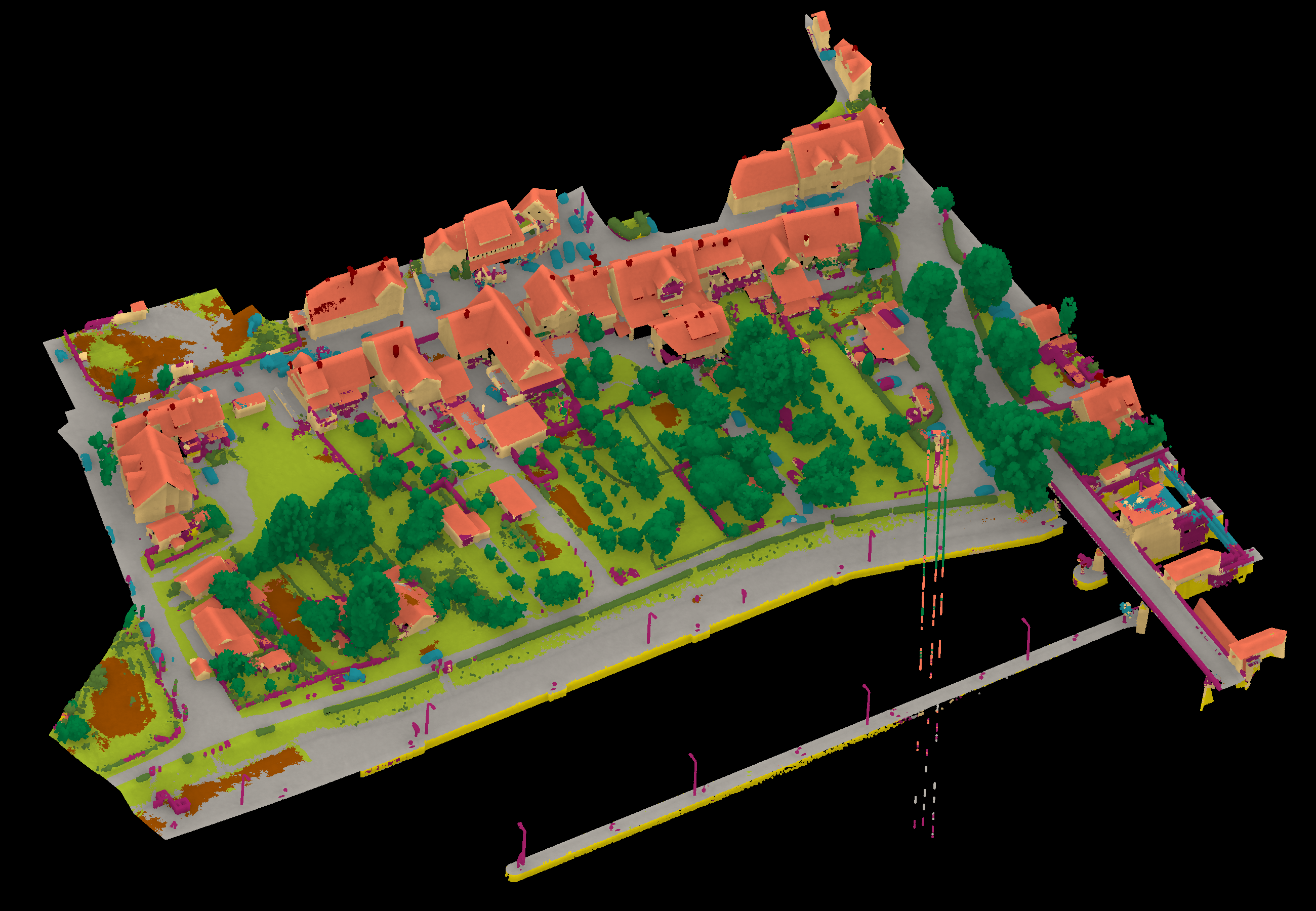
View 2
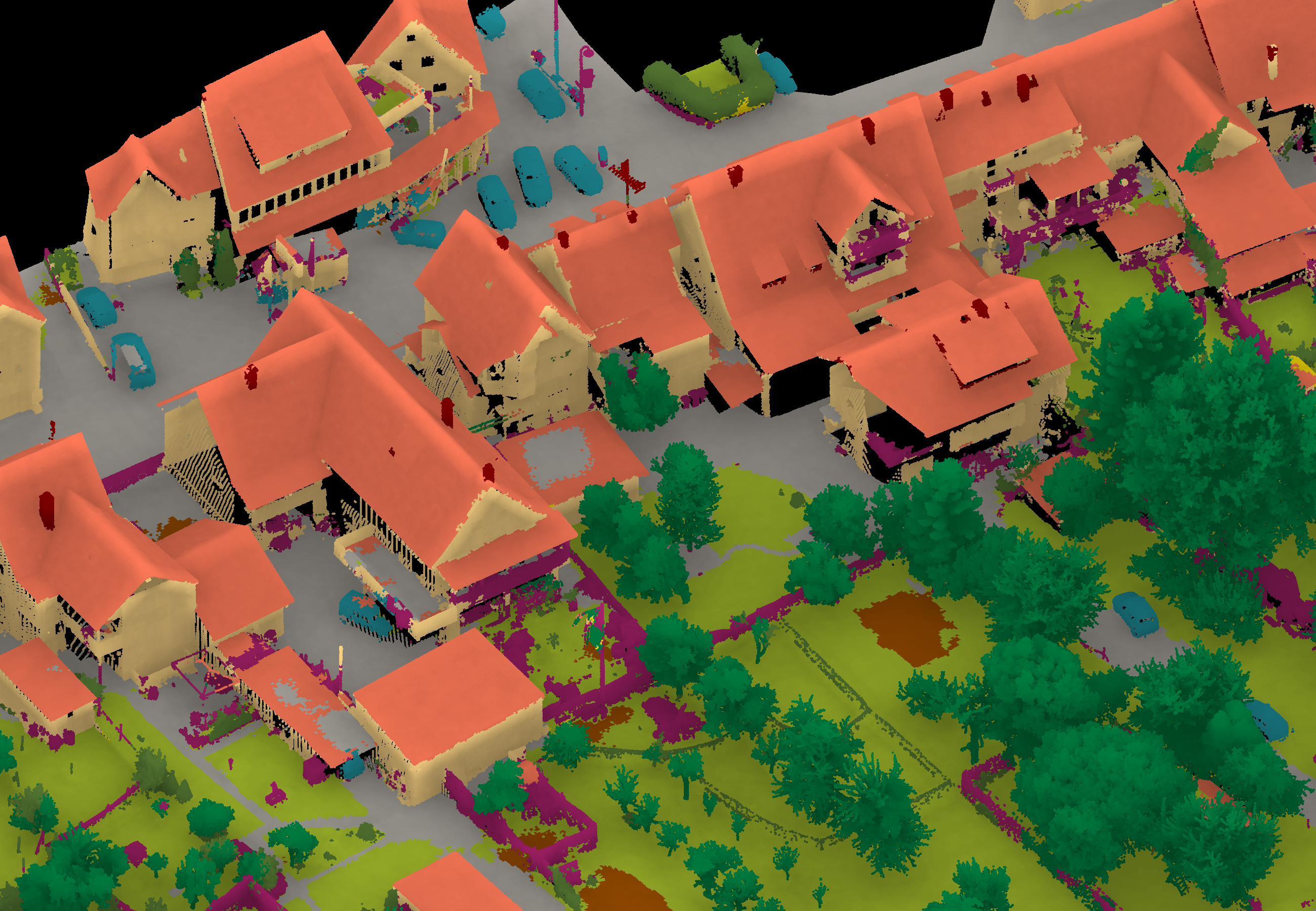
Results - Details for Esmoris230207
Confusion Matrix
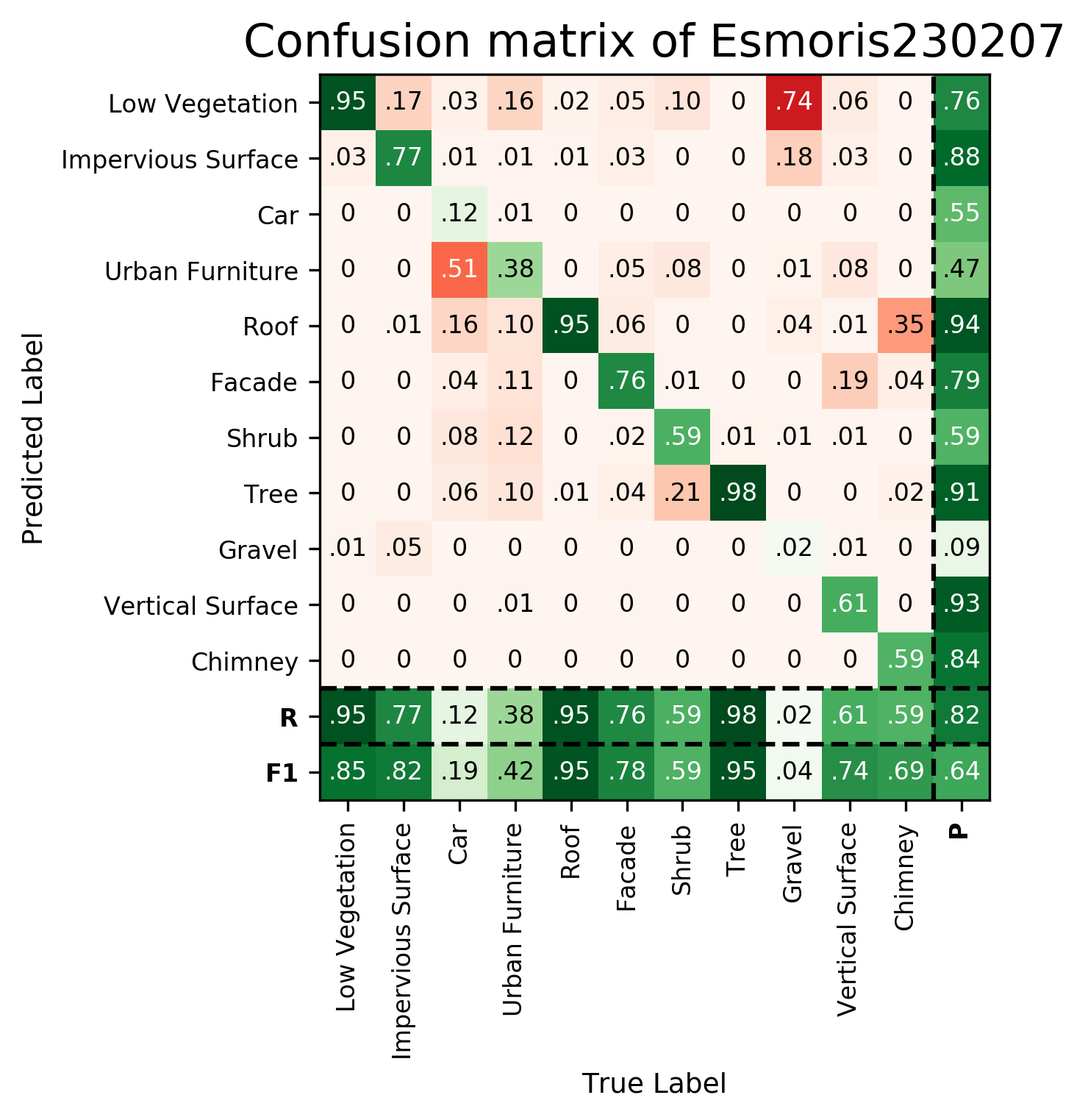
View 1
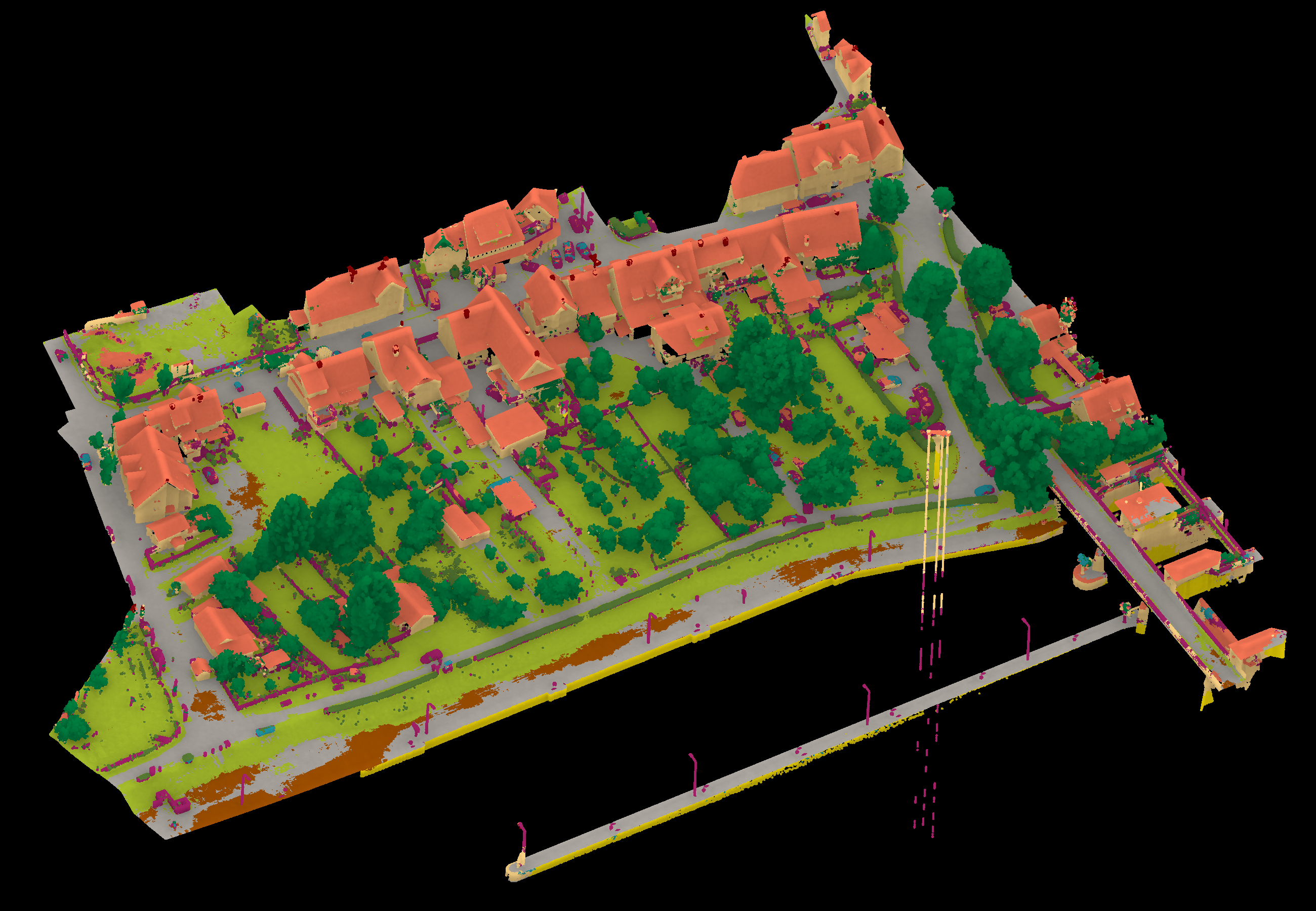
View 2
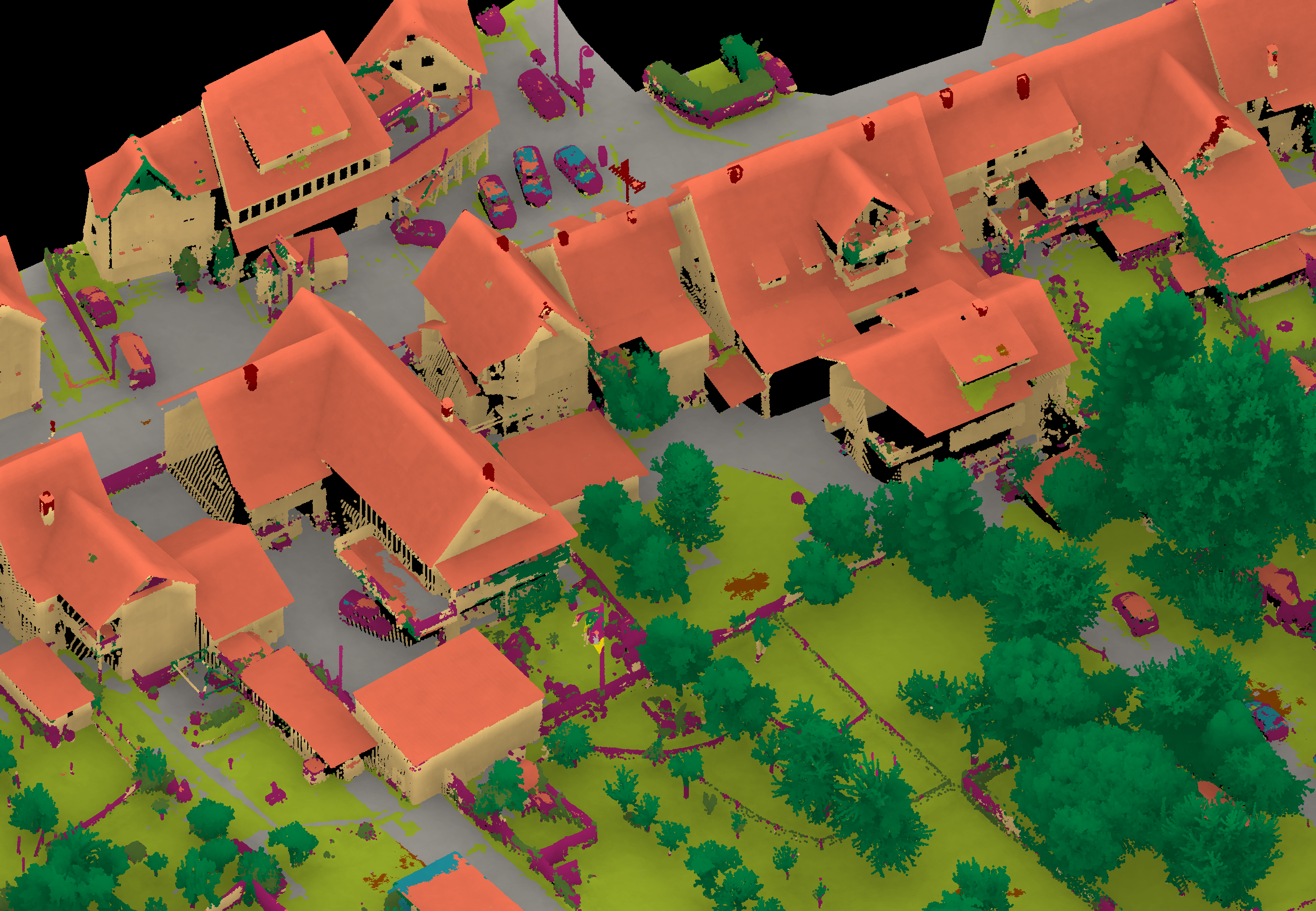
Results - Details for Esmoris230208
Confusion Matrix
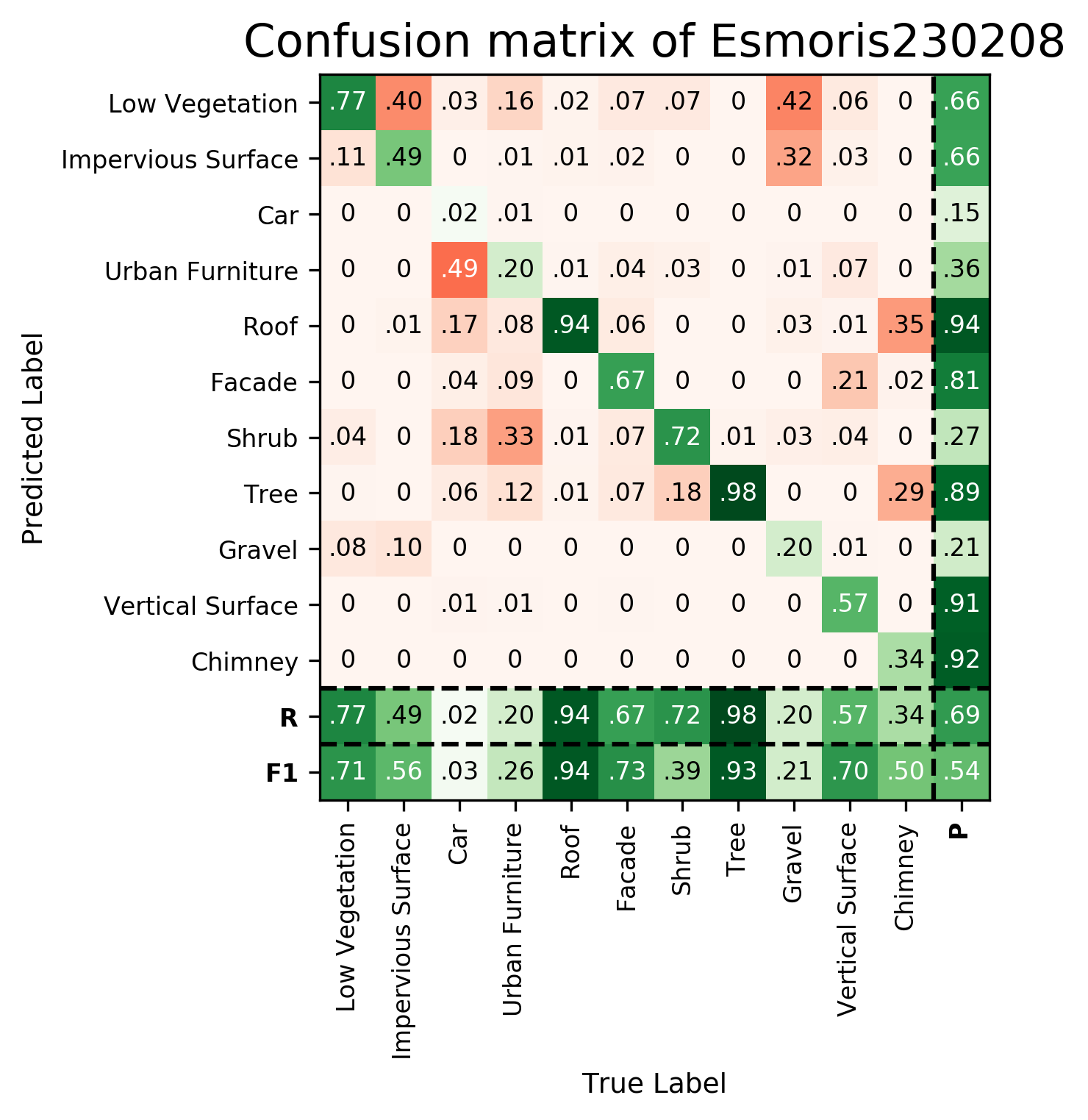
View 1
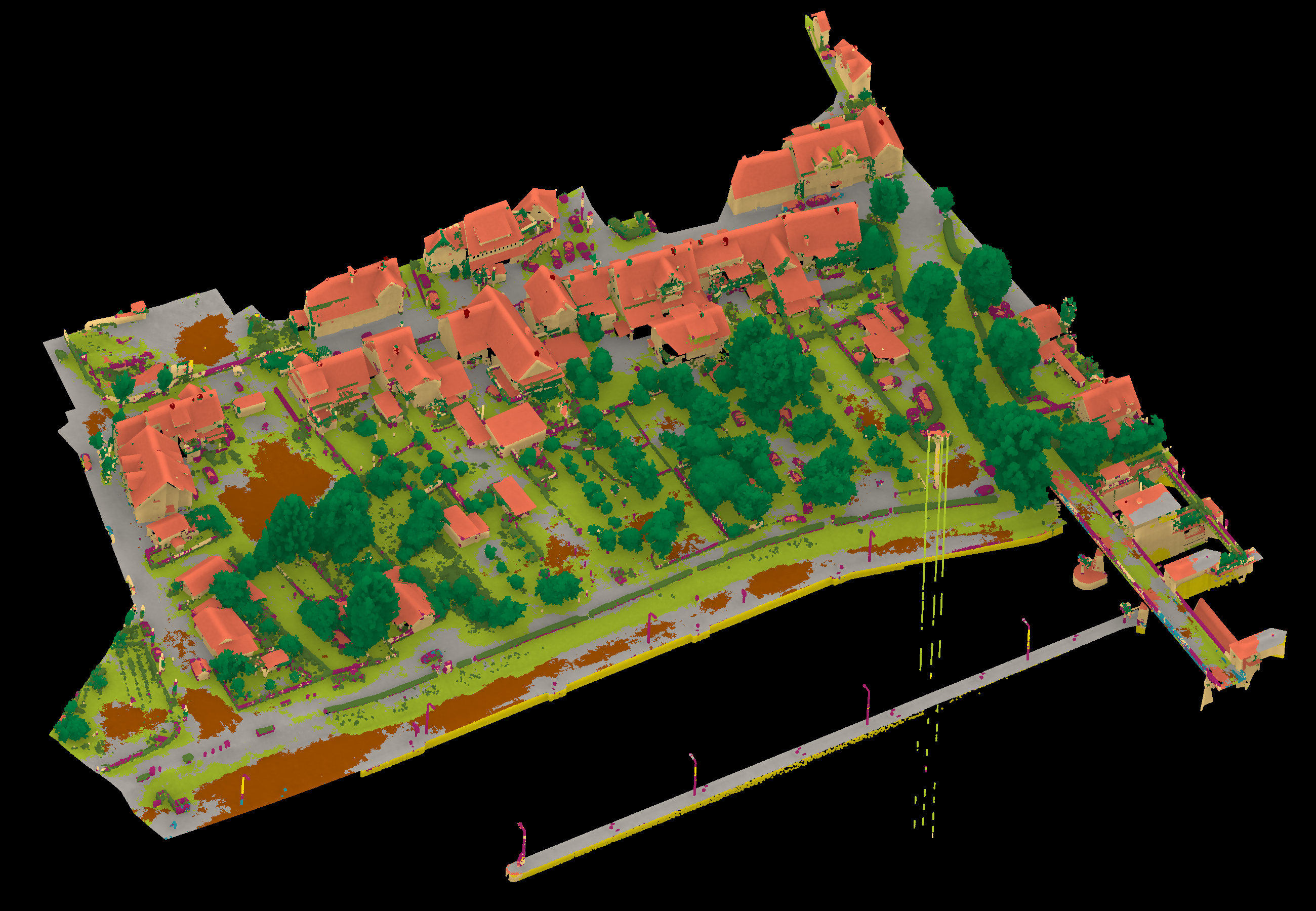
View 2
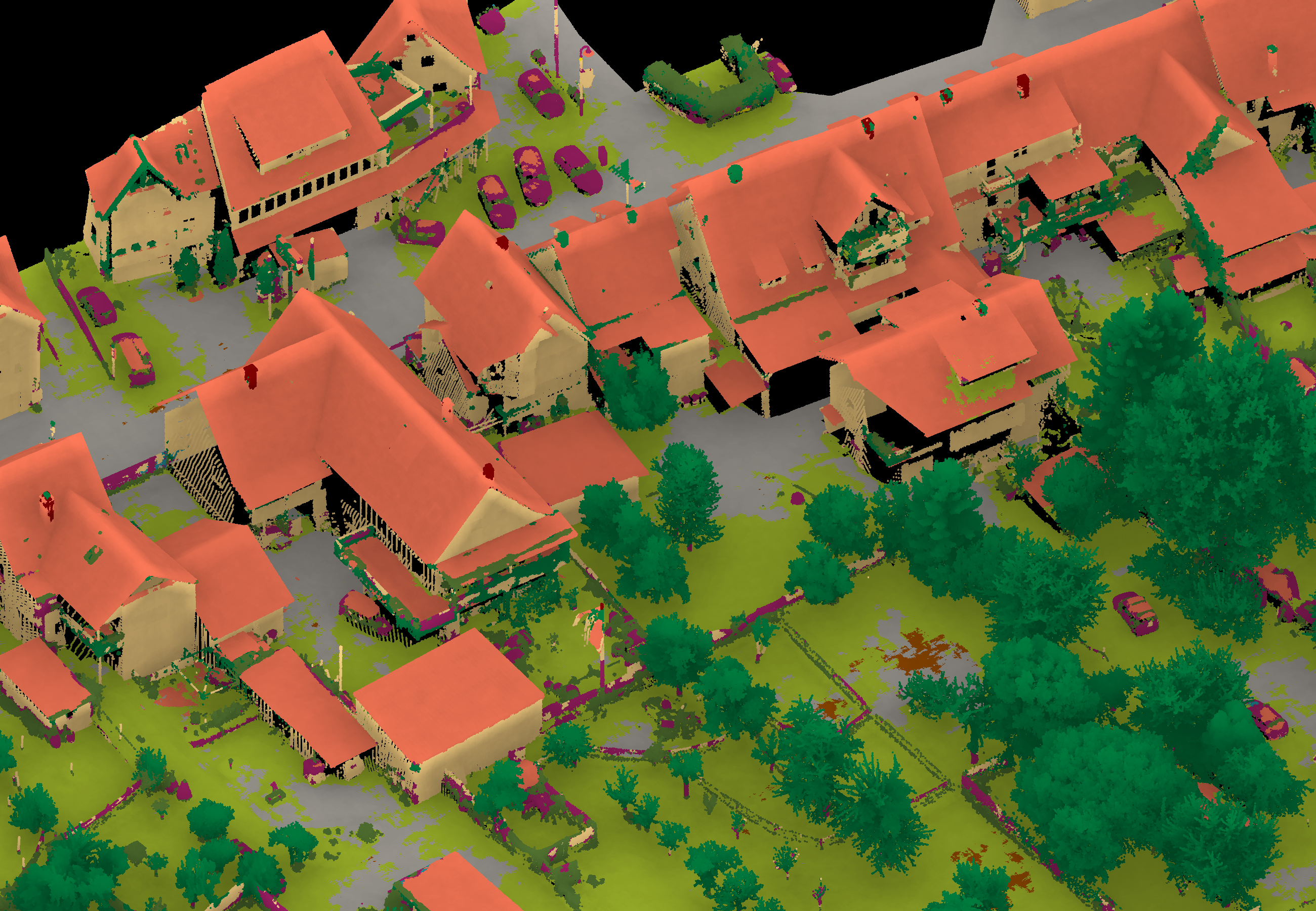
Results - Details for Letard230213
Confusion Matrix
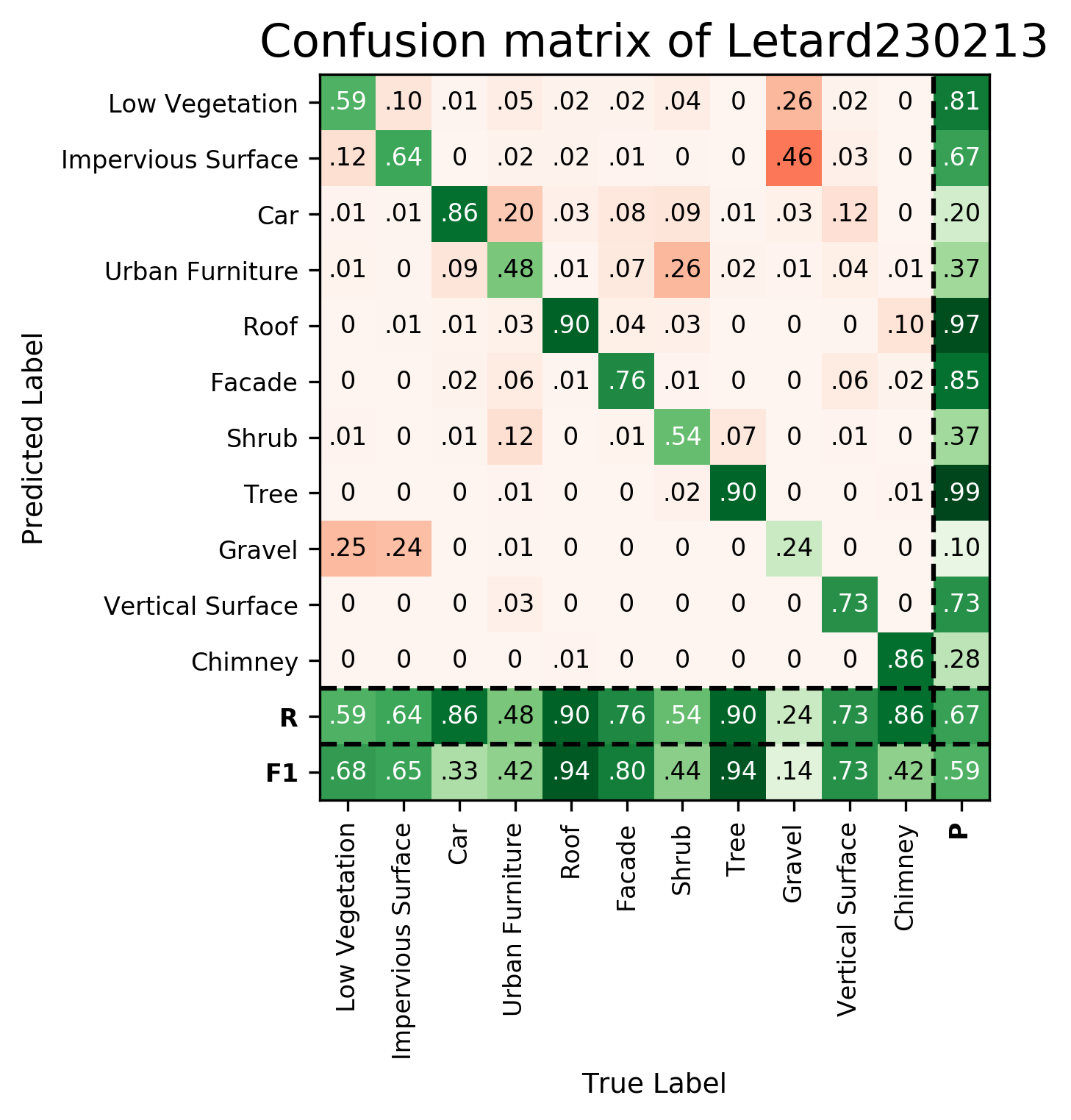
View 1
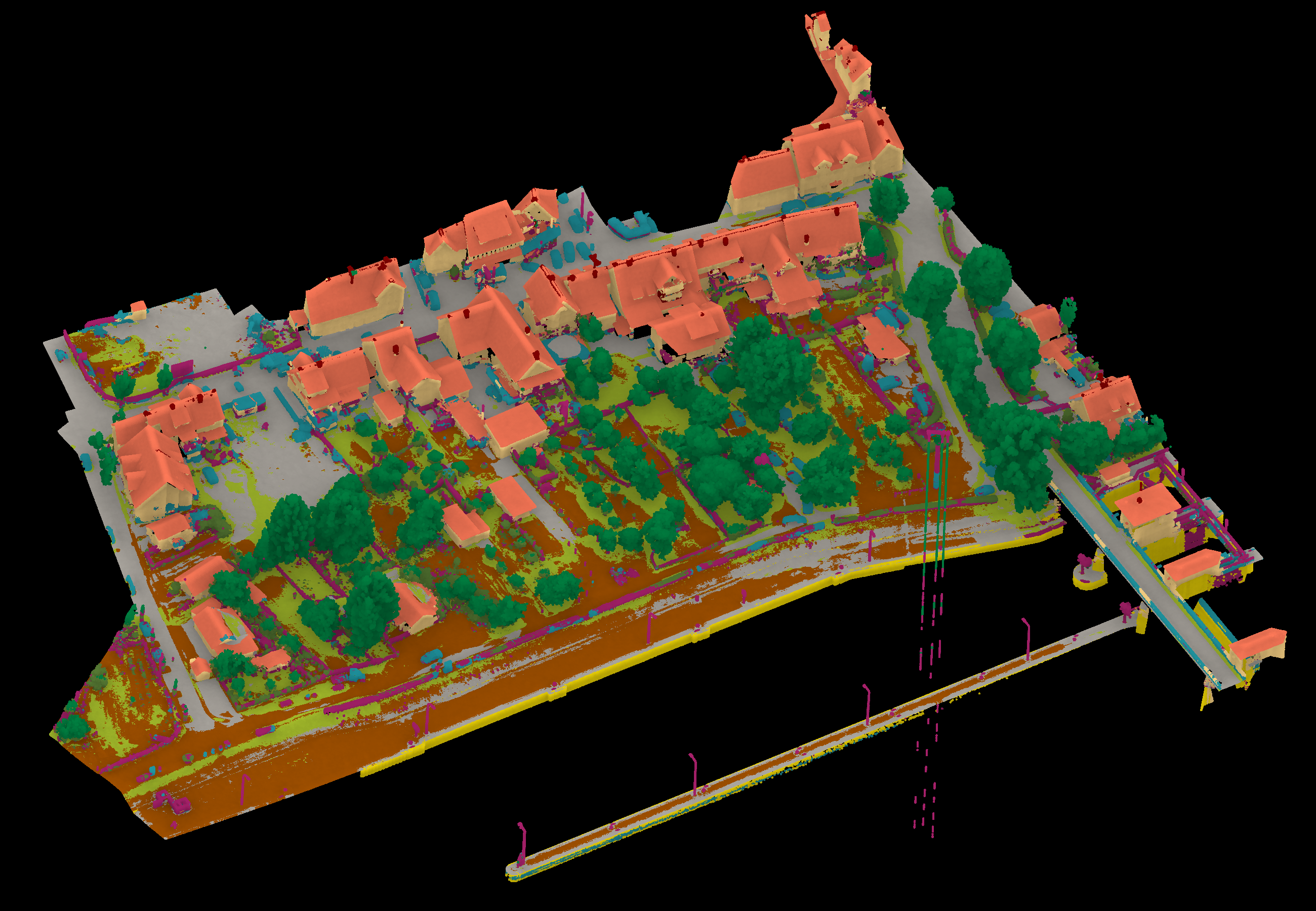
View 2
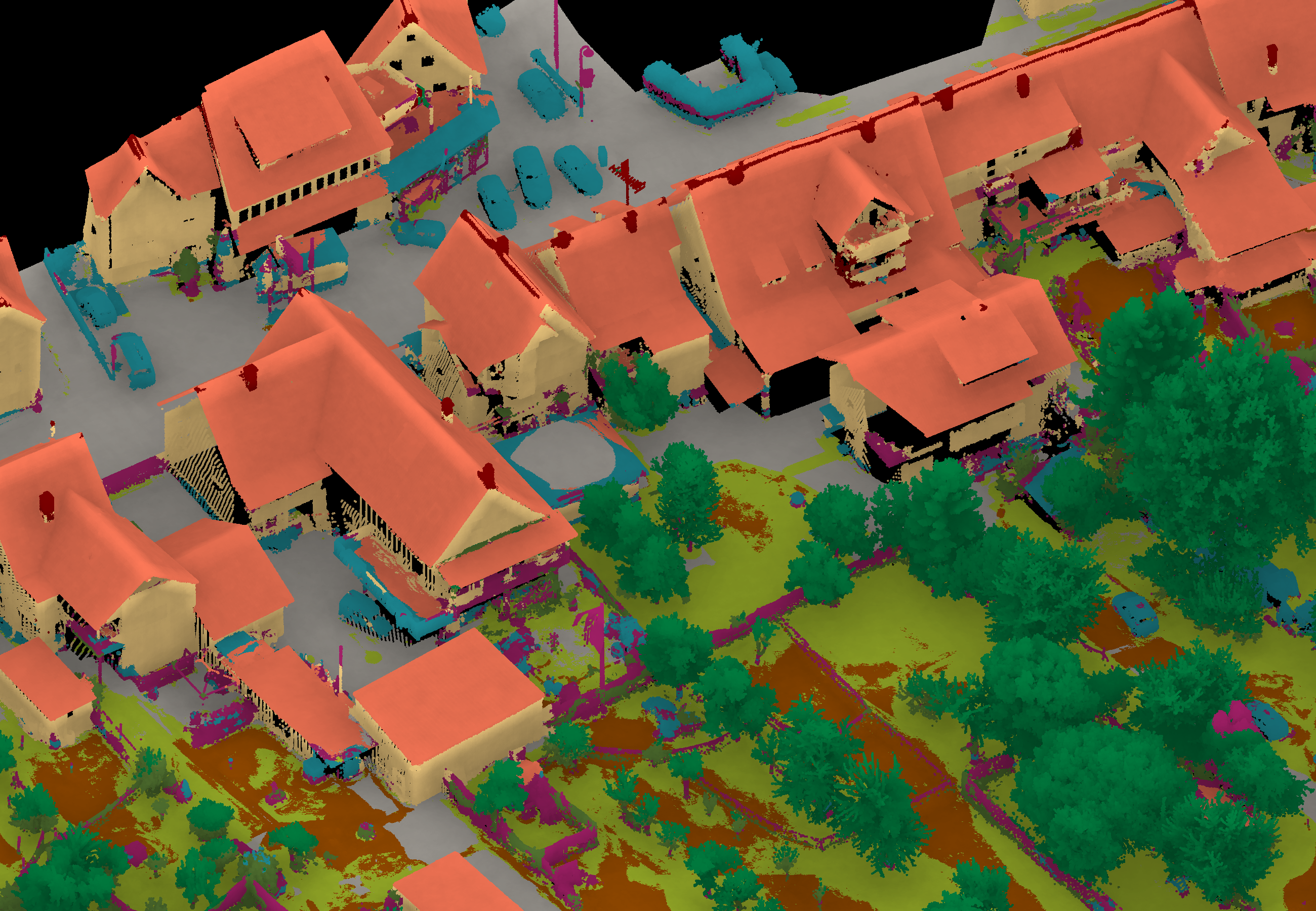
Results - Details for WHU230322
Confusion Matrix
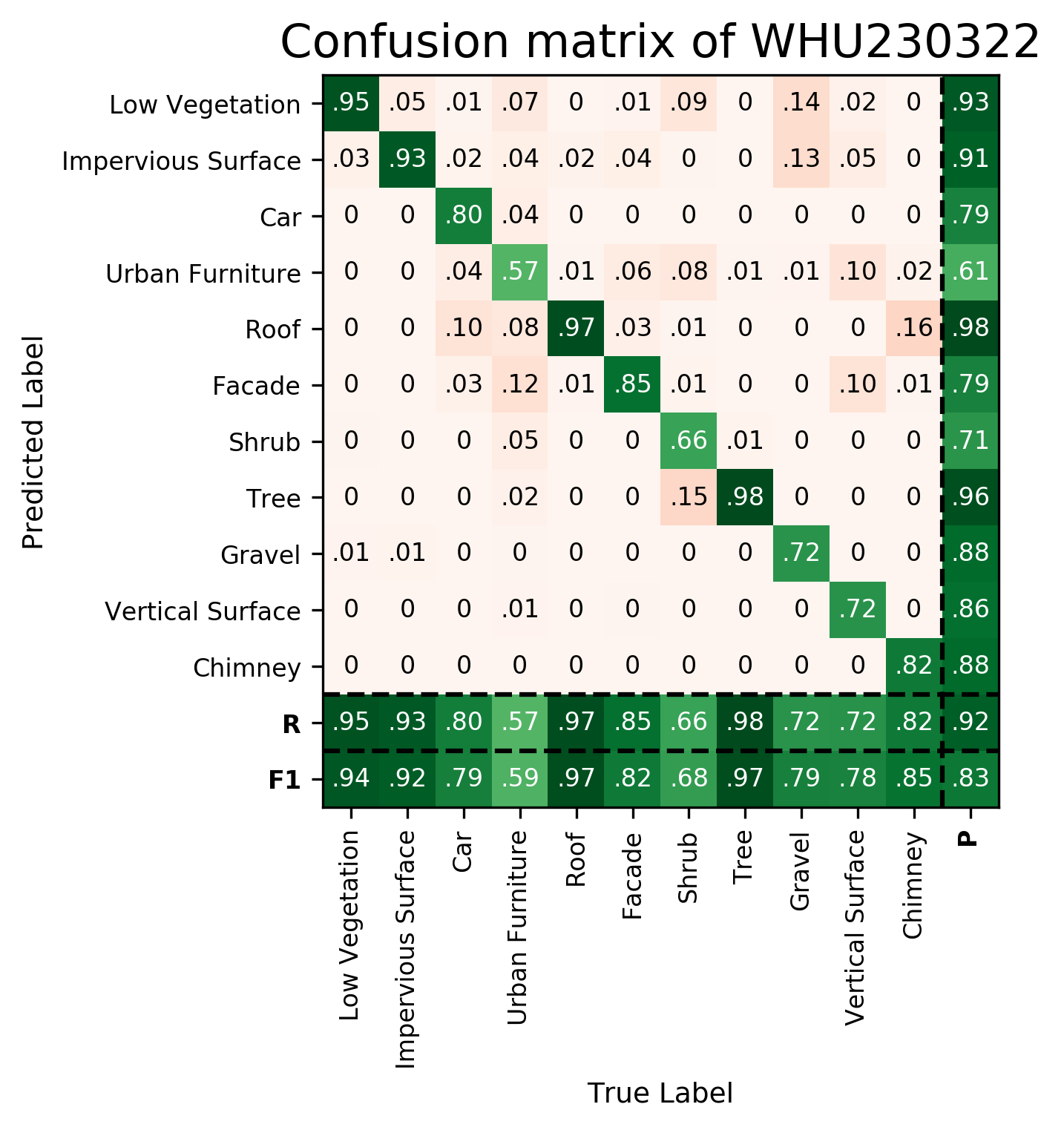
View 1
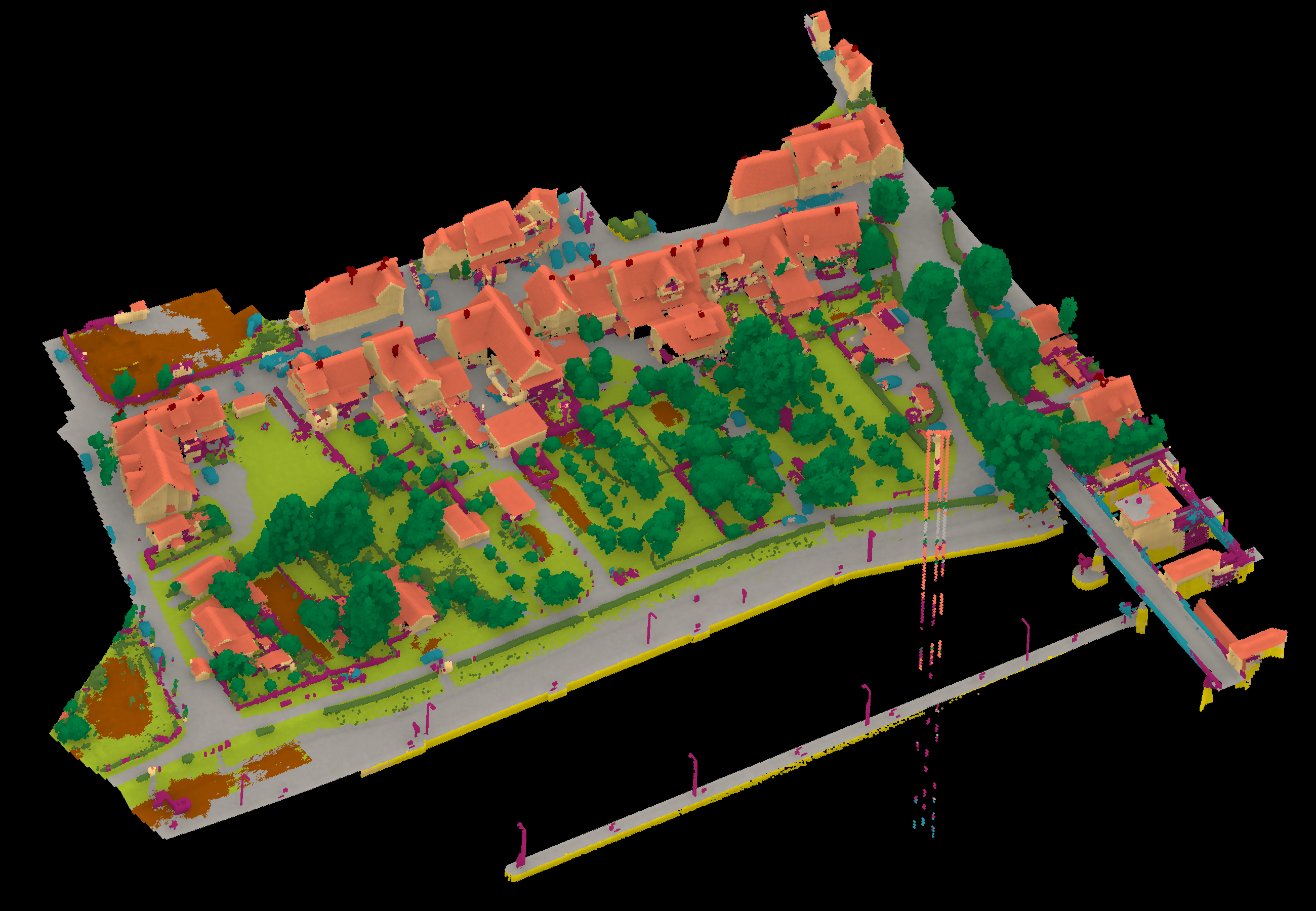
View 2
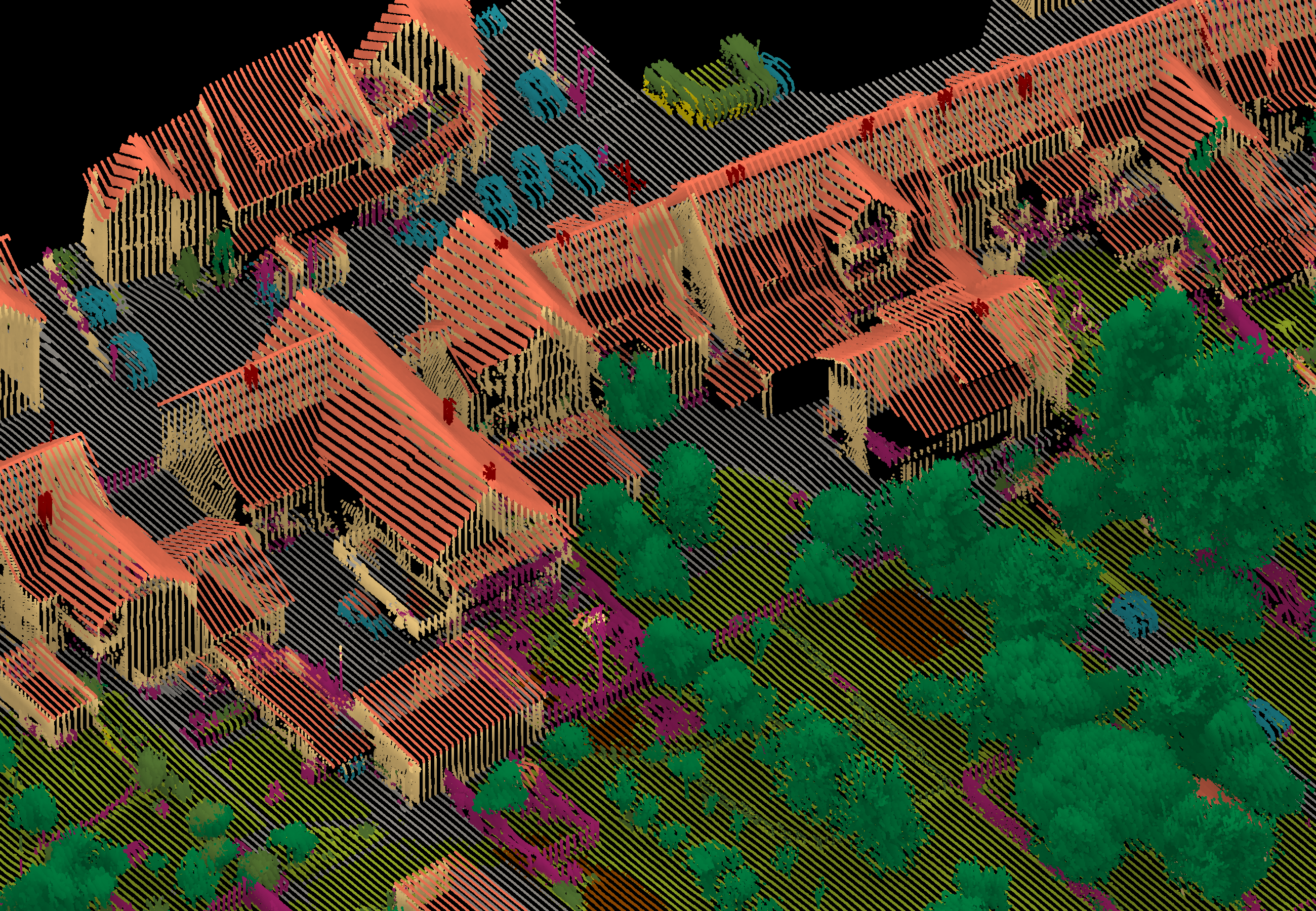
Results - Details for Qiu230831
Confusion Matrix
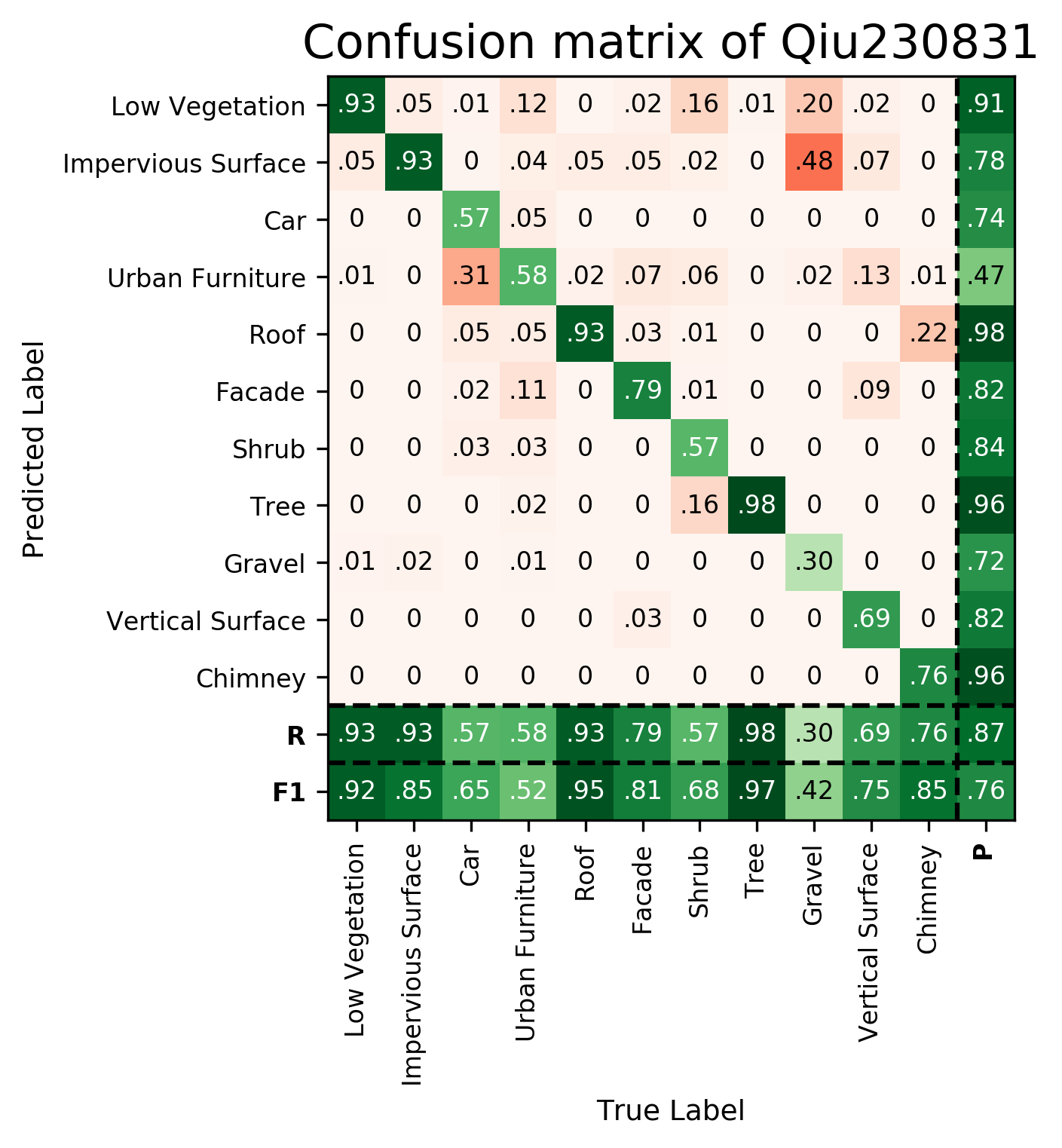
View 1
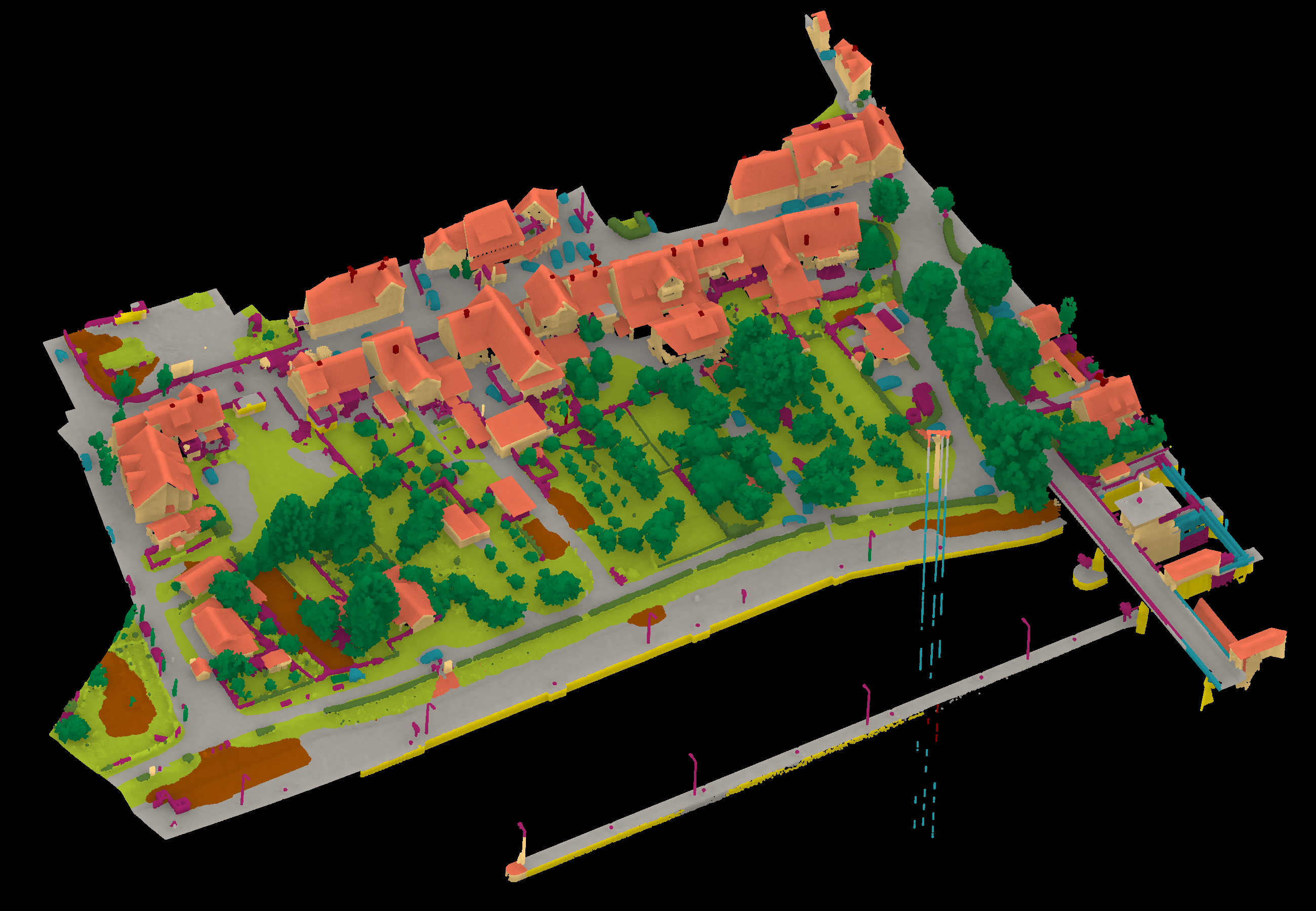
View 2
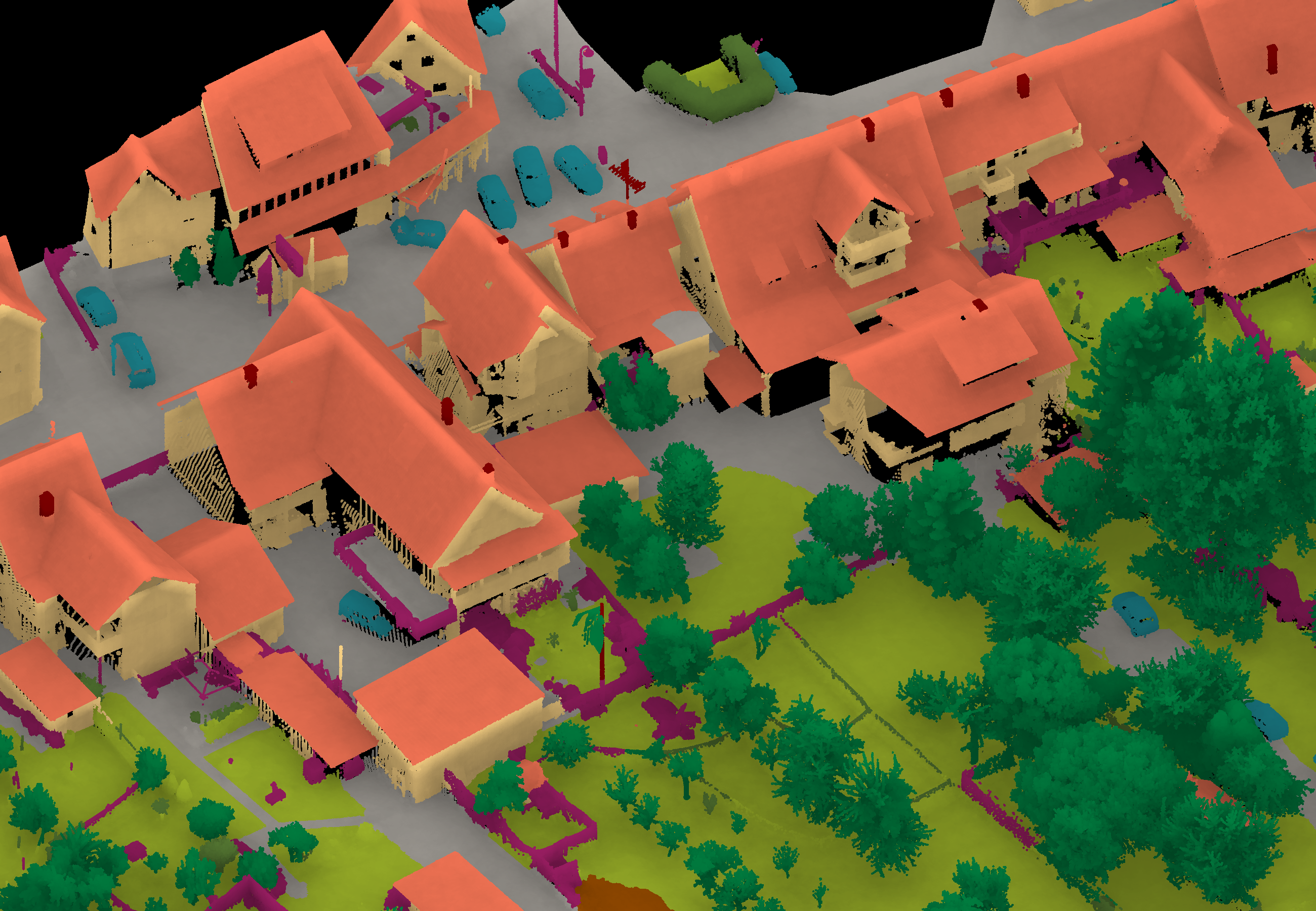
Results - Details for Zhang231204
Confusion Matrix
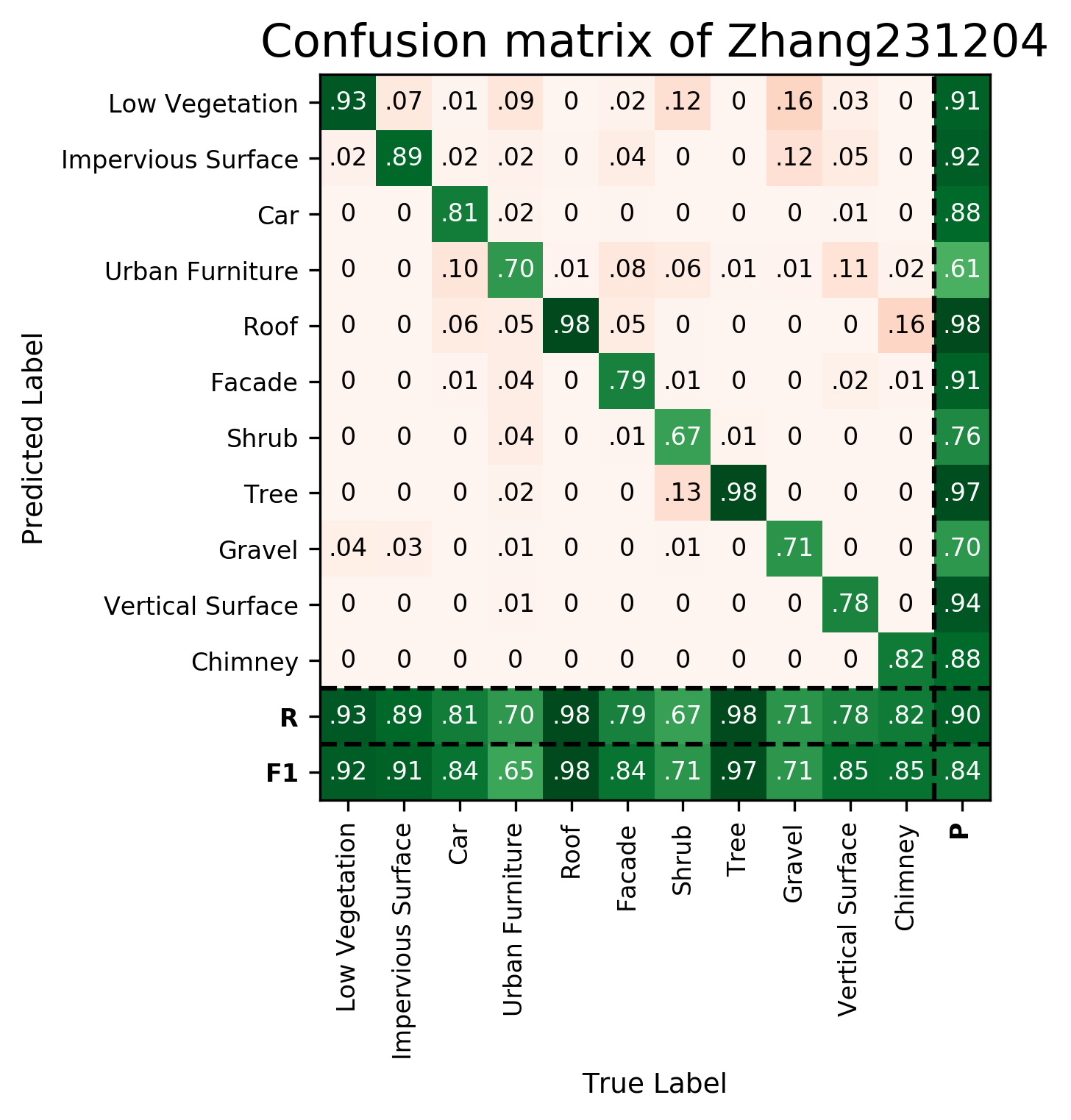
View 1
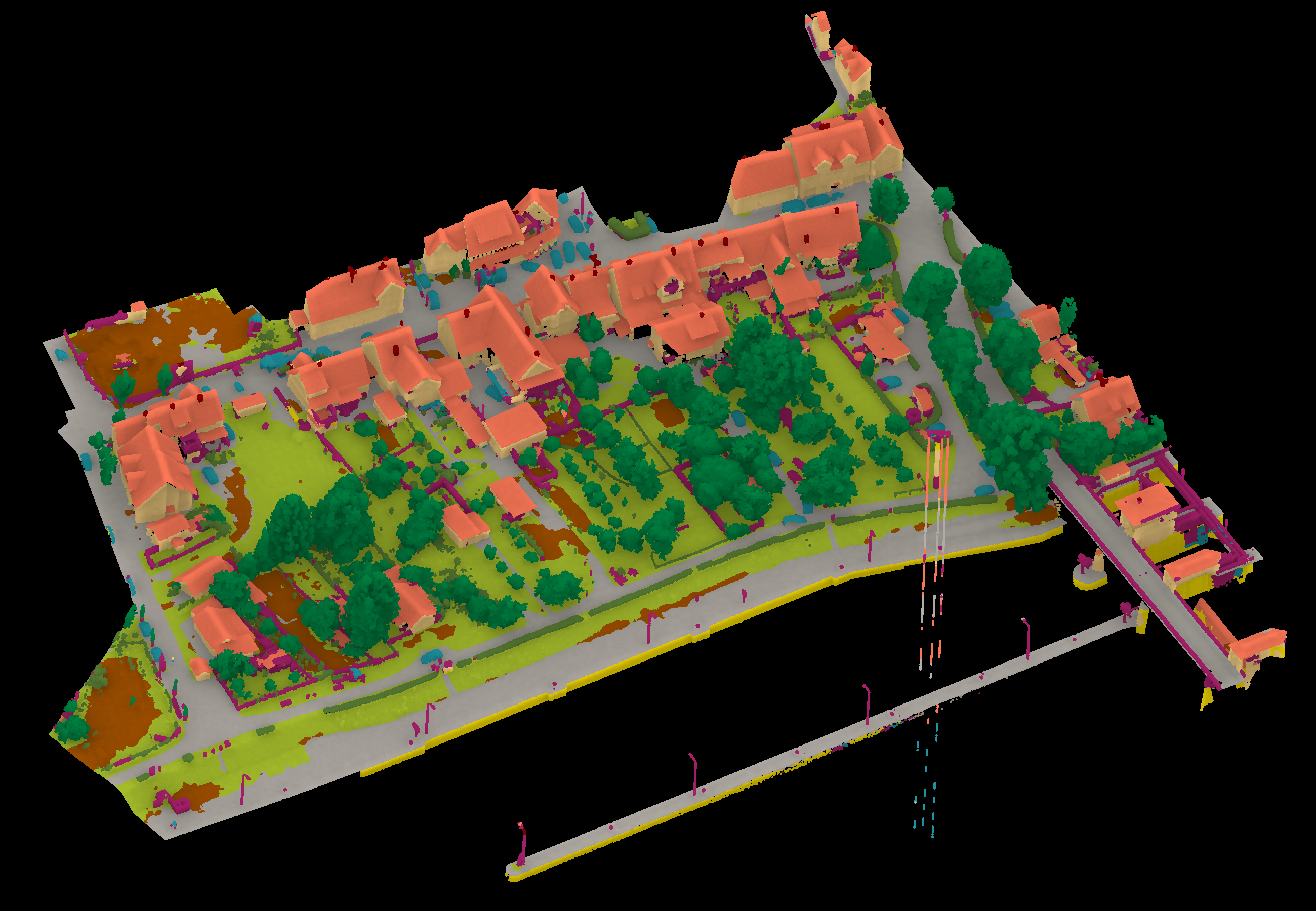
View 2
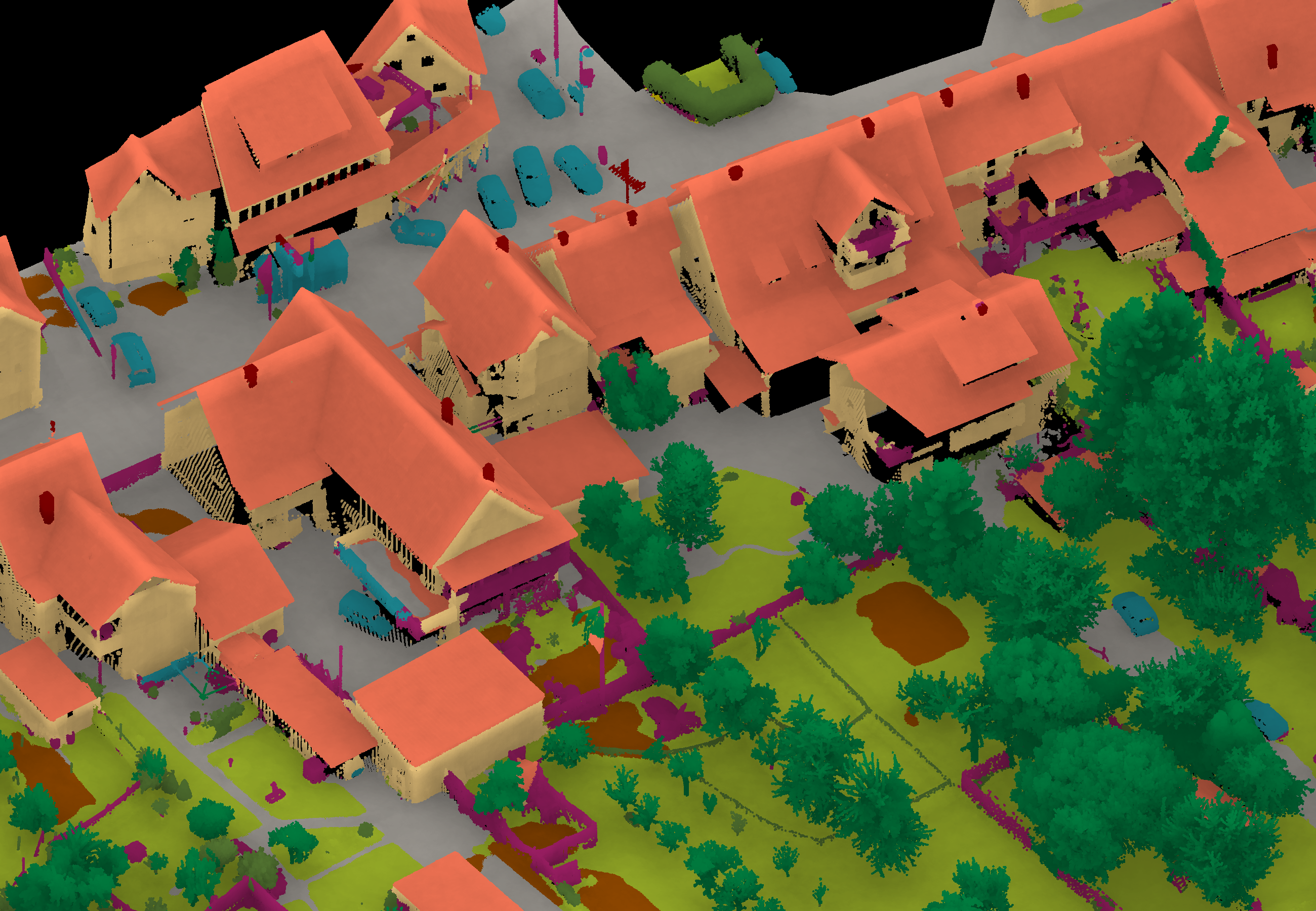
Details on the Approach of ifp-RF210223
Random Forest baseline approach as described in the official benchmark paper.
More information you will find here
Details on the Approach of ifp-SCN210223
Sparse Convolutional Network baseline approach as described in the official benchmark paper.
More information you will find here
Details on the Approach of Gao-KPConv210422
KPConv: Flexible and Deformable Convolution for Point Clouds
We use the Pytorch version of Kernel Point Convolution (KPConv) with class weights. We set the '0.1' as the first subsampling rate and 5.0 as the radius of the sphere. To avoid precision loss, we translate the coordinates to a local coordinate system. For other configurations, we follow the parameters as used in the dataset 'SemanticKitti'.
link to the code: https://github.com/HuguesTHOMAS/KPConv
More information you will find here
Details on the Approach of Gao-PN++210422
PointNet++
We use the implementation in Torch Points 3D. We add the data augmentation to reduce the problem of imbalanced classes, and we set the first subsampling rate as '0.1'. We choose the 'pointnet2_charlesmsg' as our architecture and we tune the radii to [[0.2, 0.4, 1.0], [1.0, 3.0]] to capture more contextual information.
More information you will find here
Details on the Approach of Sevgen220117
We used random sampling and radius search with k-max for multi-scale feature extraction, and random forest classifier for classification. Geometric, color, height and lidar features was used in feature extraction for total of 45 parameters for each level of samples without extra additional data. Random forest grid search was performed with training samples, the final model trained with added validation points, 25 000 training and 15 000 validation points for each class.
Details on the Approach of grilli220725
Introduction of knowledge-based rules within the last layer of a Point Transformer network, in order to improve the results in certain specific classes like vehicles, chimeys, and urban features.
More information you will find here
Details on the Approach of Sevgen220725
We are using multiscale feature extraction and gradiet boosting machine classifier.
More information you will find here
Details on the Approach of Shi220705
Our PIIE-DSA-net firstly introduce PIIE module to keep the low-level initial point cloud position and RGB information (optional), and combine them with deep features extracted by some kinds of state-of-the-art backbones. Secondly, we proposed DSA module, by using a learnable weights transformation tensor to transform the combined features and following a self-attention structure to generate better features for 3D segmentation.
More information you will find here
Details on the Approach of Zhan221025
Pt-Deeplab is a novel approach which we will pulish soon
Details on the Approach of Zhan221112
Pt-deeplab is a novel approach, which we will pulish soon
Details on the Approach of jiabin221114
We propose a point convolution network framework based on spatial location correspondence. Following the correspondence principle can guide us to design convolution networks adapted to our needs. We analyzed the intrinsic mathematical nature of the convolution operation and argue that the convolution operation remains the same when the spatial location correspondence between the convolution kernel points and the convolution range elements remain unchanged. We trained the model only using xyz without color
Details on the Approach of WHU221118
To test our novel approach, pt-deeplab
Details on the Approach of Esmoris230207
We are working on a paper analyzing VLS data for deep learning. One-third of our data comes from the Hessigheim dataset. We would like to submit two versions of the same model, one using real data (KPCSO) and the other using virtual data (VLS-KPCSO). Our analysis uses metrics and figures calculated on the validation datasets. For better scientific practices, we would like to measure how close the validation data scores are to test data for both cases. We will send a link to the paper after publication.
More information you will find here
Details on the Approach of Esmoris230208
As commented in the previous upload, we are now uploading the VLS version corresponding to our previous real model. We know the benchmark rules allow one upload per model. However, we argue that training a similar model twice, one using the real point clouds and one using virtual point clouds (generated using HELIOS++ and given meshes), is a different enough contribution. We noted an 0.0892 accuracy reduction when using VLS data wrt to real data. We'd like to verify the virtual-to-real generalization against the test data for compliance with high-quality scientific practices.
More information you will find here
Details on the Approach of Letard230213
we introduce explainable machine learning for 3D data classification using Multiple Attributes, Scales, and Clouds under 3DMASC, a new plugin in CloudCompare. Thanks to original dual-cloud and statistics-augmented 3D attributes, it offers new possibilities for point cloud classification, particularly for automatic interpretation of topo-bathymetric lidar data, still lacking operational methods. Experimental results on topo-bathymetric lidar data show that 3DMASC competes with state-of-the-art methods in terms of classification performances while demanding lower computation complexity and remaining accessible to non-specialist users.Our contributions consist in:
• Designing new joint-cloud features calculated on two PCs using their local geometry and backscattered intensity. 3DMASC uses a flexible method to compute features from two PCs,
potentially resulting in more than 80 different features;
• Screening systematically over 80 features, both classical and new, to select the essential features and scales contributing to 3D semantic point classification success to develop optimal classifiers in terms of computational efficiency, generalization capability, and interpretability;
• Demonstrating that with limited training data (< 2000 points per class) and less than ten features and five scales, the classification accuracy of TB lidar datasets can be excellent (>0.95);
• Providing a standalone plugin in the open source software Cloudcompare (CC) implementing 3DMASC that non-specialists can use for generic training and classification of any 3D PC (Airborne lidar, Terrestrial lidar, Photogrammetric PCs) and by experts for fast 3D feature computations on PCs.
We decided to evaluate 3DMASC on the H3D dataset to assess its generalization and performances on urban environments.
Details on the Approach of WHU230322
We investigate a semantic segmentation model for small target objects in urban point clouds. We will publish it soon
Details on the Approach of Qiu230831
A method for point cloud semantic segmentation based on local position encoding and attention has been extended to process typical remote sensing features. Furthermore, post-processing using CRFs has been applied.
Details on the Approach of Zhang231204
To address the class imbalance in large scenes, we propose a target-guided model for rare class segmentation.
| F1-scores in % | |
---|
Participant | A | C00 | C01 | C02 | C03 | C04 | C05 | C06 | C07 | C08 | C09 | C10 | mF1 | OA |
---|
ifp-RF-210223 | ➥ | 89.35 | 89.06 | 61.38 | 57.65 | 93.29 | 82.16 | 69.27 | 96.09 | 48.85 | 62.58 | 76.43 | 75.10 | 86.53 |
ifp-SCN-210223 | ➥ | 89.82 | 83.66 | 61.05 | 52.24 | 87.09 | 81.01 | 59.75 | 95.06 | 51.00 | 56.61 | 70.22 | 71.59 | 83.73 |
Tang-210406 | ➥ | 87.79 | 86.74 | 41.43 | 49.10 | 88.22 | 75.41 | 57.45 | 91.05 | 53.91 | 78.31 | 69.30 | 70.79 | 83.26 |
GAO-KPConv-220330 | ➥ | 64.47 | 64.45 | 66.02 | 47.29 | 89.97 | 71.26 | 56.95 | 91.41 | 12.56 | 58.83 | 27.22 | 59.13 | 70.65 |
GAO-SPG-220330 | ➥ | 54.44 | 43.04 | 5.60 | 33.08 | 74.86 | 75.30 | 0.08 | 82.39 | 20.99 | 56.14 | 0.00 | 40.54 | 56.52 |
GAO-PSSNet-220330 | ➥ | 84.88 | 86.37 | 49.19 | 39.47 | 88.37 | 72.34 | 41.31 | 89.90 | 40.94 | 70.86 | 60.54 | 65.83 | 79.25 |
GAO-PN++-220330 | ➥ | 57.54 | 70.67 | 0.00 | 2.14 | 37.53 | 36.62 | 0.31 | 43.32 | 0.00 | 3.42 | 0.00 | 22.87 | 46.83 |
Schreiber230926 | ➥ | 89.64 | 86.18 | 63.47 | 55.27 | 90.16 | 85.06 | 60.83 | 94.31 | 40.09 | 78.86 | 78.65 | 74.77 | 85.38 |
Results - Details for ifp-RF-210223
Confusion Matrix
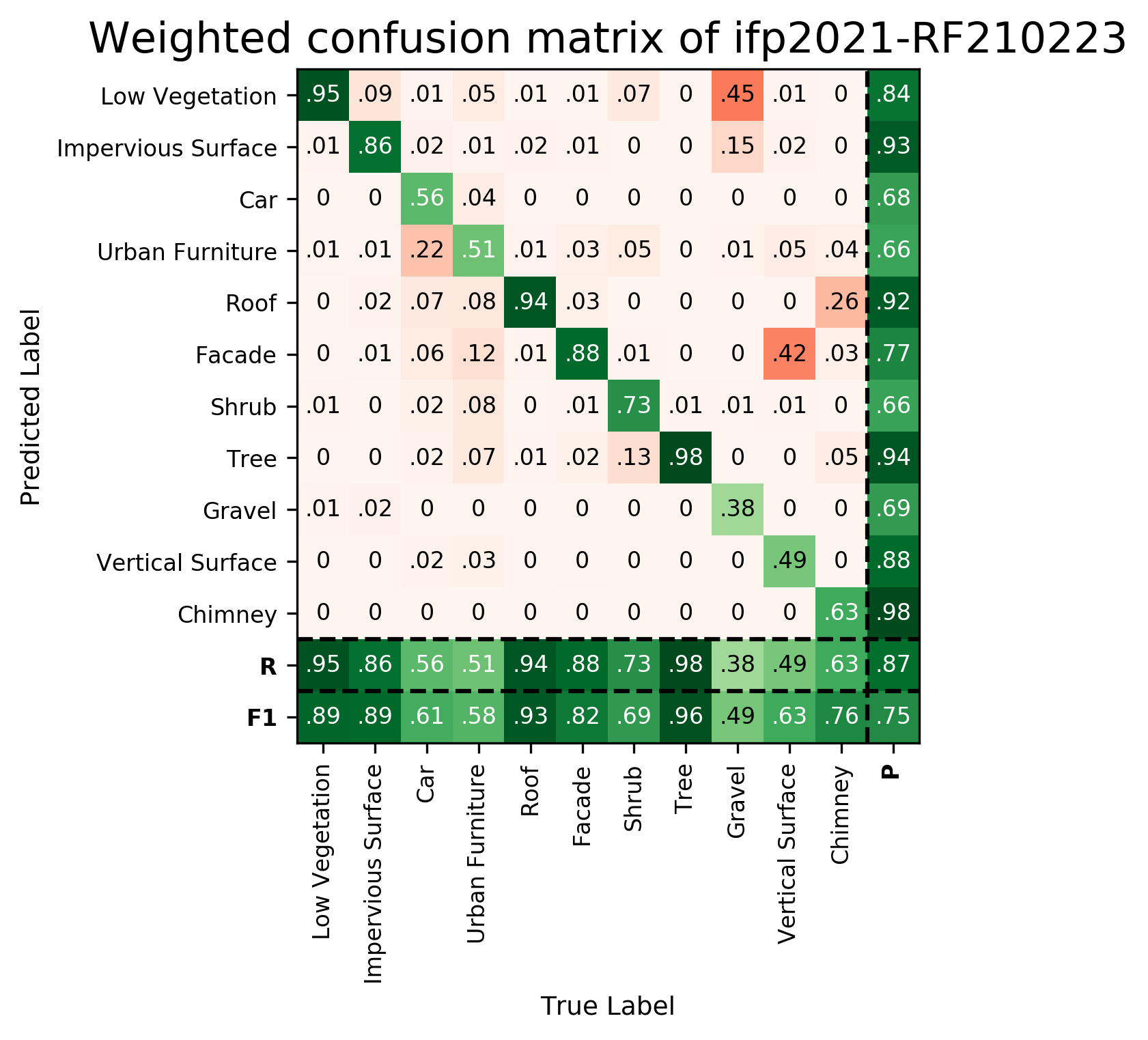
View 1
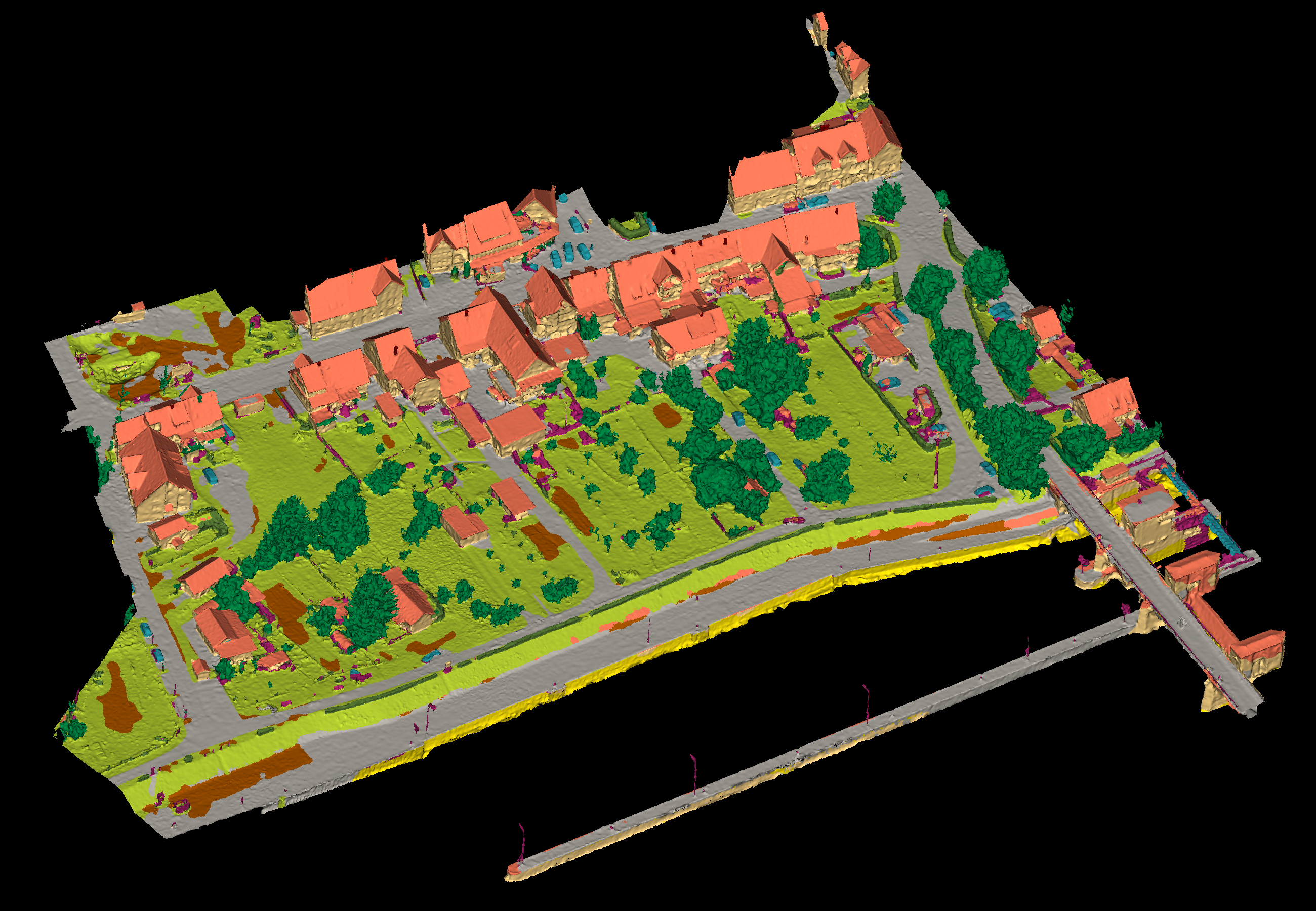
View 2
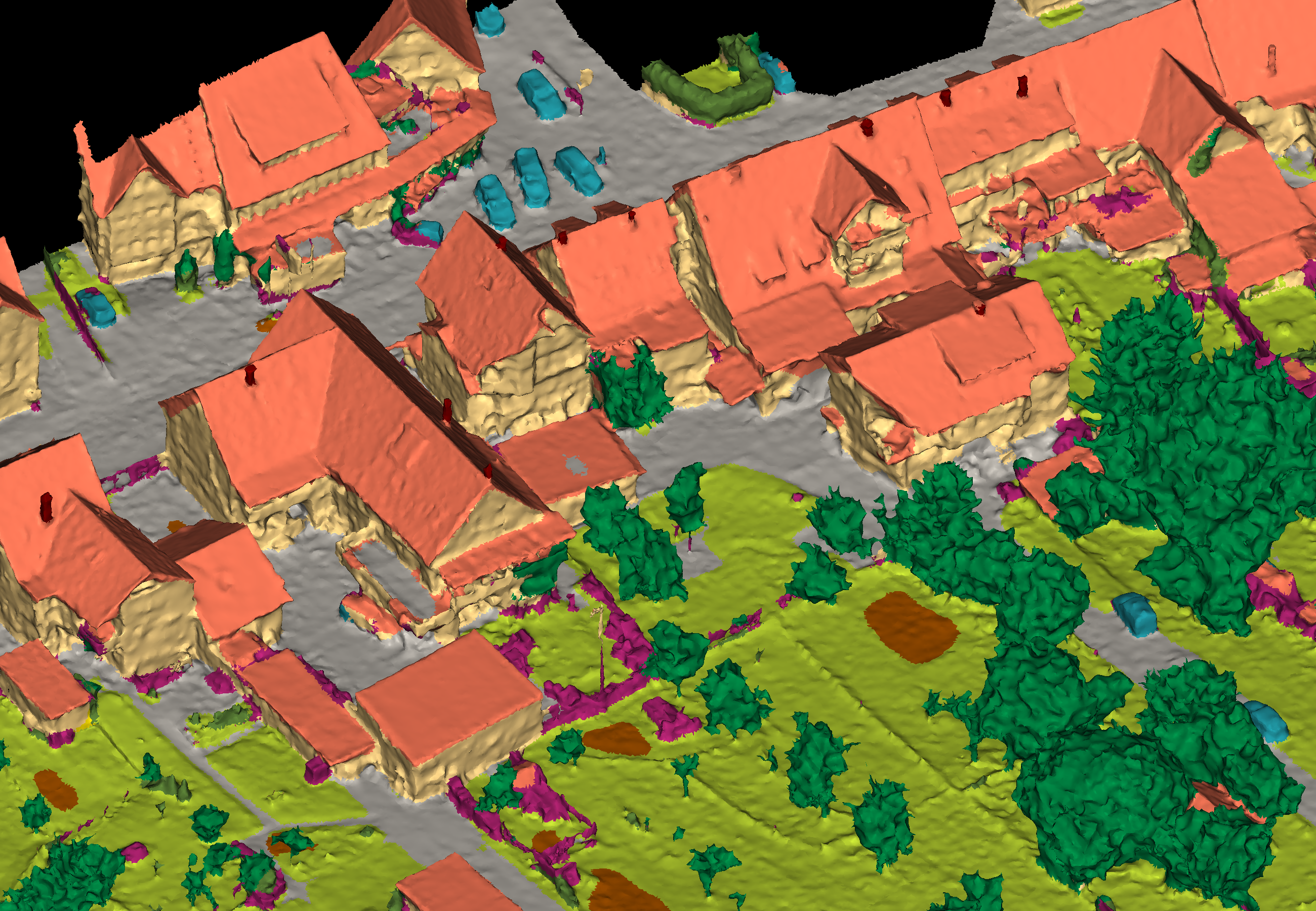
Results - Details for ifp-SCN-210223
Confusion Matrix
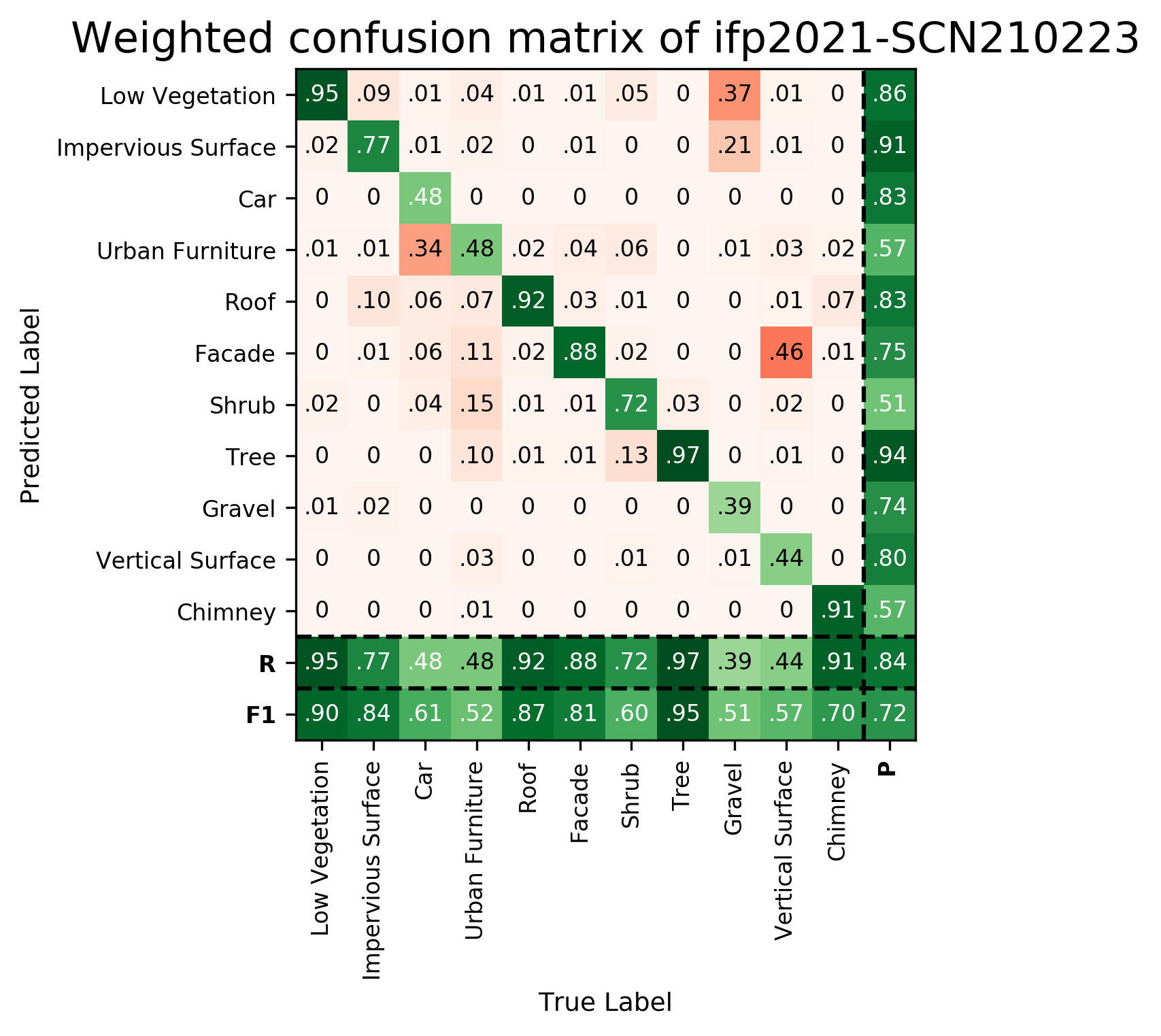
View 1
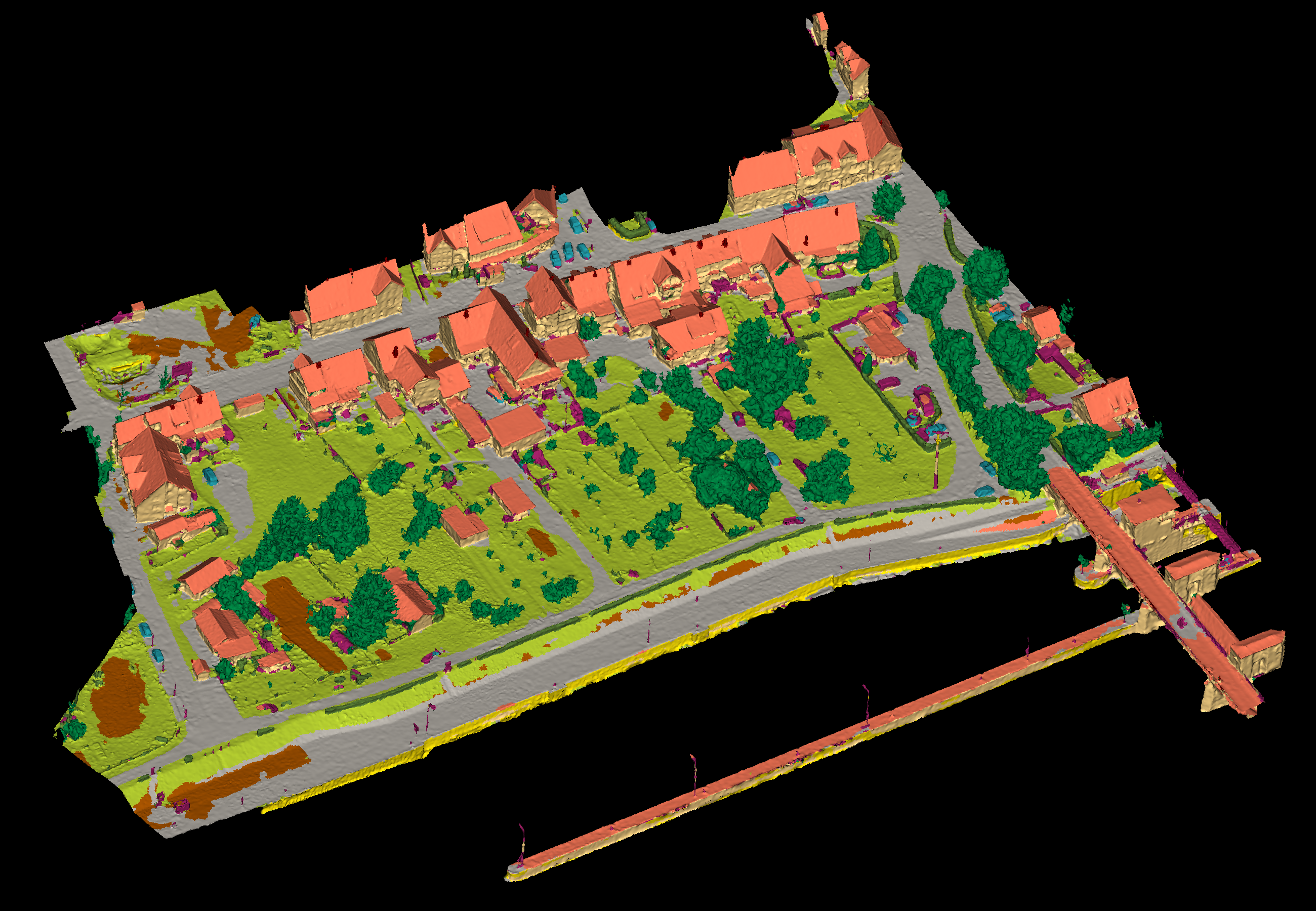
View 2
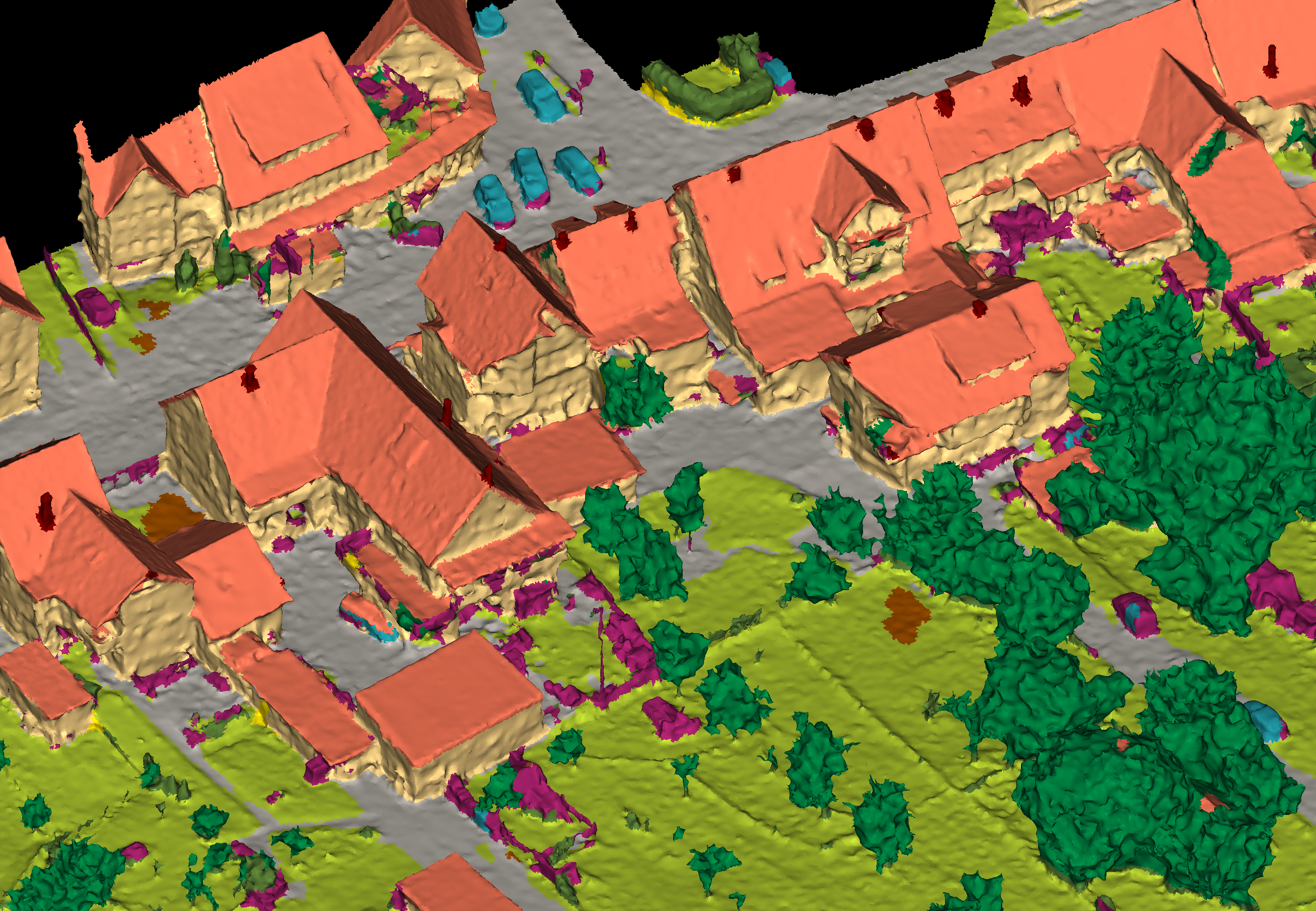
Results - Details for Tang-210406
Confusion Matrix
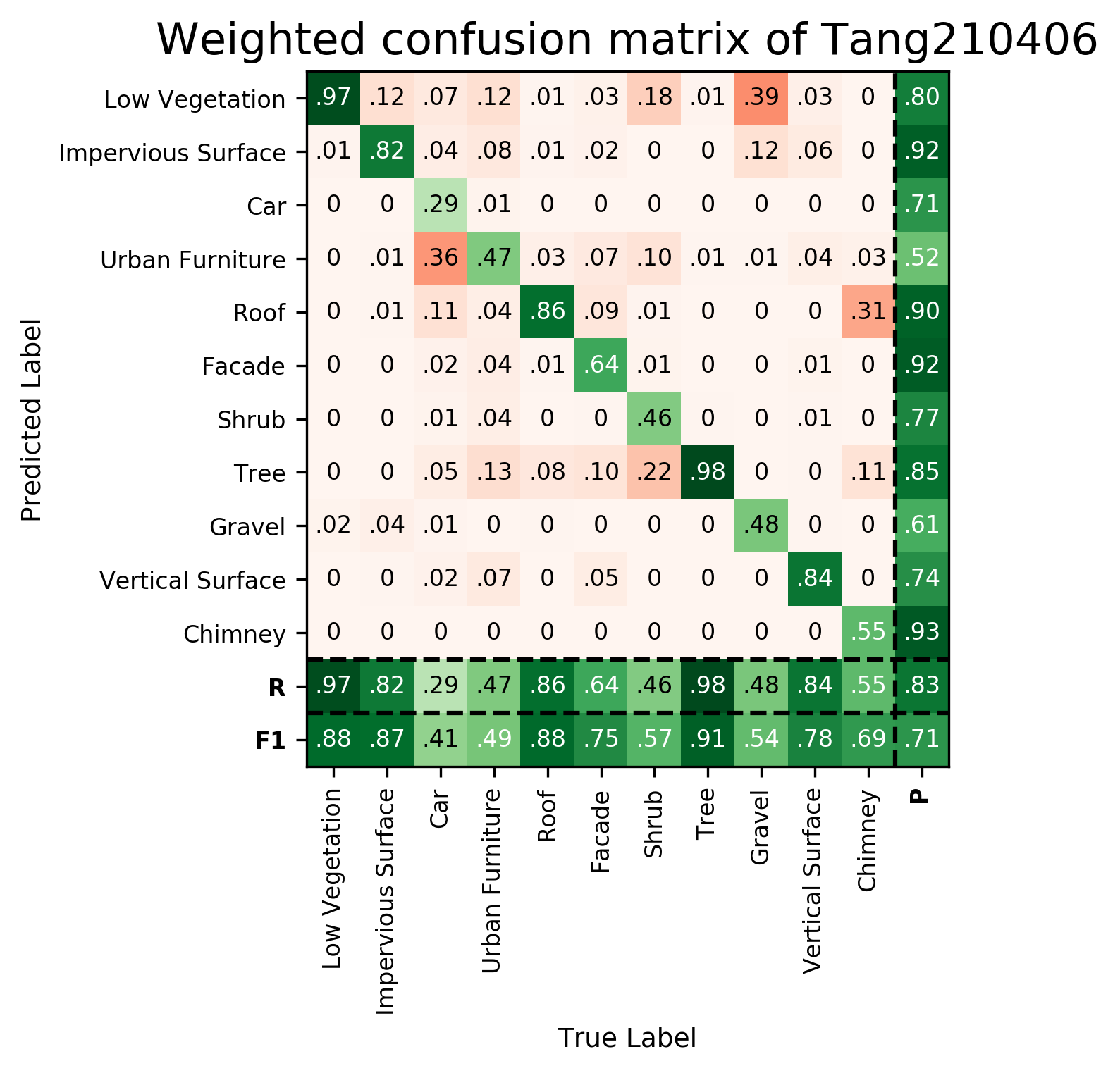
View 1
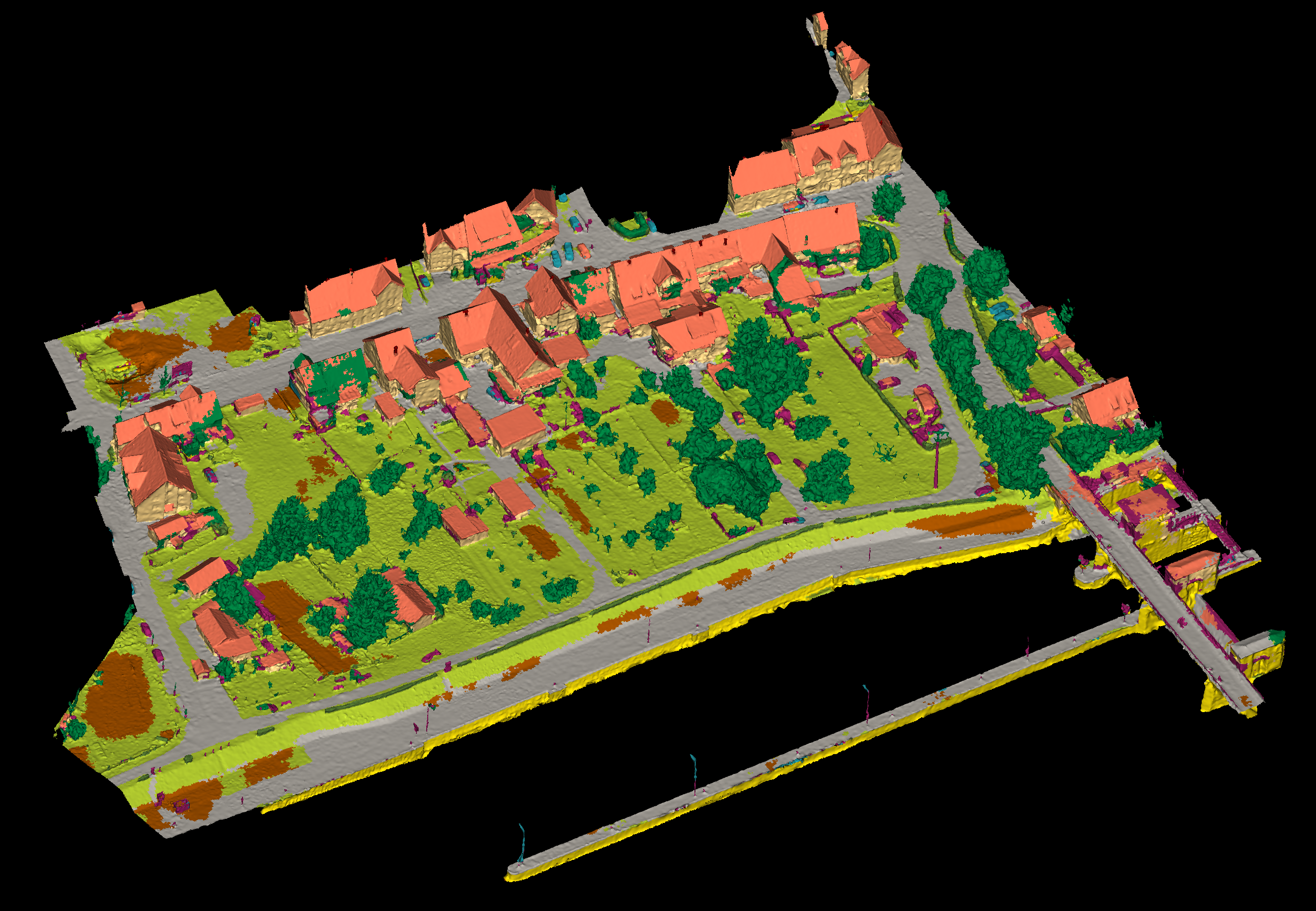
View 2
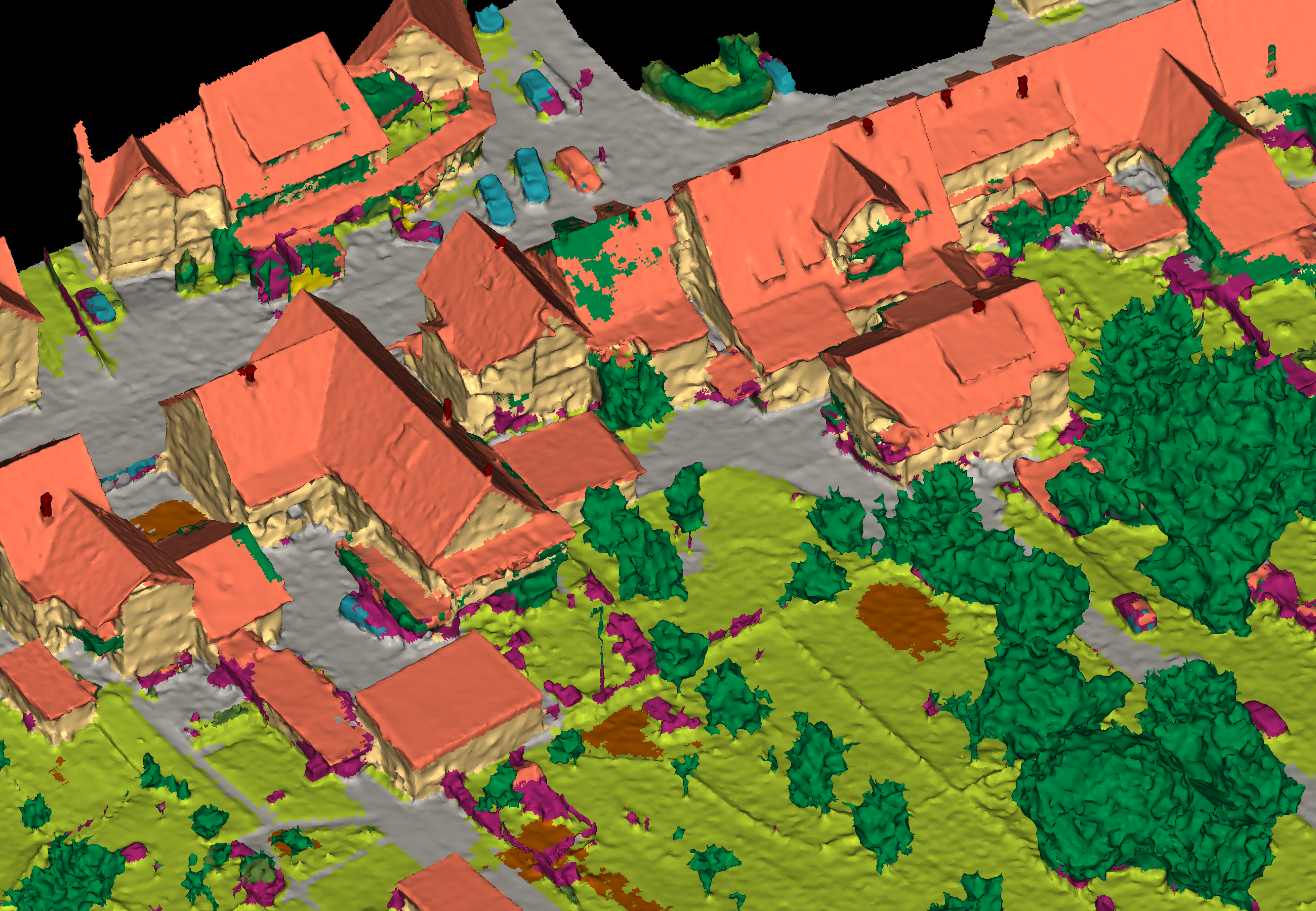
Results - Details for GAO-KPConv-220330
Confusion Matrix
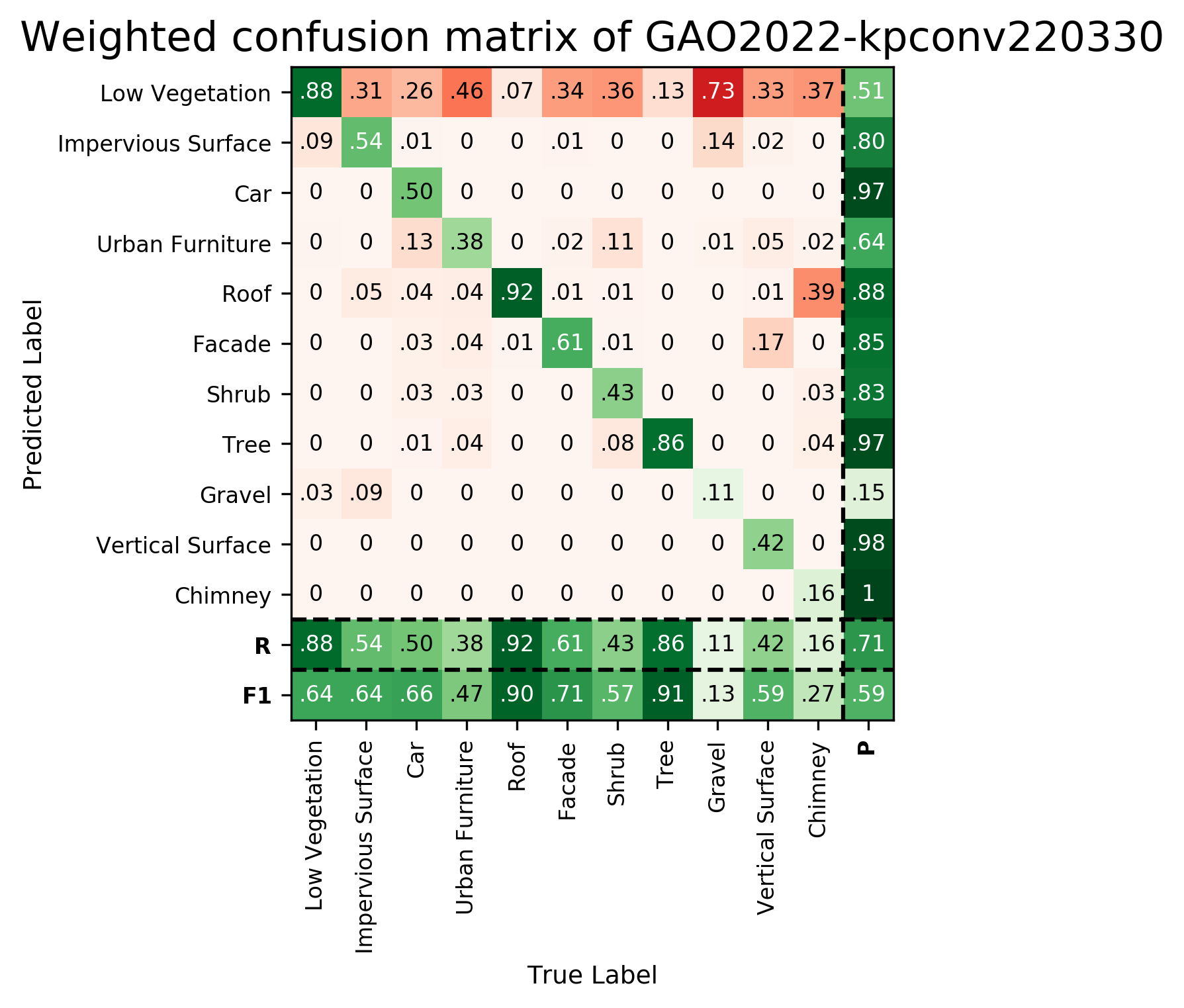
View 1
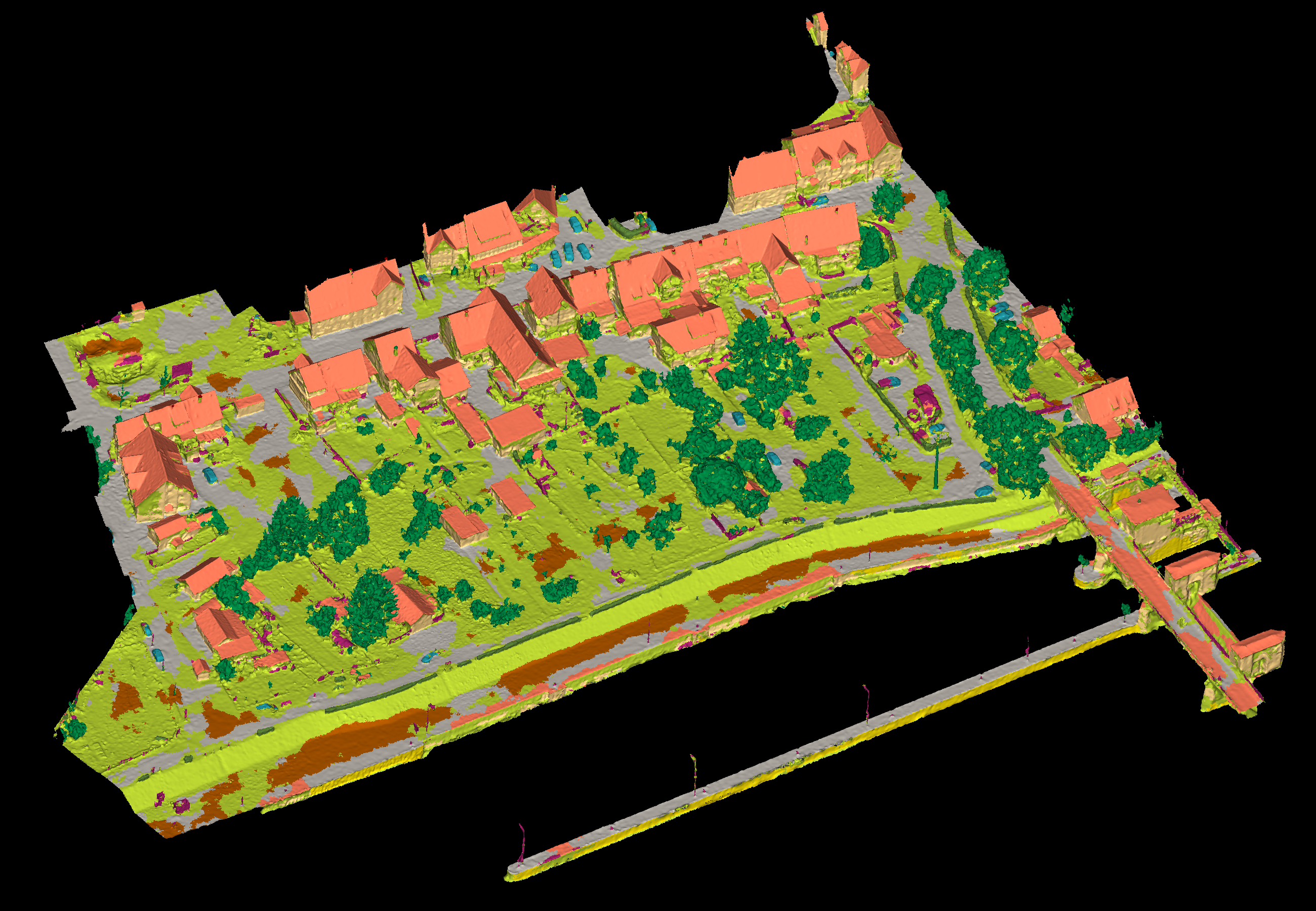
View 2
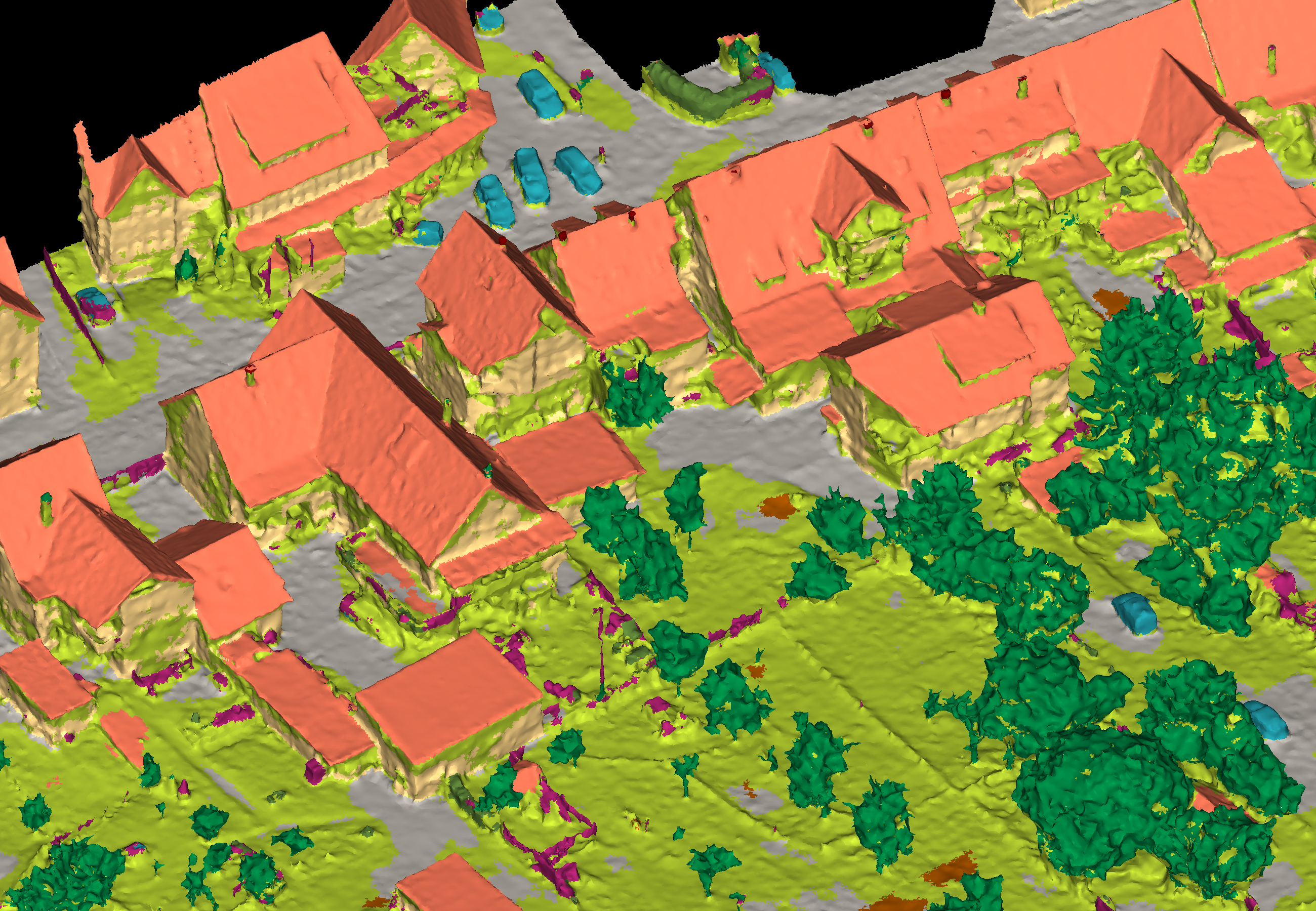
Results - Details for GAO-SPG-220330
Confusion Matrix
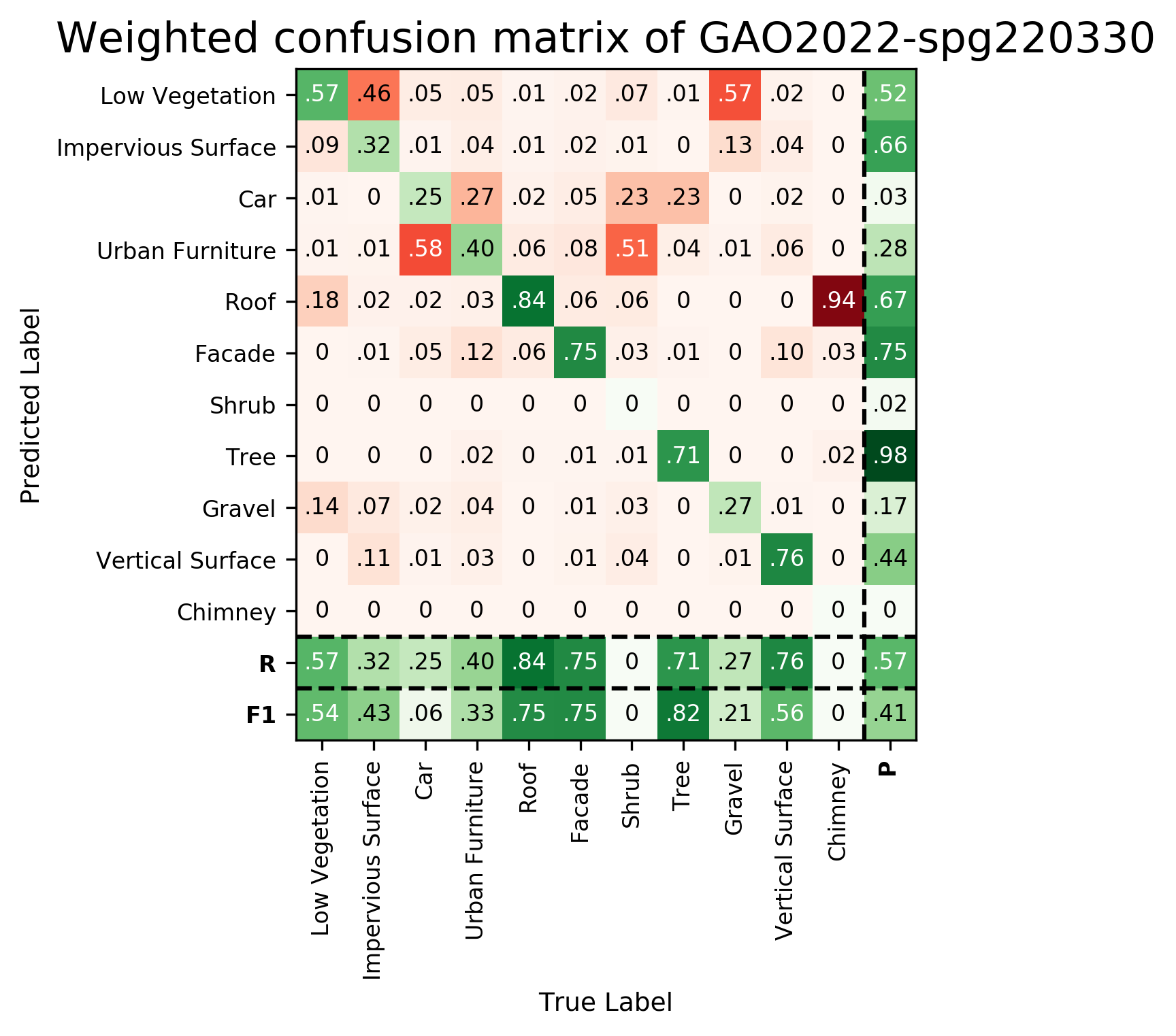
View 1
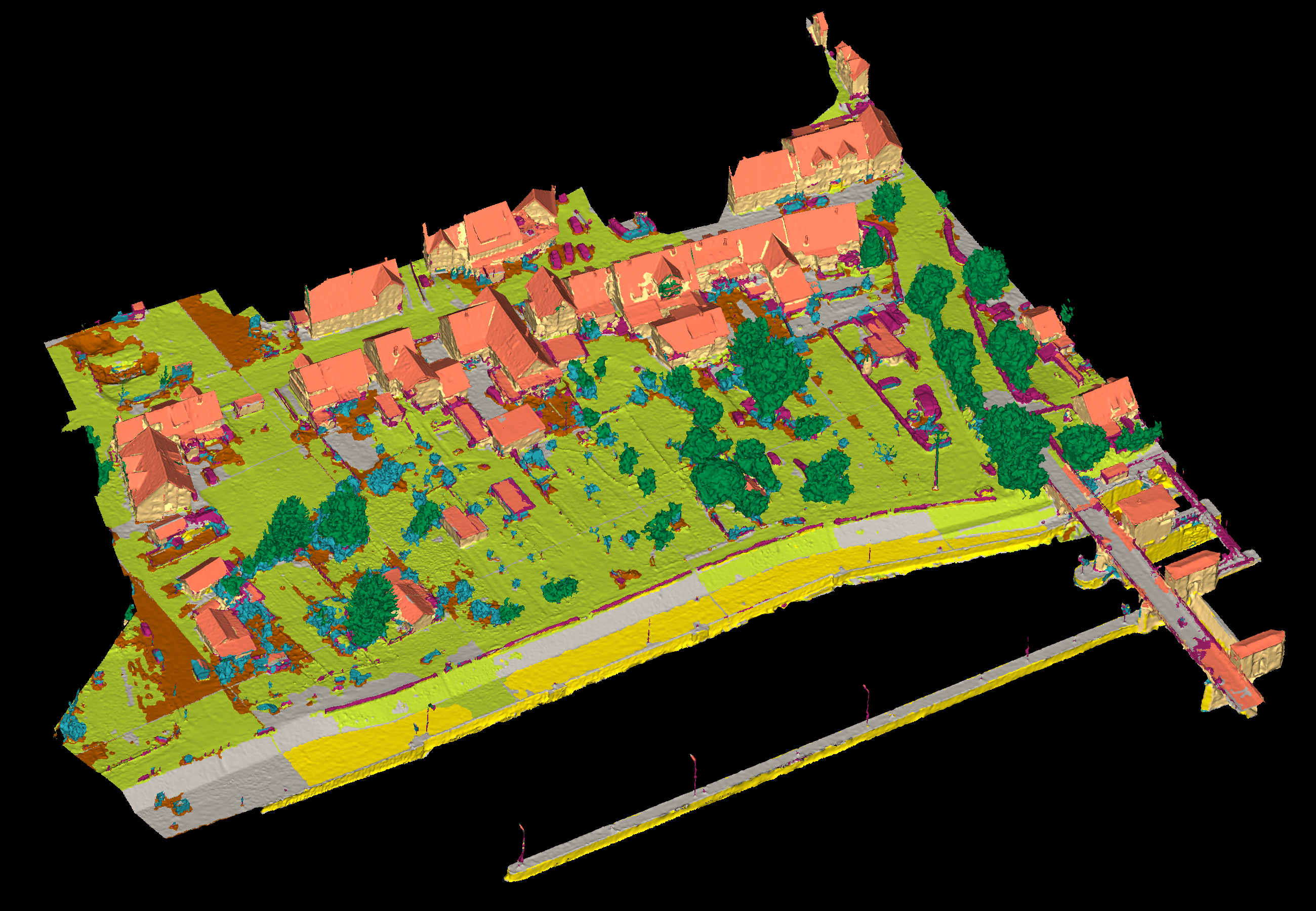
View 2
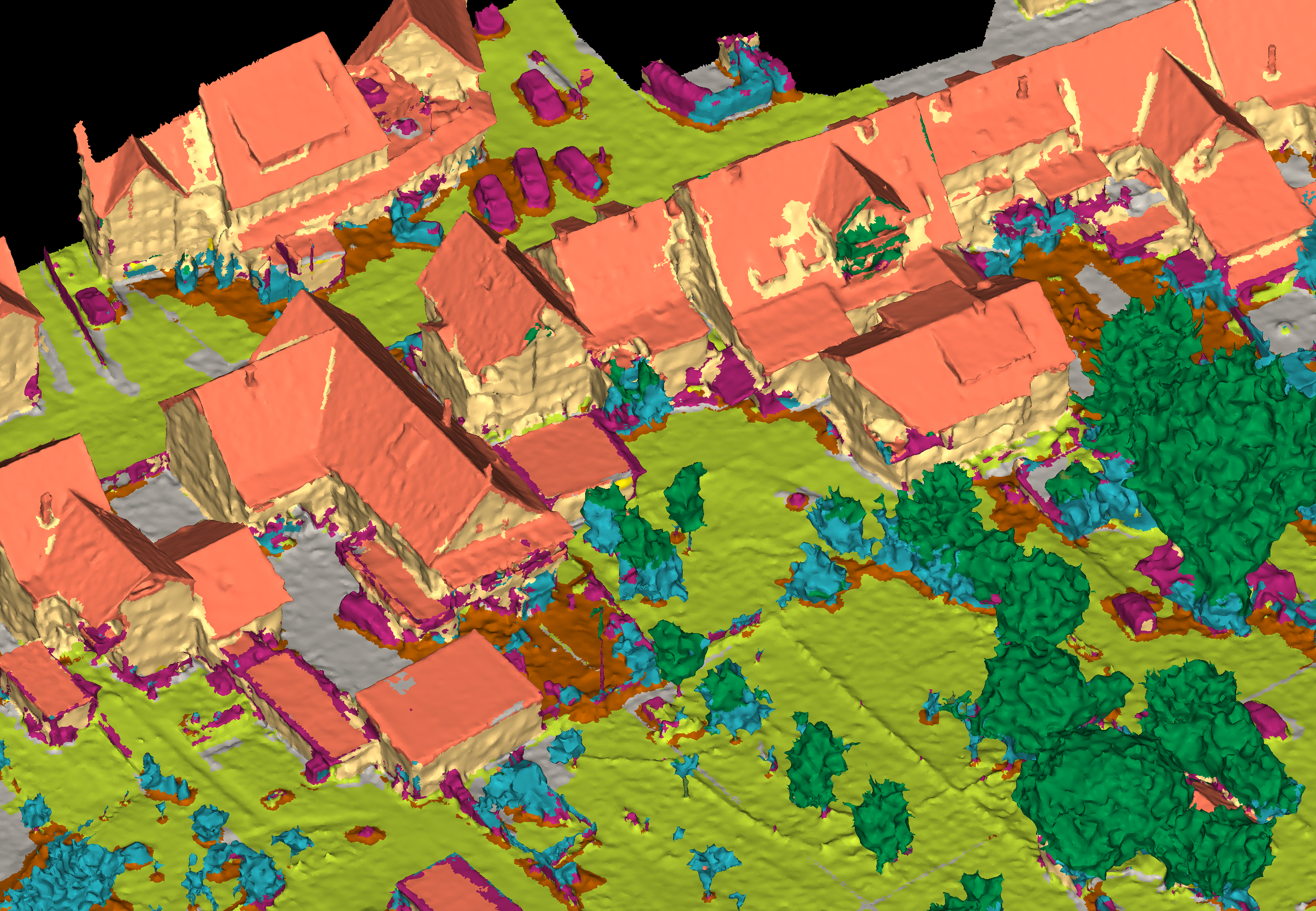
Results - Details for GAO-PSSNet-220330
Confusion Matrix
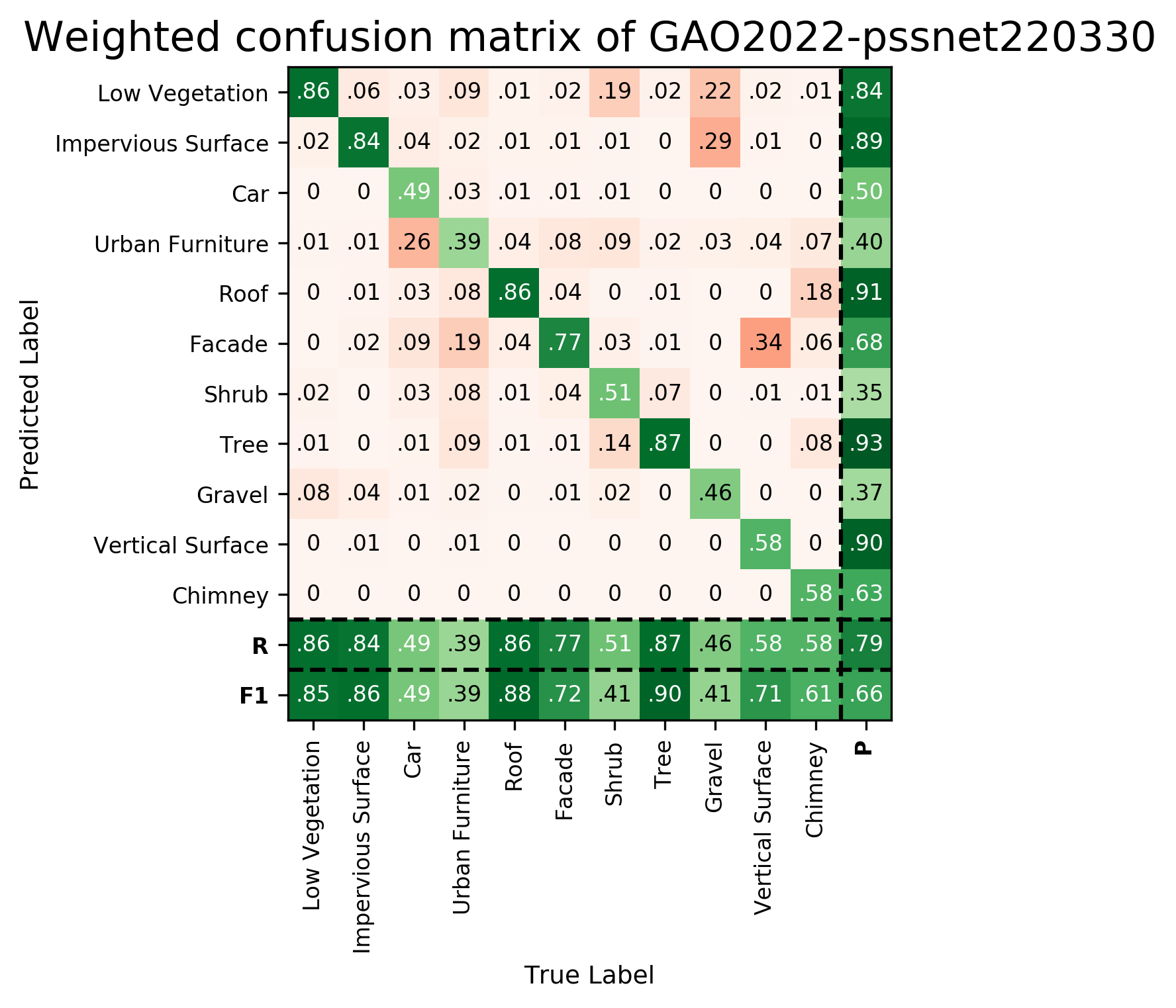
View 1
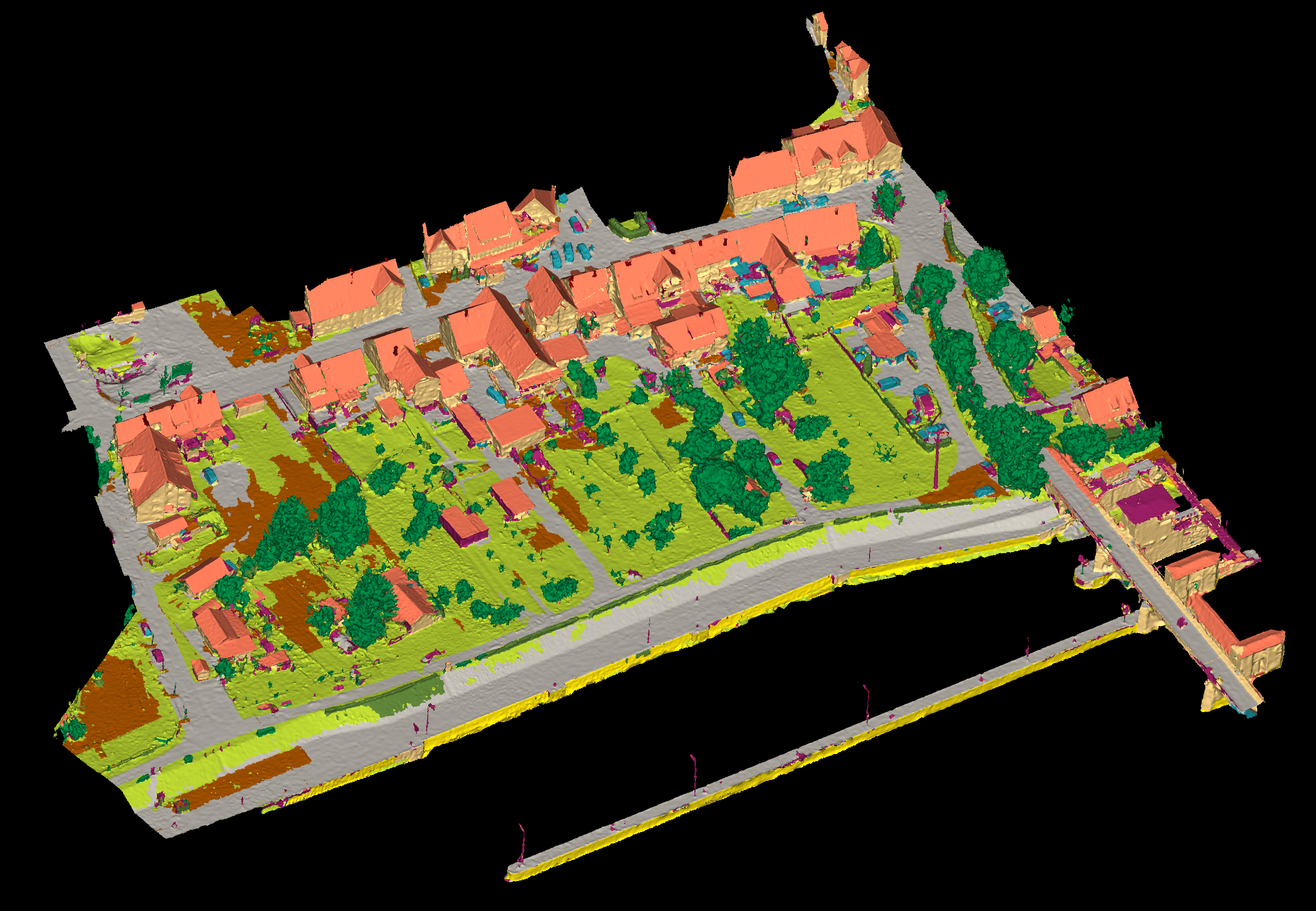
View 2
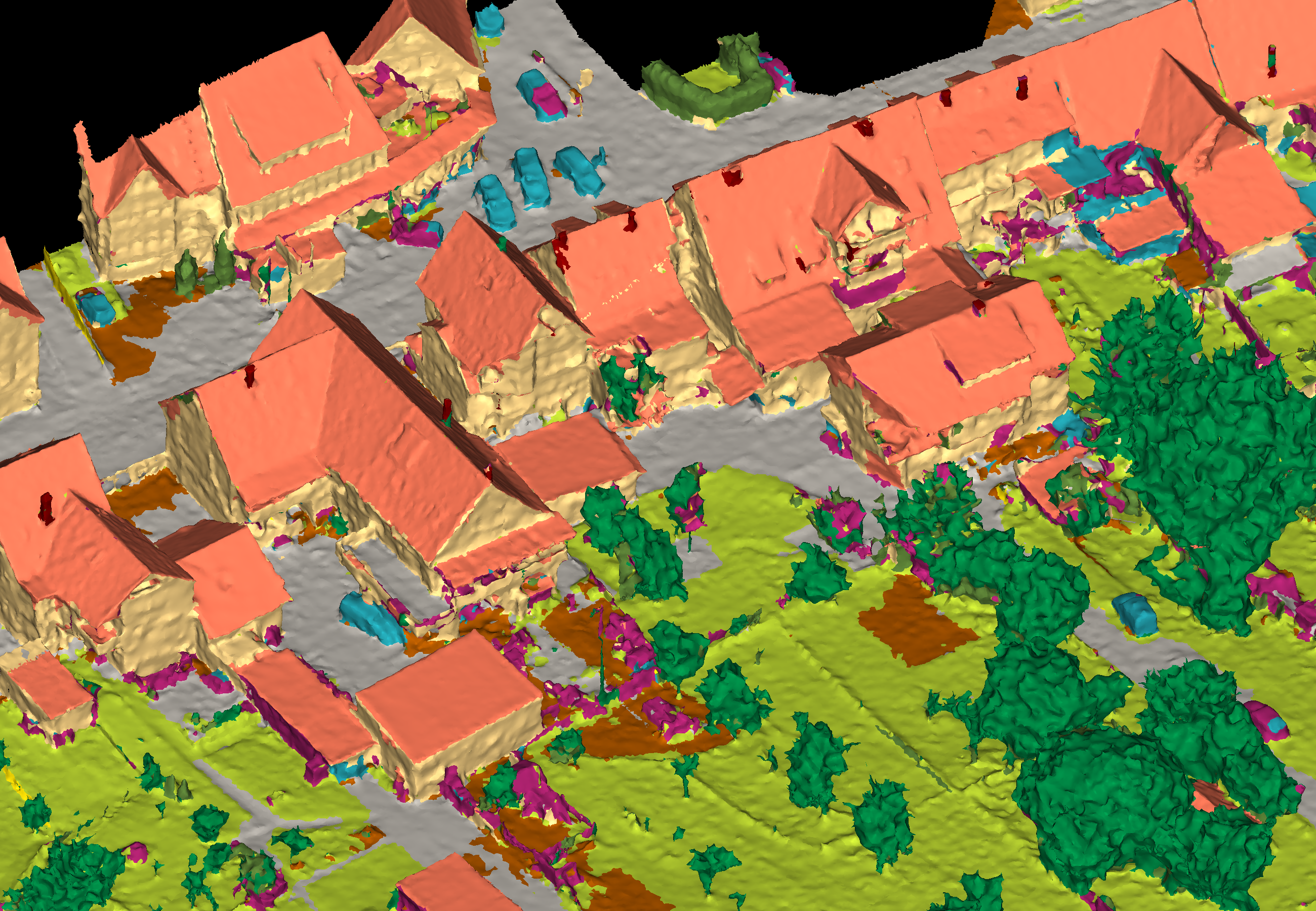
Results - Details for GAO-PN++-220330
Confusion Matrix
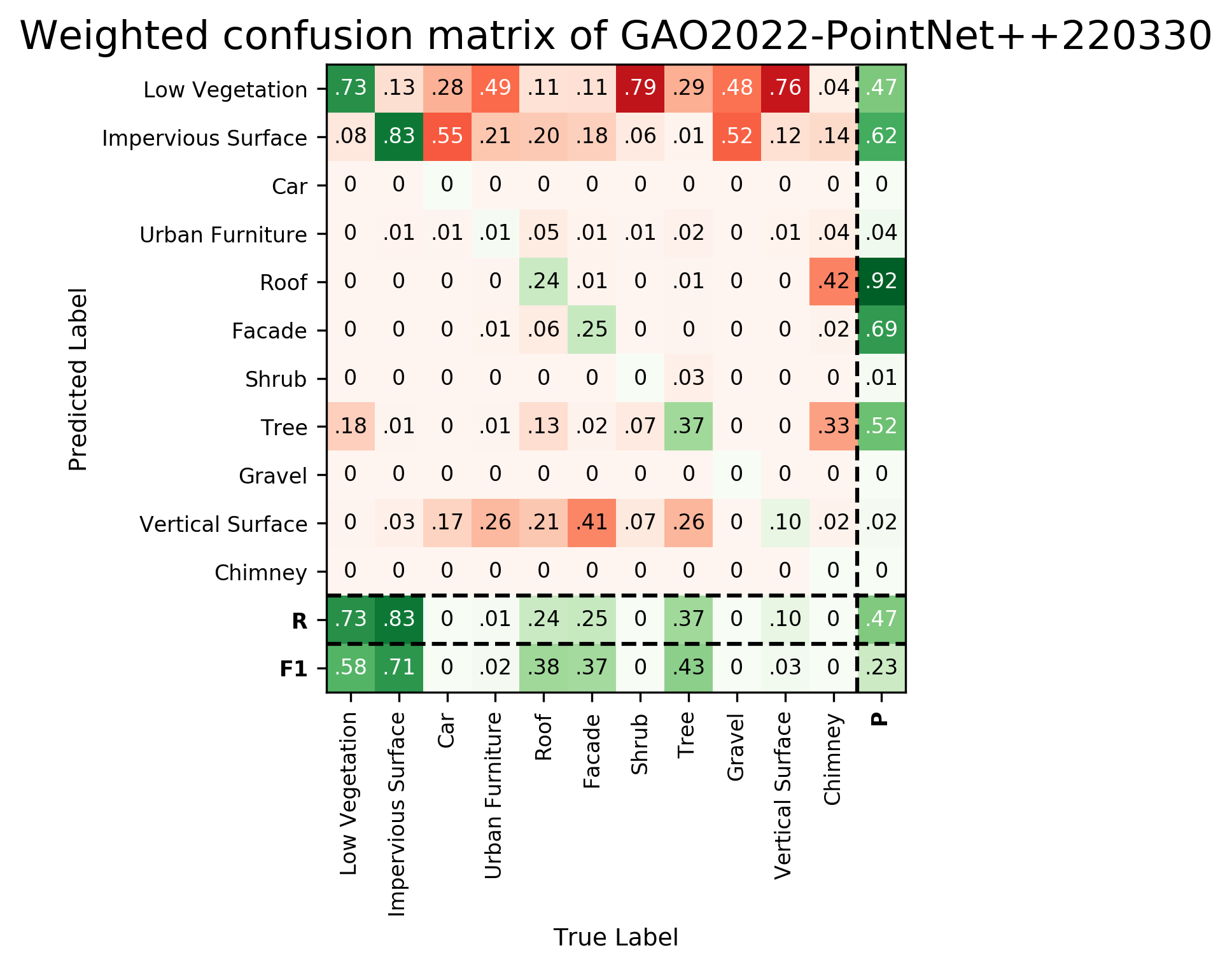
View 1
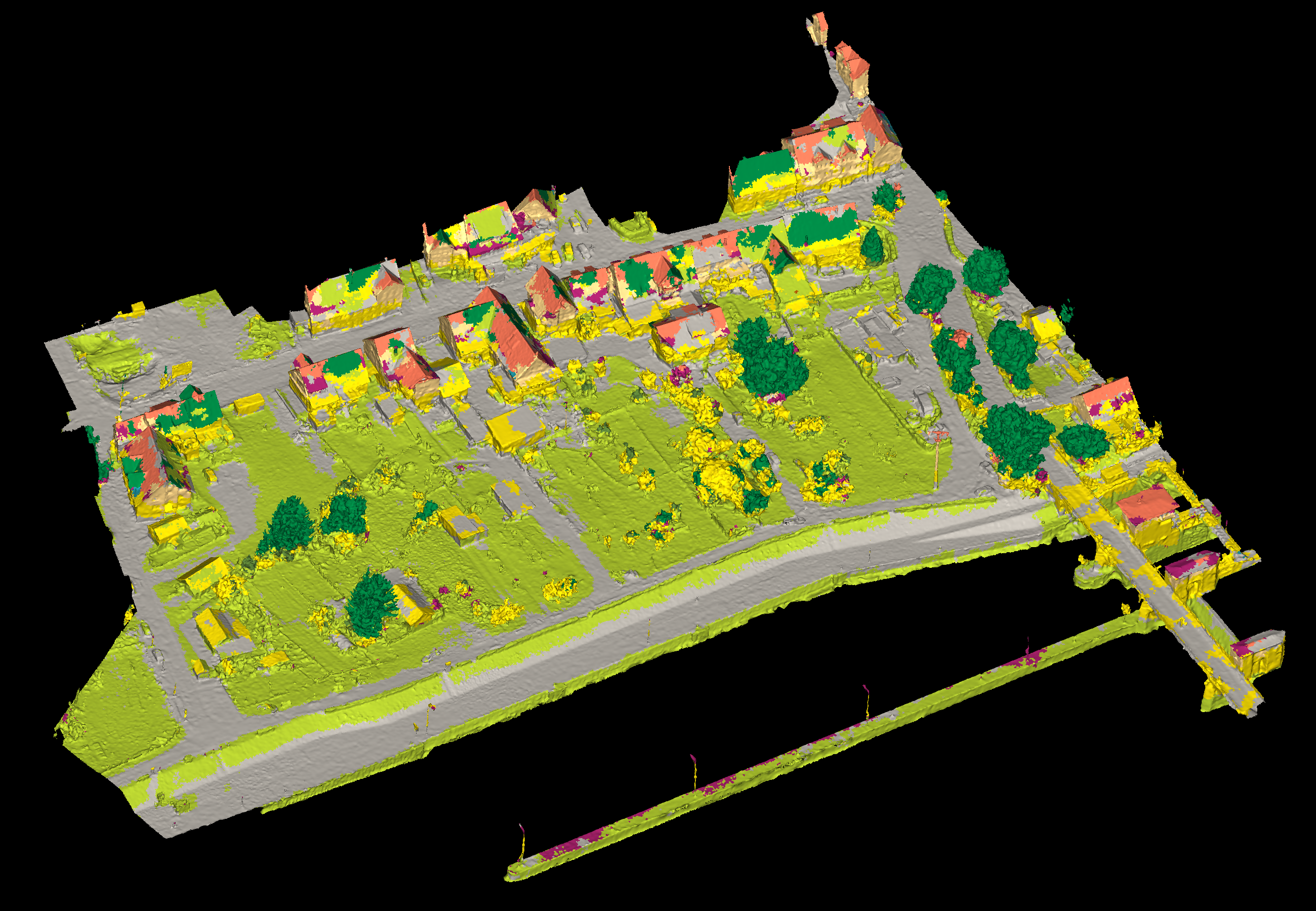
View 2
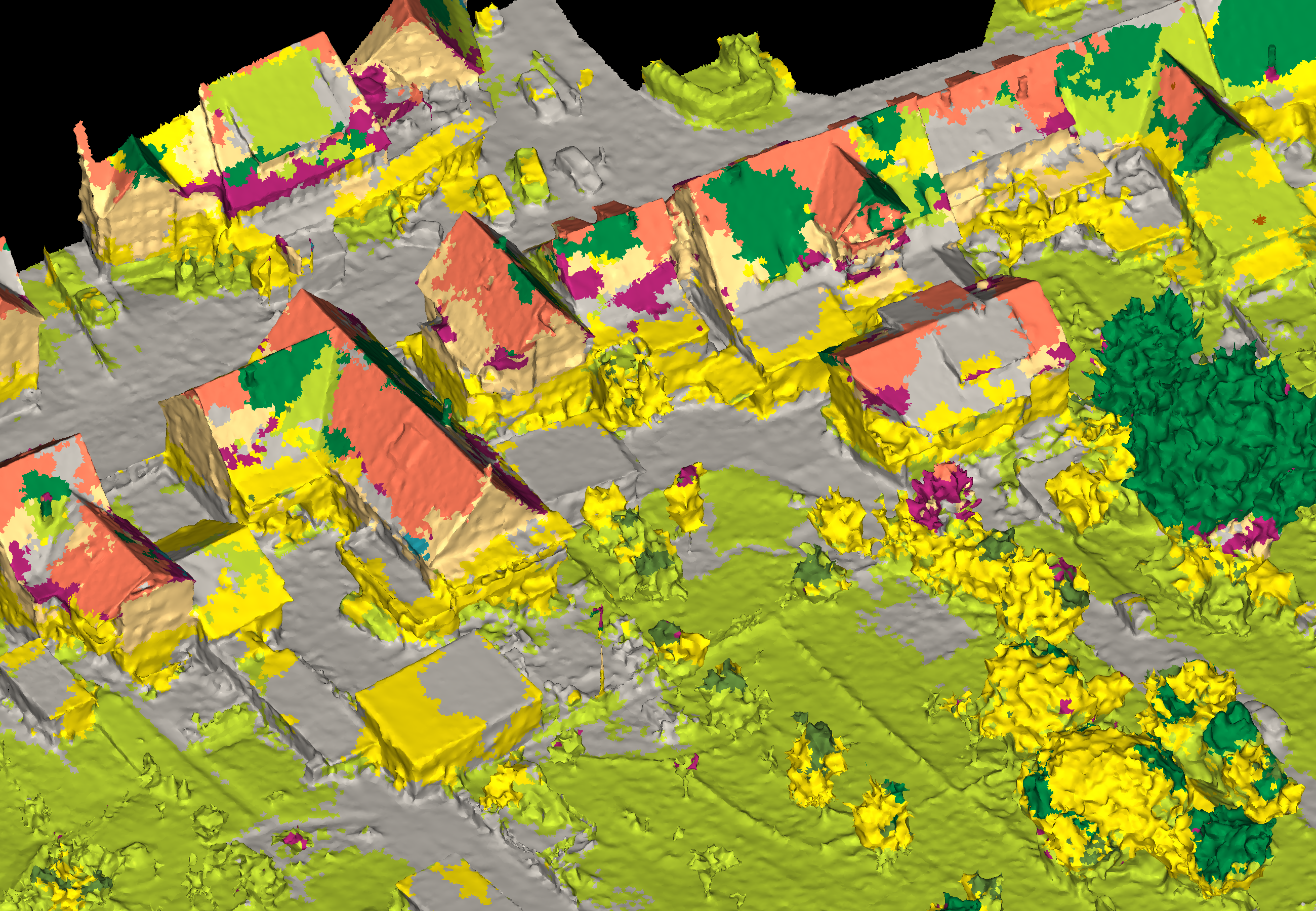
Results - Details for Schreiber230926
Confusion Matrix
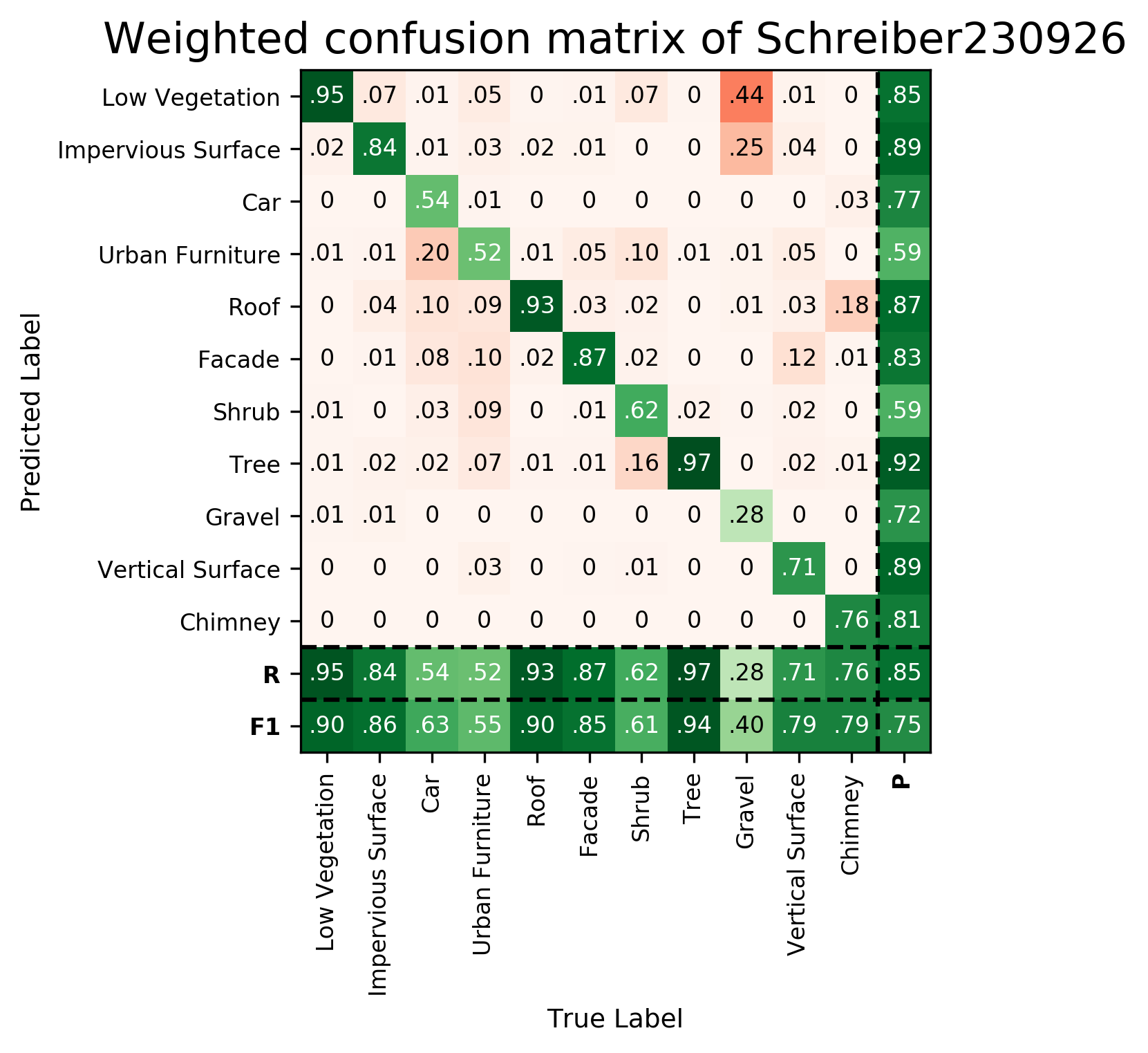
View 1
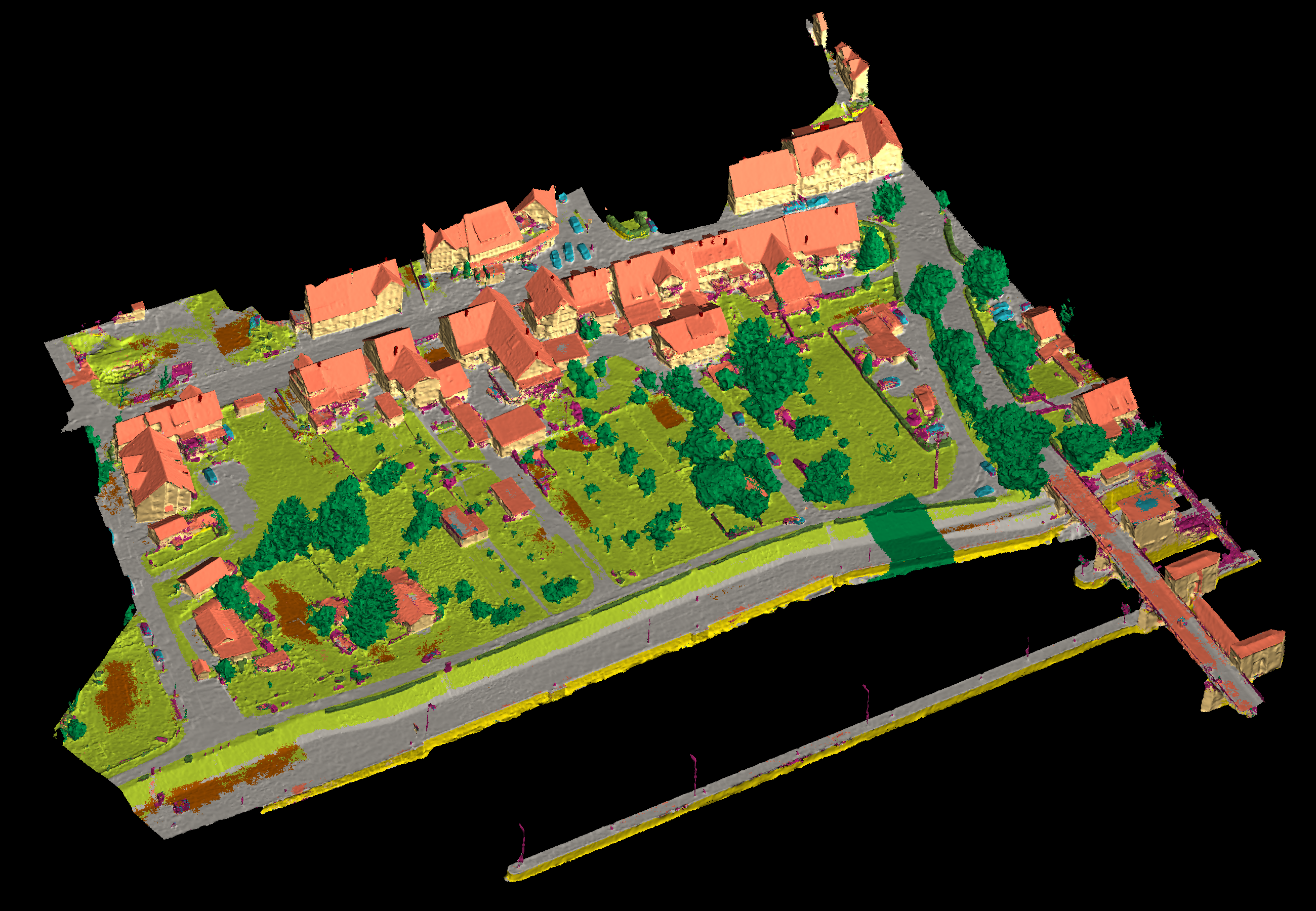
View 2
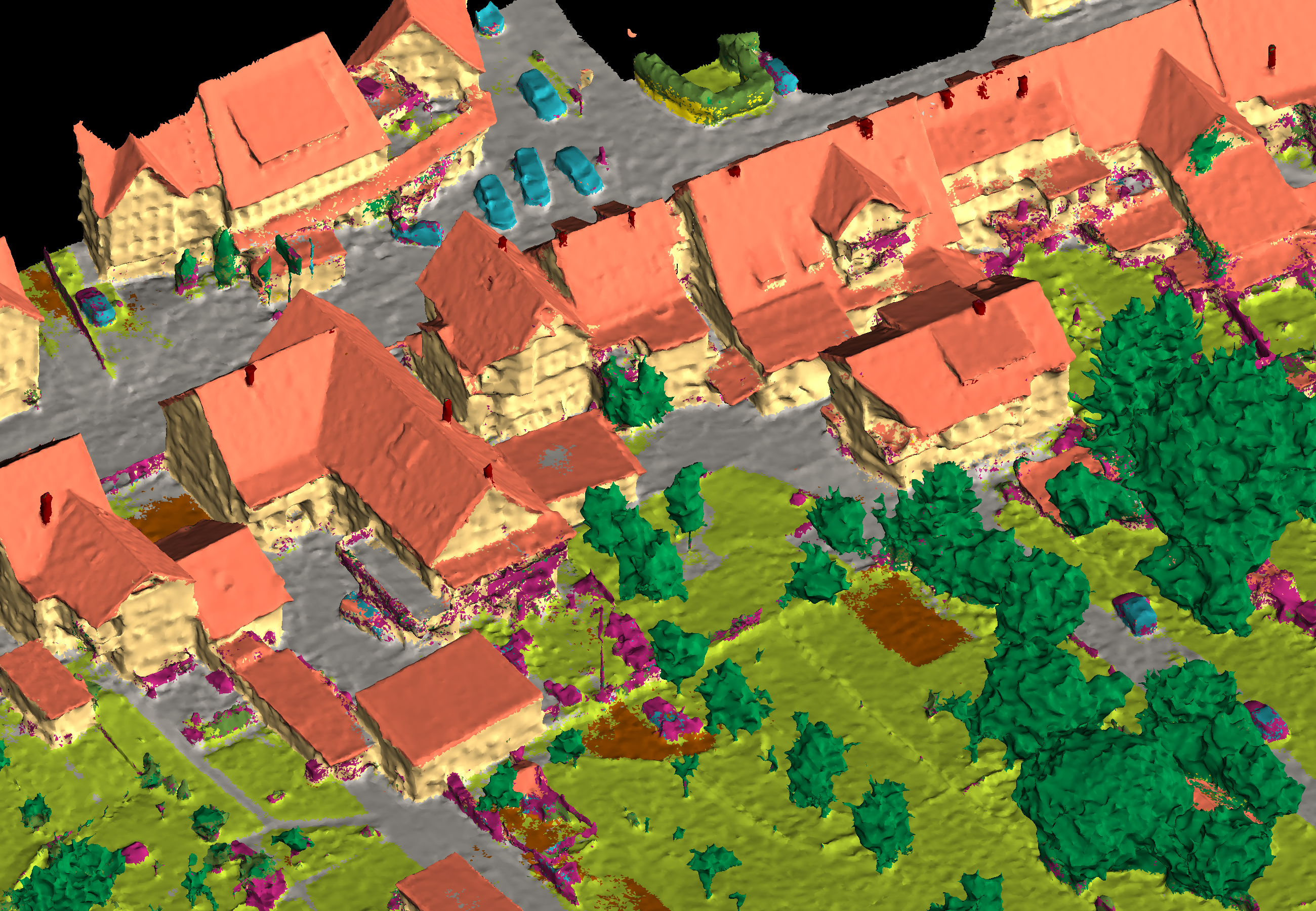
Details on the Approach of ifp-RF-210223
Random Forest baseline approach as described in the official benchmark paper.
More information you will find here
Details on the Approach of ifp-SCN-210223
Sparse Convolutional Network baseline approach as described in the official benchmark paper.
More information you will find here
Details on the Approach of Tang-210406
We develop a deep learning method that combines texture convolution and point convolution for the mesh segmentation task. The method firstly performs texture convolution on faces to extract texture features. Then texture features are concatenated with the normal features of the faces. Finally, we use a U-Net network similar to Pointnet++ to perform point convolution on point cloud, which derived from COGs of the faces, to label the mesh.
Details on the Approach of GAO-KPConv-220330
KPConv: Flexible and Deformable Convolution for Point Clouds
We sample the mesh into colored point clouds with a density of about 10 pts/m2 as input. In particular, we use Monte Carlo sampling (Cignoni et al., 1998) to generate randomly uniform dense samples, and we further prune these samples according to Poisson distributions (Corsini et al., 2012) and assign the colour via searching the nearest neighbour from the textures. We then use the Pytorch version of Kernel Point Convolution (KPConv) with class weights. We set the '0.1' as the first subsampling rate and 5.0 as the radius of the sphere. To avoid precision loss, we translate the coordinates to a local coordinate system. For other configurations, we follow the parameters as used in the dataset 'SemanticKitti'.
link to the code: https://github.com/HuguesTHOMAS/KPConv
More information you will find here
Details on the Approach of GAO-SPG-220330
SPG: Large-scale Point Cloud Semantic Segmentation with Superpoint Graphs
We sample the mesh into colored point clouds (see Fig. 13) with a density of about 10 pts/m2 as input
In particular, we use Monte Carlo sampling (Cignoni et al., 1998) to generate randomly uniform dense
samples, and we further prune these samples according to Poisson distributions (Corsini et al., 2012) and assign the color via searching the nearest neighbor from the textures. We then apply the Superpoint Graph for semantic segmentation, and the original implementation can be found in https://github.com/loicland/superpoint_graph .
More information you will find here
Details on the Approach of GAO-PSSNet-220330
We introduce a novel deep learning-based framework to interpret 3D urban scenes represented as textured meshes. Based on the observation that object boundaries typically align with the boundaries of planar regions, our framework achieves semantic segmentation in two steps: planarity-sensible over-segmentation followed by semantic classification. The over-segmentation step generates an initial set of mesh segments that capture the planar and non-planar regions of urban scenes. In the subsequent classification step, we construct a graph that encodes geometric and photometric features of the segments in its nodes and multi-scale contextual features in its edges. The final semantic segmentation is obtained by classifying the segments using a graph convolutional network.
More information you will find here
Details on the Approach of GAO-PN++-220330
PointNet++
We sample the mesh into colored point clouds with a density of about 10 pts/m2 as input In particular, we use Monte Carlo sampling to generate randomly uniform dense samples, and we further prune these samples according to Poisson distributions and assign the color via searching the nearest neighbor from the textures. We then apply the PointNet++ for semantic segmentation, and the original implementation can be found at https://github.com/torch-points3d/torch-points3d.
More information you will find here
Details on the Approach of Schreiber230926
We present the neural network Mesh Exploring Tensor Net (METNet) for the segmentation of 3D urban scenes, that operates directly on textured meshes. METNet generates its input directly from the textured triangular mesh. It applies a new star-shaped exploration strategy, starting from a triangle on the mesh and expanding in various directions. This way, a vertex based regular local tensor from the unstructured mesh is generated, which we call a Mesh Exploring Tensor (MET). By expanding on the mesh, a MET maintains the geodesic information of the mesh. It also effectively captures the characteristics of large regions. Our new architecture METNet is optimized for processing MET's as input.